Harnessing Blockchain for Collective Defense: A Strategy for Detecting and Combating DDoS Attacks
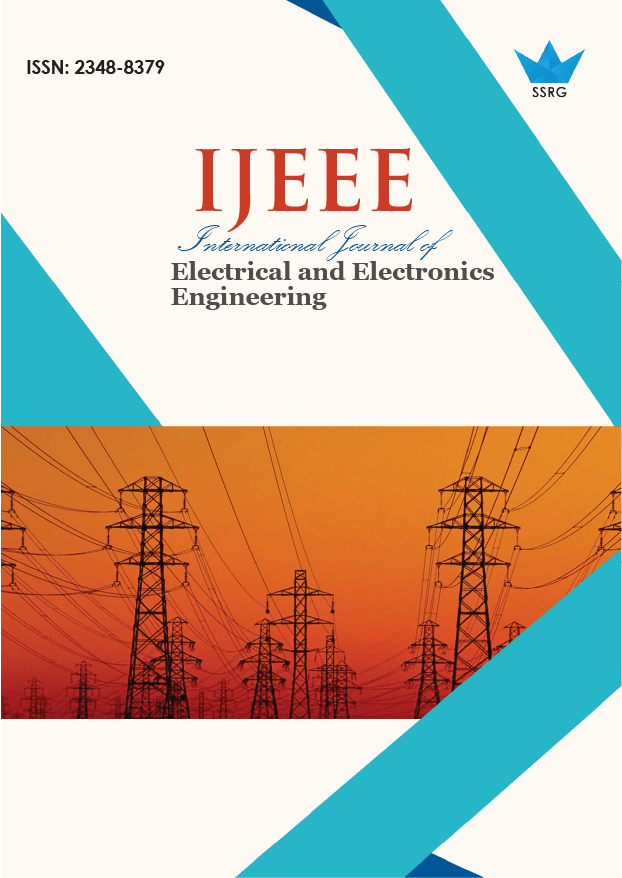
International Journal of Electrical and Electronics Engineering |
© 2024 by SSRG - IJEEE Journal |
Volume 11 Issue 3 |
Year of Publication : 2024 |
Authors : Kriti Patidar, Swapnil Jain |
How to Cite?
Kriti Patidar, Swapnil Jain, "Harnessing Blockchain for Collective Defense: A Strategy for Detecting and Combating DDoS Attacks," SSRG International Journal of Electrical and Electronics Engineering, vol. 11, no. 3, pp. 299-307, 2024. Crossref, https://doi.org/10.14445/23488379/IJEEE-V11I3P125
Abstract:
Among the current security challenges faced by the Internet, Distributed Denial of Service (DDoS) attacks loom as a significant threat. DDoS attacks represent potent cyber threats designed to incapacitate services by overwhelming servers, thereby hindering their responsiveness to users. These attacks can swiftly deplete the processing and communication capabilities of the targeted entity. The previous few years have seen a noticeable surge in the frequency and duration of DDoS attacks, rendering them more impactful and perilous. The surge in insecure mobile device usage and escalating traffic volumes contribute to the heightened risk posed by DDoS attacks on various services. This paper proposes a collaborative approach for identifying and mitigating DDoS flooding attacks. The utilization of smart contracts can play a crucial role in identifying malicious actors, subsequently enabling their inclusion in blocklists. Leveraging blockchain technology simplifies the complexity of the DDoS signaling system, offering an effective means for numerous independent and distributed systems to collaborate. Through resource and defense characteristic sharing, blockchain facilitates a robust defense strategy against DDoS attacks.
Keywords:
Blockchain, Denial of Service, DDoS attacks, DDoS mitigation, IP spoofing, TCP SYN flooding.
References:
[1] Neha Agrawal, and Shashikala Tapaswi, “Defense Mechanisms against DDoS Attacks in a Cloud Computing Environment: State-of-theArt and Research Challenges,” IEEE Communications Surveys & Tutorials, vol. 21, no. 4, pp. 3769-3795, 2019.
[CrossRef] [Google Scholar] [Publisher Link]
[2] Karanbir Singh, Kanwalvir Singh Dhindsa, and Deepa Nehra, “T-CAD: A threshold Based Collaborative DDoS Attack Detection in Multiple Autonomous Systems,” Journal of Information Security and Applications, vol. 51, 2020.
[CrossRef] [Google Scholar] [Publisher Link]
[3] Afsaneh Banitalebi Dehkordi, MohammadReza Soltanaghaei, and Farsad Zamani Boroujeni, “The DDoS Attacks Detection through Machine Learning and Statistical Methods in SDN,” The Journal of Supercomputing, vol. 77, pp. 2383-2415, 2021.
