Using Optimized Artificial Intelligence Techniques to Prevent Cyber Security with the Internet of Things
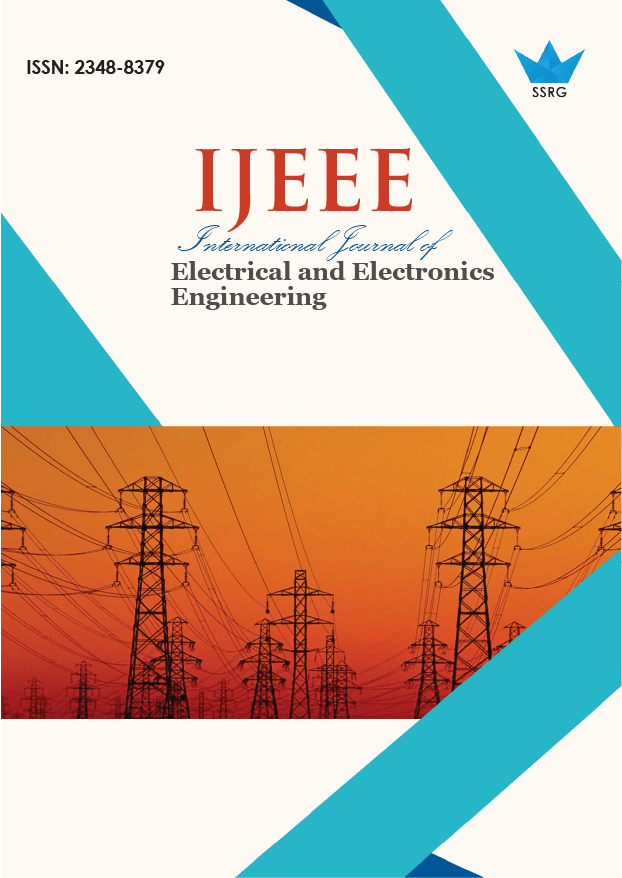
International Journal of Electrical and Electronics Engineering |
© 2024 by SSRG - IJEEE Journal |
Volume 11 Issue 3 |
Year of Publication : 2024 |
Authors : Vidya Sivalingam, Shabana Parveen, Rubeena, Jayasuriya Panchalingam |
How to Cite?
Vidya Sivalingam, Shabana Parveen, Rubeena, Jayasuriya Panchalingam, "Using Optimized Artificial Intelligence Techniques to Prevent Cyber Security with the Internet of Things," SSRG International Journal of Electrical and Electronics Engineering, vol. 11, no. 3, pp. 201-208, 2024. Crossref, https://doi.org/10.14445/23488379/IJEEE-V11I3P116
Abstract:
The Internet of Things (IoT) provides high levels of security for physical items like smart appliances and household appliances. The Internet Protocol (IP) gives each physical item a distinct online address that it may use to communicate with other devices on the network and the outside world via the internet. As the number of hacker attacks on data transmission over the internet continues to climb, there is a growing concern about cybersecurity vulnerabilities in IoT devices. To construct a reliable Cyber Security (CS) system in the face of such potent attacks, attack detection is essential. Common threats to IoT systems include data-type probing, Denial-of-Service (DoS), and User-to-Root (U2R) attacks. Unfortunately, current methods for detecting and investigating IoT malware are insufficient. DoS attacks occur in IoT settings due to inadequate security monitoring and preventive actions. In order to predict attacks as well as problems with IoT devices, this article examines a number of performance-based Artificial Intelligence (AI) algorithms. Several improved optimisation approaches, particularly particle swarm optimisation techniques, were used to determine the productivity of the proposed AI strategy in detail for four different parameters. Hence, this article combines a machine learning method with an optimization algorithm to perform efficient feature extraction. The proposed method’s efficiency is shown by relating its outcomes to those of the existing systems.
Keywords:
Cyber Security, AI, Deep Learning (DL), IoT, Optimization.
References:
[1] Hui Wu et al., “Research on Artificial Intelligence Enhancing Internet of Things Security: A Survey,” IEEE Access, vol. 8, pp. 153826- 153848, 2020.
[CrossRef] [Google Scholar] [Publisher Link]
[2] Murat Kuzlu, Corinne Fair, and Ozgur Guler, “Role of Artificial Intelligence in the Internet of Things (IoT) Cybersecurity,” Discover Internet of Things, vol. 1, pp. 1-14, 2021.
[CrossRef] [Google Scholar] [Publisher Link]
[3] Diptiben Ghelani, “Deep Learning and Artificial Intelligence Framework to Improve the Cyber Security,” Authorea Preprints, pp. 1-11, 2022.
[CrossRef] [Google Scholar] [Publisher Link]
[4] Aimin Yang et al., “Design of Intrusion Detection System for Internet of Things Based on Improved BP Neural Network,” IEEE Access, vol. 7, pp. 106043-106052, 2019.
[CrossRef] [Google Scholar] [Publisher Link]
[5] Bin Cao et al., “A Many-Objective Optimization Model of Industrial Internet of Things Based on Private Blockchain,” IEEE Network, vol. 34, no. 5, pp. 78-83, 2020.
[CrossRef] [Google Scholar] [Publisher Link]
[6] Ricardo Jorge Raimundo, and Albérico Travassos Rosário, “Cybersecurity in the Internet of Things in Industrial Management,” Applied Sciences, vol. 12, no. 3, pp. 1-19, 2022.
[CrossRef] [Google Scholar] [Publisher Link]
[7] Mohamed Amine Ferrag et al., “Federated Deep Learning for Cyber Security in the Internet of Things: Concepts, Applications, and Experimental Analysis,” IEEE Access, vol. 9, pp. 138509-138542, 2021.
