Smart Controllers Enabled Dynamic Energy Routing in DC-Grid PV Systems with Uninterrupted EV Charging
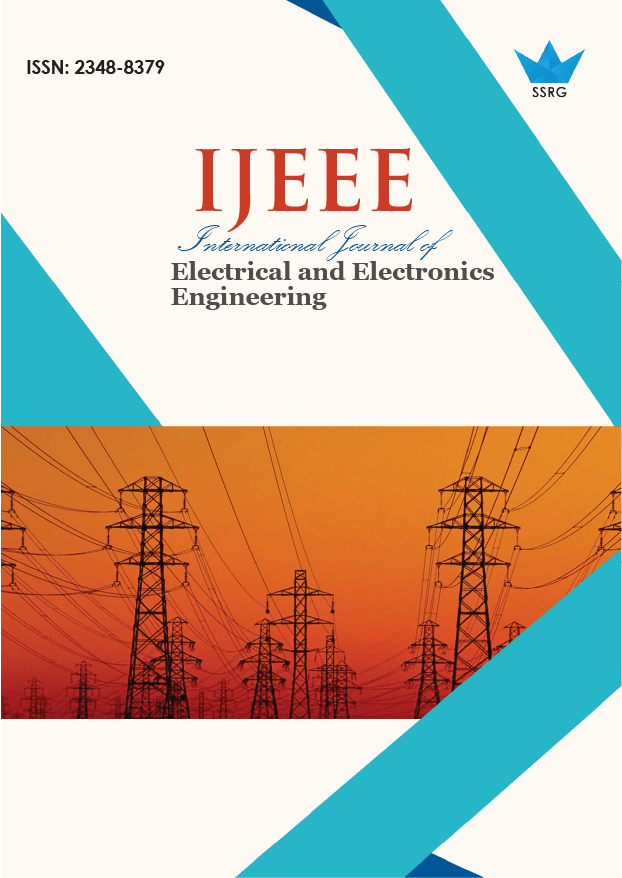
International Journal of Electrical and Electronics Engineering |
© 2024 by SSRG - IJEEE Journal |
Volume 11 Issue 3 |
Year of Publication : 2024 |
Authors : S.V. Kirubakaran, S. Singaravelu |
How to Cite?
S.V. Kirubakaran, S. Singaravelu, "Smart Controllers Enabled Dynamic Energy Routing in DC-Grid PV Systems with Uninterrupted EV Charging," SSRG International Journal of Electrical and Electronics Engineering, vol. 11, no. 3, pp. 165-177, 2024. Crossref, https://doi.org/10.14445/23488379/IJEEE-V11I3P113
Abstract:
Revealing a shift in energy management, this research introduces intelligent energy routing in a dynamic DC-grid integrated Photovoltaic (PV) system named Dynamic Energy Routing (DER). The system features a robust PV array, an enhanced DC-DC boost converter, and a Machine Learning-Support Vector Regression (ML-SVR) MPPT controller connected to a common DC grid. Complementing this setup is a standby battery with a 40AH capacity synchronized with an AC grid through a universal bridge rectifier controlled by an Artificial Neural Network (ANN). Integrating an Electric Vehicle (EV) charging station into the DC grid enhances the system’s versatility. This research explores the dynamic behaviour of a DC-grid integrated PV system under varying State of Charge (SOC) conditions of the standby battery. In scenarios where the standby battery’s SOC is high (≥70%), the system intelligently directs power from the PV system and standby battery to both the EV battery and the AC grid. This strategic routing is activated in response to adverse PV irradiance conditions by ensuring efficient energy utilization. In situations with moderate SOC levels (≤50%), the PV system and standby battery collaborate to supply power to the grid and EV batteries. However, the AC grid intervenes early to adapt to the moderate SOC and reduced irradiance conditions. In low standby battery SOC (<10%), the PV system takes charge by providing charging power to both the standby and EV batteries. In this scenario, the AC grid is promptly activated to contribute the necessary charging power by showing the system’s adaptive response to diverse SOC levels and ensuring reliable energy distribution. Notably, the AC grid activation has done at worst irradiances and lower SOC of standby battery power. This research provides valuable insights into the system’s adaptive and efficient energy routing strategies that contribute to understanding smart control mechanisms in DC-grid integrated PV systems with standby batteries and electric vehicle charging stations.
