Modified Leach Ant Colony Optimization Hybrid Algorithm for Energy-Harvesting Wireless Sensor Network
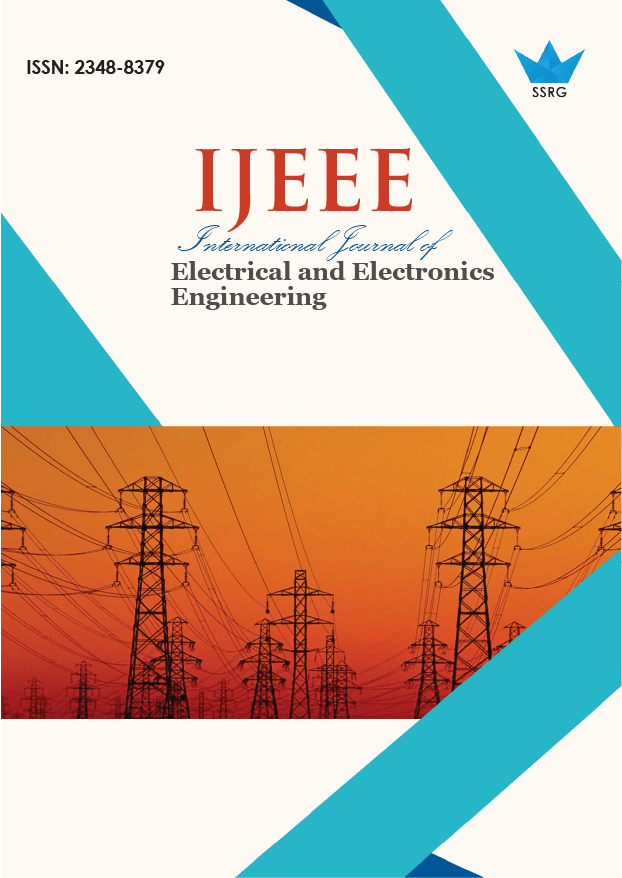
International Journal of Electrical and Electronics Engineering |
© 2024 by SSRG - IJEEE Journal |
Volume 11 Issue 3 |
Year of Publication : 2024 |
Authors : Angeeta Hirwe Chouhan, Nitin Kumar Jain, Ravi Sindal |
How to Cite?
Angeeta Hirwe Chouhan, Nitin Kumar Jain, Ravi Sindal, "Modified Leach Ant Colony Optimization Hybrid Algorithm for Energy-Harvesting Wireless Sensor Network," SSRG International Journal of Electrical and Electronics Engineering, vol. 11, no. 3, pp. 324-331, 2024. Crossref, https://doi.org/10.14445/23488379/IJEEE-V11I3P127
Abstract:
Wireless Sensor Networks (WSNs) comprise a multitude of sensor nodes distributed in a random fashion across the designated area. These nodes are responsible for monitoring and recording physical data from their surroundings. Subsequently, they transmit this collected information to a central base station using wireless communication. Two critical applications of WSNs include biomedical and environmental monitoring. However, in challenging environments, replacing the sensor node’s battery is nearly impractical once the sensor node’s battery is fully depleted. Consequently, maintaining the network’s lifespan and ensuring reliable communication between nodes in such harsh conditions pose significant challenges for researchers. This research aims to enhance both the survivability of the network and its Network Throughput (NTh). To achieve this, a simplified energy harvesting model is employed to enhance the network’s ability to survive in challenging conditions. This paper introduces a novel hybrid scheme for selecting Cluster Heads (CH) in energy harvesting WSNs, which is termed Modified Leach Ant Colony Optimization (MLACO). This scheme not only enhances the Network Throughput but also improves the network’s overall survivability. Comparative analysis against existing clustering methods like MLEACH and FEEDC reveals that the proposed MLACO scheme outperforms both of them. On average, MLACO exhibits a 31.5% higher network throughput compared to MLEACH and a 26.6% improvement over the FEEDC CH selection strategy. Moreover, the network survivability demonstrated by the proposed scheme is consistent with that of established methods and surpasses them, particularly between 900 and 1300 rounds.
Keywords:
Solar Harvested Energy Wireless Sensor Network (SHE-WSN), Network’s lifespan, Network survivability, Cluster Heads (CHs), Network throughput.
References:
[1] Hoi Ching Tung et al., “A Mobility Enabled Inpatient Monitoring System Using A ZigBee Medical Sensor Network,” Sensors, vol. 14, no. 2, pp. 2397-2416, 2014.
[CrossRef] [Google Scholar] [Publisher Link]
[2] J.J. McCullagh et al., “Long-Term Testing of a Vibration Harvesting System for the Structural Health Monitoring of Bridges,” Sensors and Actuators A: Physical, vol. 217, pp. 139-150, 2014.