[CrossRef] [Google Scholar] [Publisher Link]
[4] Elahe Fazeldehkordi, Olaf Owe, and Toktam Ramezanifarkhani, “A Language-Based Approach to Prevent DDoS Attacks in Distributed Financial Agent Systems,” International Workshop on Information and Operational Technology Security Systems, Computer Security, pp. 258-277, 2019.
[CrossRef] [Google Scholar] [Publisher Link]
[5] An Wang et al., “A Data-Driven Study of DDoS Attacks and Their Dynamics,” IEEE Transactions on Dependable and Secure Computing, vol. 17, no. 3, pp. 648-661, 2020.
[CrossRef] [Google Scholar] [Publisher Link]
[6] Jieren Cheng et al., “A Novel DDOS Attack Detection Method Using Optimized Generalized Multiple Kernel Learning,” Computers, Materials & Continua, vol. 62, no. 3, pp. 1423-1443, 2020.
[CrossRef] [Google Scholar] [Publisher Link]
[7] Seth Djane Kotey, Eric Tutu Tchao, and James Dzisi Gadze, “On Distributed Denial of Service Current Defense Schemes,” Technologies, vol. 7, no. 1, pp. 1-24, 2019.
[CrossRef] [Google Scholar] [Publisher Link]
[8] Sanghun Choi, Yichen An, and Iwao Sasase, “A Lightweight Detection Using Bloom Filter against Flooding DDoS Attack,” IEICE Transactions on Information and Systems, vol. E103.D, no. 12, pp. 2600-2610, 2020.
[CrossRef] [Google Scholar] [Publisher Link]
[9] Marco Mazzoni, Antonio Corradi, and Vincenzo Di Nicola, “Performance Evaluation of Permissioned Blockchains for Financial Applications: The Consensys Quorum Case Study,” Blockchain: Research and Applications, vol. 3, no. 1, pp. 1-12, 2022.
[CrossRef] [Google Scholar] [Publisher Link]
[10] Tobias Guggenberger et al., “An In-Depth Investigation of the Performance Characteristics of Hyperledger Fabric,” Computers & Industrial Engineering, vol. 173, pp. 1-20, 2022.
[CrossRef] [Google Scholar] [Publisher Link]
[11] Ahmed Afif Monrat, Olov Schelén, and Karl Andersson, “A Survey of Blockchain from the Perspectives of Applications, Challenges, and Opportunities,” IEEE Access, vol. 7, pp. 117134-117151, 2019.
[CrossRef] [Google Scholar] [Publisher Link]
[12] Bruno Rodrigues et al., “Evaluating A Blockchain-Based Cooperative Defense,” 2019 IFIP/IEEE Symposium on Integrated Network and Service Management (IM), Arlington, USA, pp. 533-538, 2019.
[Google Scholar] [Publisher Link]
[13] B.B. Gupta, R.C. Joshi, and Manoj Misra, “Distributed Denial of Service Prevention Techniques,” International Journal of Computer and Electrical Engineering, vol. 2, no. 2, pp. 268-276, 2012.
[CrossRef] [Google Scholar] [Publisher Link]
[14] Tasnuva Mahjabi et al., “A Survey of Distributed Denial-of-Service Attack, Prevention, and Mitigation Techniques,” International Journal of Distributed Sensor Networks, vol. 13, no. 12, 2017.
[CrossRef] [Google Scholar] [Publisher Link]
[15] Neelam Dayal, and Shashank Srivastava, “Analyzing Behavior of DDoS Attacks to Identify DDoS Detection Features in SDN,” 2017 9 th International Conference on Communication Systems and Networks (COMSNETS), Bengaluru, India, pp. 274-281, 2017.
[CrossRef] [Google Scholar] [Publisher Link]
[16] K. Geetha, and N. Sreenath, “SYN Flooding Attack - Identification and Analysis,” International Conference on Information Communication and Embedded Systems (ICICES2014), Chennai, India, pp. 1-7, 2014.
[CrossRef] [Google Scholar] [Publisher Link]
[17] Mitko Bogdanoski, Tomislav Shuminoski, and Aleksandar Risteski, “Analysis of the SYN Flood DoS Attack,” International Journal of Computer Network and Information Security, vol. 5, no. 8, pp. 1-11, 2013.
[CrossRef] [Google Scholar] [Publisher Link]
[18] Xiaojun Guo, and Xuan Gao, “A SYN Flood Attack Detection Method Based on Hierarchical Multihead Self-Attention Mechanism,” Security and Communication Networks, vol. 2022, pp. 1-13, 2022.
[CrossRef] [Google Scholar] [Publisher Link]
[19] Omer Elsier Tayfour, and Muhammad Nadzir Marsono, “Collaborative Detection and Mitigation of Distributed Denial-of-Service Attacks on Software-Defined Network,” Mobile Networks and Applications, vol. 25, pp. 1338-1347, 2020.