Keywords:
ANN, ANFIS, ML-SVR, PV, Efficiency, SOC, EV, MPPT, DC grid.
References:
[1] IEA Website, Renewables 2021, IEA, Paris. [Online]. Available: https://www.iea.org/reports/renewables-2021
[2] Santosh Ghosh, and Ranjana Yadav, “Future of Photovoltaic Technologies: A Comprehensive Review,” Sustainable Energy Technologies and Assessments, vol. 47, 2021.
[CrossRef] [Google Scholar] [Publisher Link]
[3] Alexandra Catalina Lazaroiu et al., “A Comprehensive Overview of Photovoltaic Technologies and Their Efficiency for Climate Neutrality,” Sustainability, vol. 15, no. 23, pp. 1-24, 2023.
[CrossRef] [Google Scholar] [Publisher Link]
[4] Sunghyun Moon et al., “Highly Efficient Single-Junction GaAs Thin-Film Solar Cell on Flexible Substrate,” Scientific Reports, vol. 6, pp. 1-6, 2016.
[CrossRef] [Google Scholar] [Publisher Link]
[5] Martin A. Green et al., “Solar Cell Efficiency Tables (Version 60),” Progress in Photovoltaics: Research and Applications, vol. 30, no. 7, pp. 685-805, 2022.
[CrossRef] [Google Scholar] [Publisher Link]
[6] Thai Thanh Nguyen, Hyeong-Jun Yoo, and Hak-Man Kim, “A Flywheel Energy Storage System Based on a Doubly Fed Induction Machine and Battery for Microgrid Control,” Energies, vol. 8, no. 6, pp. 5074-5089, 2015.
[CrossRef] [Google Scholar] [Publisher Link]
[7] Rohit Nishant, Mike Kennedy, and Jacqueline Corbett, “Artificial Intelligence for Sustainability: Challenges, Opportunities, and A Research Agenda,” International Journal of Information Management, vol. 53, 2020.
[CrossRef] [Google Scholar] [Publisher Link]
[8] Salman Salman, Xin AI, and Zhouyang WU, “Design of A P-&-O Algorithm Based MPPT Charge Controller for A Stand-Alone 200W PV System,” Protection and Control Modern Power Systems, vol. 3, pp. 1-8, 2018.
[CrossRef] [Google Scholar] [Publisher Link]
[9] Dezso Sera et al., “Improved MPPT Algorithms for Rapidly Changing Environmental Conditions,” 12th International Power Electronics and Motion Control Conference, Portoroz, Slovenia, pp. 1614-1619, 2006.
[CrossRef] [Google Scholar] [Publisher Link]
[10] A. Frezzetti, S. Manfredi, and A. Suardi, “Adaptive FOCV-Based Control Scheme to Improve the MPP Tracking Performance: An Experimental Validation,” IFAC Proceedings Volumes, vol. 47, no. 3, pp. 4967-4971, 2014.
[CrossRef] [Google Scholar] [Publisher Link]
[11] Hegazy Rezk, and Ali M. Eltamaly, “A Comprehensive Comparison of Different MPPT Techniques for Photovoltaic Systems,” Solar Energy, vol. 112, pp. 1-11, 2015.
[CrossRef] [Google Scholar] [Publisher Link]
[12] Carlos Robles Algarín, John Taborda Giraldo, and Omar Rodríguez Álvarez, “Fuzzy Logic Based MPPT Controller for A PV System,” Energies, vol. 10, no. 12, pp. 1-18, 2017.
[CrossRef] [Google Scholar] [Publisher Link]
[13] Cesar G. Villegas-Mier et al., “Artificial Neural Networks in MPPT Algorithms for Optimization of Photovoltaic Power Systems: A Review,” Micromachines, vol. 12, no. 10, pp. 1-19, 2021.
[CrossRef] [Google Scholar] [Publisher Link]
[14] A. Kumar, M. Rizwan, and U. Nangia, “Development of ANFIS-Based Algorithm for MPPT Controller for Standalone Photovoltaic System,” International Journal of Advanced Intelligence Paradigms, vol. 18, no. 2, pp. 247-264, 2021.
[CrossRef] [Google Scholar] [Publisher Link]
[15] Mpho Sam Nkambule et al., “Comprehensive Evaluation of Machine Learning MPPT Algorithms for a PV System under Different Weather Conditions,” Journal of Electrical Engineering and Technology, vol. 16, pp. 411-427, 2020.