[CrossRef] [Google Scholar] [Publisher Link]
[8] Hao Xu et al., “A Data-Driven Approach for Intrusion and Anomaly Detection Using Automated Machine Learning for the Internet of Things,” Soft Computing, vol. 27, pp. 14469-14481, 2023.
[CrossRef] [Google Scholar] [Publisher Link]
[9] Claudio Tomazzoli, Simone Scannapieco, and Matteo Cristani, “Internet of Things and Artificial Intelligence Enable Energy Efficiency,” Journal of Ambient Intelligence and Humanized Computing, vol. 14, no. 5, pp. 4933-4954, 2023.
[CrossRef] [Google Scholar] [Publisher Link]
[10] Joey Li et al., “Methods and Applications for Artificial Intelligence, Big Data, Internet of Things, and Blockchain in Smart Energy Management,” Energy and AI, vol. 11, pp. 1-18, 2023.
[CrossRef] [Google Scholar] [Publisher Link]
[11] Bomin Mao, Yuichi Kawamoto, and Nei Kato, “AI-Based Joint Optimization of QoS and Security for 6G Energy Harvesting Internet of Things,” IEEE Internet of Things Journal, vol. 7, no. 8, pp. 7032-7042, 2020.
[CrossRef] [Google Scholar] [Publisher Link]
[12] Bimal Ghimire, and Danda B. Rawat, “Recent Advances on Federated Learning for Cybersecurity and Cybersecurity for Federated Learning for Internet of Things,” IEEE Internet of Things Journal, vol. 9, no. 11, pp. 8229-8249, 2022.
[CrossRef] [Google Scholar] [Publisher Link]
[13] Iqbal H. Sarker et al., “Internet of Things (IoT) Security Intelligence: A Comprehensive Overview, Machine Learning Solutions and Research Directions,” Mobile Networks and Applications, vol. 28, pp. 296-312, 2023.
[CrossRef] [Google Scholar] [Publisher Link]
[14] Romany Fouad Mansour et al., “Artificial Intelligence and Internet of Things Enabled Disease Diagnosis Model for Smart Healthcare Systems,” IEEE Access, vol. 9, pp. 45137-45146, 2021.
[CrossRef] [Google Scholar] [Publisher Link]
[15] Emil Blixt Hansen, and Simon Bøgh, “Artificial Intelligence and Internet of Things in Small and Medium-Sized Enterprises: A Survey,” Journal of Manufacturing Systems, vol. 58, pp. 362-372, 2021.
[CrossRef] [Google Scholar] [Publisher Link]
[16] Fan Liang et al., “Machine Learning for Security and the Internet of Things: The Good, The Bad, and The Ugly,” IEEE Access, vol. 7, pp. 158126-158147, 2019.
[CrossRef] [Google Scholar] [Publisher Link]
[17] Jesús Galeano-Brajones et al., “Detection and Mitigation of DoS and DDoS Attacks in IoT-Based Stateful SDN: An Experimental Approach,” Sensors, vol. 20, no. 3, pp. 1-18, 2020.
[CrossRef] [Google Scholar] [Publisher Link]
[18] By Wang Qi, “Analysis on the Application of Artificial Intelligence in Classroom,” Journal of Physics: Conference Series, vol. 1345, no. 4, pp. 402-420, 2019.
[CrossRef] [Google Scholar] [Publisher Link]
[19] Hongrui Bao et al., “Research on Information Security Situation Awareness System Based on Big Data and Artificial Intelligence Technology,” 2019 International Conference on Robots & Intelligent System (ICRIS), Haikou, China, pp. 318-322, 2019.
[CrossRef] [Google Scholar] [Publisher Link]
[20] S. Shitharth et al., “An Innovative Perceptual Pigeon Galvanized Optimization (PPGO) Based Likelihood Naïve Bayes (LNB) Classification Approach for Network Intrusion Detection System,” IEEE Access, vol. 10, pp. 46424-46441, 2022.
[CrossRef] [Google Scholar] [Publisher Link]
[21] Pratibha Singh, Ajay Verma, and Narendra S. Chaudhari, “Feature Selection-Based Classifier Combination Approach for Handwritten Devanagari Numeral Recognition,” Sadhana, vol. 40, no. 6, pp. 1701-1714, 2015.
[CrossRef] [Google Scholar] [Publisher Link]
[22] Christopher D. McDermott, John P. Isaacs, and Andrei V. Petrovski, “Evaluating Awareness and Perception of Botnet Activity within Consumer Internet-of Things (IoT) Networks,” Informatics, vol. 6, no. 8, pp. 1-15, 2019.
[CrossRef] [Google Scholar] [Publisher Link]
[23] Hassan A. Alterazi et al., “Prevention of Cyber Security with the Internet of Things Using Particle Swarm Optimization,” Sensors, vol. 22, no. 16, pp. 1-17, 2022.
[CrossRef] [Google Scholar] [Publisher Link]
[24] Nilesh Kunhare, Ritu Tiwari, and Joydip Dhar, “Particle Swarm Optimization and Feature Selection for Intrusion Detection System,” Sādhanā, vol. 45, pp. 1-14, 2020.
[CrossRef] [Google Scholar] [Publisher Link]
[25] Sami Ben Jabeur et al., “CatBoost Model and Artificial Intelligence Techniques for Corporate Failure Prediction,” Technological Forecasting and Social Change, vol. 166, 2021.
[CrossRef] [Google Scholar] [Publisher Link]