[CrossRef] [Google Scholar] [Publisher Link]
[20] Meryam Essaid et al., “A Collaborative DDoS Mitigation Solution Based on Ethereum Smart Contract and RNN-LSTM,” 2019 20th AsiaPacific Network Operations and Management Symposium (APNOMS), Matsue, Japan, pp. 1-6, 2019.
[CrossRef] [Google Scholar] [Publisher Link]
[21] Nguyen Ngoc Tuan et al., “A DDoS Attack Mitigation Scheme in ISP Networks Using Machine Learning Based on SDN,” Electronics, vol. 9, no. 3, pp. 1-19, 2020.
[CrossRef] [Google Scholar] [Publisher Link]
[22] Georgios Spathoulas et al., “Collaborative Blockchain-Based Detection of Distributed Denial of Service Attacks Based on Internet of Things Botnets,” Future Internet, vol. 11, no. 11, pp. 1-24, 2019.
[CrossRef] [Google Scholar] [Publisher Link]
[23] Weizhi Meng et al., “When Intrusion Detection Meets Blockchain Technology: A Review,” IEEE Access, vol. 6, pp. 10179-10188, 2018.
[CrossRef] [Google Scholar] [Publisher Link]
[24] Ui-Jun Baek et al., “DDoS Attack Detection on Bitcoin Ecosystem Using Deep-Learning,” 2019 20th Asia-Pacific Network Operations and Management Symposium (APNOMS), Matsue, Japan, pp. 1-4, 2019.
[CrossRef] [Google Scholar] [Publisher Link]
[25] Tsuyoshi Idé, “Collaborative Anomaly Detection on Blockchain from Noisy Sensor Data,” 2018 IEEE International Conference on Data Mining Workshops (ICDMW), Singapore, pp. 120-127, 2018.
[CrossRef] [Google Scholar] [Publisher Link]
[26] Bruno Rodrigues et al., “A Blockchain-Based Architecture for Collaborative DDoS Mitigation with Smart Contracts,” Security of Networks and Services in an All-Connected World, pp. 16-29, 2017.
[CrossRef] [Google Scholar] [Publisher Link]
[27] Yiyun Zhou et al., “Improving IoT Services in Smart-Home Using Blockchain Smart Contract,” 2018 IEEE International Conference on Internet of Things (iThings) and IEEE Green Computing and Communications (GreenCom) and IEEE Cyber, Physical and Social Computing (CPSCom) and IEEE Smart Data (SmartData), Halifax, Canada, pp. 81-87, 2018.
[CrossRef] [Google Scholar] [Publisher Link]
[28] Jose Eduardo A. Sousa et al., “Fighting Under-Price DoS Attack in Ethereum with Machine Learning Techniques,” ACM Sigmetrics - Performance Evaluation Review, vol. 48, no. 4, pp. 24-27, 2021.
[CrossRef] [Google Scholar] [Publisher Link]
[29] Muhammad Saad, My T. Thai, and Aziz Mohaisen, “POSTER: Deterring DDoS Attacks on Blockchain-Based Cryptocurrencies through Mempool Optimization,” Proceedings of the 2018 on Asia Conference on Computer and Communications Security, pp. 809-811, 2018.
[CrossRef] [Google Scholar] [Publisher Link]
[30] D.V.V.S. Manikumar, and B. Uma Maheswari, “Blockchain Based DDoS Mitigation Using Machine Learning Techniques,” 2020 Second International Conference on Inventive Research in Computing Applications (ICIRCA), Coimbatore, India, pp. 794-800, 2020.
[CrossRef] [Google Scholar] [Publisher Link]
[31] A.P. Pandian, and S. Smys, “DDOS Attack Detection in Telecommunication Network Using Machine Learning,” Journal of Ubiquitous Computing and Communication Technologies, vol. 1, no. 1, pp. 33-44, 2019.
[CrossRef] [Google Scholar] [Publisher Link]
[32] Yuan-Hsiang Su, Amir Rezapour, and Wen-Guey Tzeng, “The Forward-Backward String: A New Robust Feature for Botnet Detection,” 2017 IEEE Conference on Dependable and Secure Computing, Taipei, Taiwan, pp. 485-492, 2017.
[CrossRef] [Google Scholar] [Publisher Link]
[33] Xiaoyong Yuan, Chuanhuang Li, and Xiaolin Li, “DeepDefense: Identifying DDoS Attack via Deep Learning,” 2017 IEEE International Conference on Smart Computing (SMARTCOMP), Hong Kong, China, pp. 1-8, 2017.
[CrossRef] [Google Scholar] [Publisher Link]
[34] Yichen An et al., “Traffic Feature-Based Botnet Detection Scheme Emphasizing the Importance of Long Patterns,” International Conference on Image Processing and Communications, pp. 181-188, 2019.
[CrossRef] [Google Scholar] [Publisher Link]