[CrossRef] [Google Scholar] [Publisher Link]
[16] P. Venkata Mahesh, S. Meyyappan, and Rama Koteswara Rao Alla, “Maximum Power Point Tracking Using Decision-Tree Machine-Learning Algorithm for Photovoltaic Systems,” Clean Energy, vol. 6, no. 5, pp. 762-775, 2022.
[CrossRef] [Google Scholar] [Publisher Link]
[17] Bo Zhao et al., “Operation Optimization of Standalone Microgrids Considering Lifetime Characteristics of Battery Energy Storage System,” IEEE Transactions on Sustainable Energy, vol. 4, no. 4, pp. 934-943, 2013.
[CrossRef] [Google Scholar] [Publisher Link]
[18] Muhammad Hammad Saeed et al., “A Review on Microgrids’ Challenges & Perspectives,” IEEE Access, vol. 9, pp. 166502-166517, 2021.
[CrossRef] [Google Scholar] [Publisher Link]
[19] Sigma Ray et al., “Review of Electric Vehicles Integration Impacts in Distribution Networks: Placement, Charging/Discharging Strategies, Objectives and Optimisation Models,” Journal of Energy Storage, vol. 72, Part D, 2023.
[CrossRef] [Google Scholar] [Publisher Link]
[20] K.N. Nwaigwe, P. Mutabilwa, and E. Dintwa, “An Overview of Solar Power (PV Systems) Integration into Electricity Grids,” Materials Science for Energy Technologies, vol. 2, no. 3, pp. 629-633, 2019.
[CrossRef] [Google Scholar] [Publisher Link]
[21] Md. Shohag Hossain, Naruttam K. Roy, and Md. Osman Ali, “Modeling of Solar Photovoltaic System Using MATLAB/Simulink,” 19th International Conference on Computer and Information Technology (ICCIT), Dhaka, Bangladesh, pp. 128-133, 2016.
[CrossRef] [Google Scholar] [Publisher Link]
[22] Taesoo D. Lee, and Abasifreke U. Ebong, “A Review of Thin Film Solar Cell Technologies and Challenges,” Renewable and Sustainable Energy Reviews, vol. 70, pp. 1286-1297, 2017.
[CrossRef] [Google Scholar] [Publisher Link]
[23] Why Use Gallium Arsenide Solar Cells? - Alta Devices, The Atla Devices Website, 2022. [Online]. Available: https://www.altadevices.com/use-gallium-arsenide-solar-cells/
[24] Abdul Ghani Olabi et al., “Rechargeable Batteries: Technological Advancement, Challenges, Current and Emerging Applications,” Energy, vol. 266, 2023.
[CrossRef] [Google Scholar] [Publisher Link]
[25] Ignacio Villanueva et al., “L vs. LCL Filter for Photovoltaic Grid-Connected Inverter: A Reliability Study,” International Journal of Photoenergy, vol. 2020, pp. 1-10, 2020.
[CrossRef] [Google Scholar] [Publisher Link]
[26] Pradeep Kumar S., Vidhya Koothupalakkal Viswambharan, and Swaroop Pillai, “Performance Analysis of Maximum Power Point Tracking of PV Systems Using Artificial Neural Networks and Support Vector Machines,” International Conference on Computational Intelligence and Knowledge Economy (ICCIKE), Dubai, United Arab Emirates, pp. 511-515, 2023.
[CrossRef] [Google Scholar] [Publisher Link]
[27] Chih-Chung Chang, and Chih-Jen Lin, “LIBSVM: A Library for Support Vector Machines,” ACM Transactions on Intelligent Systems and Technology, vol. 2, no. 3, pp. 1-27, 2011.
[CrossRef] [Google Scholar] [Publisher Link]
[28] Dominic Savio Abraham et al., “Fuzzy-Based Efficient Control of DC Microgrid Configuration for PV-Energized EV Charging Station,” Energies, vol. 16, no. 6, pp. 1-17, 2023.
[CrossRef] [Google Scholar] [Publisher Link]
[29] Youssef Alidrissi et al., “An Energy Management Strategy for DC Microgrids with PV/Battery Systems,” Journal of Electrical Engineering & Technology, vol. 16, pp. 1285-1296, 2021.
[CrossRef] [Google Scholar] [Publisher Link]