[CrossRef] [Google Scholar] [Publisher Link]
[3] Stefanos A. Nikolidakis et al., “Energy Efficient Automated Control of Irrigation in Agriculture by Using Wireless Sensor Networks,” Computers and Electronics in Agriculture, vol. 113, pp. 154-163, 2015.
[CrossRef] [Google Scholar] [Publisher Link]
[4] Michele Magno et al., “Extended Wireless Monitoring through Intelligent Hybrid Energy Supply,” IEEE Transactions on Industrial Electronics, vol. 61, no. 4, pp. 1871-1881, 2014.
[CrossRef] [Google Scholar] [Publisher Link]
[5] Gwo-Jiun Horng, and Hsin-Te Wu, “The Adaptive Path Selection Mechanism for Solar-Powered Wireless Sensor Networks,” Wireless Personal Communications, vol. 81, pp. 1289-1301, 2015.
[CrossRef] [Google Scholar] [Publisher Link]
[6] Feng Yu, Wei Liu, and Gang Li, “An Improved Cluster Head Algorithm for Wireless Sensor Network,” Computer Engineering and Networking, pp. 65-71, 2013.
[CrossRef] [Google Scholar] [Publisher Link]
[7] Norah Tuah, Mahamod Ismail, and Kasmiran Jumari, “Cluster-Head Selection by Remaining Energy Consideration in a Wireless Sensor Network,” International Conference on Informatics Engineering and Information Science, pp. 498-507, 2011.
[CrossRef] [Google Scholar] [Publisher Link]
[8] W.R. Heinzelman, A. Chandrakasan, and H. Balakrishnan, “Energy-Efficient Communication Protocol for Wireless Microsensor Networks,” Proceedings of the 33rd Annual Hawaii International Conference on System Sciences, Maui, USA, vol. 2, 2000.
[CrossRef] [Google Scholar] [Publisher Link]
[9] B. Baranidharan, and B. Santhi, “DUCF: Distributed Load Balancing Unequal Clustering in Wireless Sensor Networks Using Fuzzy Approach,” Applied Soft Computing, vol. 40, pp. 495-506, 2016.
[CrossRef] [Google Scholar] [Publisher Link]
[10] Aysegul Alaybeyoglu, “A Distributed Fuzzy Logic-Based Root Selection Algorithm for Wireless Sensor Networks,” Computers and Electrical Engineering, vol. 41, pp. 216-225, 2015.
[CrossRef] [Google Scholar] [Publisher Link]
[11] Khalid Haseeb et al., “WECRR: Weighted Energy-Efficient Clustering with Robust Routing for Wireless Sensor Networks,” Wireless Personal Communications, vol. 97, pp. 695-721, 2017.
[CrossRef] [Google Scholar] [Publisher Link]
[12] Adnan Ismail Al-Sulaifanie, Bayez Khorsheed Al-Sulaifanie, and Subir Biswas, “Recent Trends in Clustering Algorithms for Wireless Sensor Networks: A Comprehensive Review,” Computer Communications, vol. 91, pp. 395-424, 2022.
[CrossRef] [Google Scholar] [Publisher Link]
[13] Masood Ahmad et al., “Optimal Clustering in Wireless Sensor Networks for the Internet of Things Based on Memetic Algorithm: memeWSN,” Wireless Communications and Mobile Computing, vol. 2021, pp. 1-14, 2021.
[CrossRef] [Google Scholar] [Publisher Link]
[14] Indra Kumar Shah et al., “ICIC: A Dual Mode Intra-Cluster and Inter-Cluster Energy Minimization Approach for Multihop WSN,” IEEE Access, vol. 10, pp. 70581-70594, 2022.
[CrossRef] [Google Scholar] [Publisher Link]
[15] Indra Kumar Shah, Tanmoy Maity, and Yogendra Singh Dohare, “Algorithm for Energy Consumption Minimisation in Wireless Sensor Network,” IET Communications, vol. 14, no. 8, pp. 1301-1310, 2020.
[CrossRef] [Google Scholar] [Publisher Link]
[16] Indra Kumar Shah, Tanmoy Maity, and Yogendra Singh Dohare, “Weight Based Approach for Optimal Position of Base Station in Wireless Sensor Network,” 2020 International Conference on Inventive Computation Technologies (ICICT), Coimbatore, India, pp. 734- 738 2020.
[CrossRef] [Google Scholar] [Publisher Link]
[17] Liang Zhao, Shaocheng Qu, and Yufan Yi, “A Modified Cluster-Head Selection Algorithm in Wireless Sensor Networks Based on LEACH,” EURASIP Journal on Wireless Communications and Networking, vol. 2018, pp. 1-8, 2018.
[CrossRef] [Google Scholar] [Publisher Link]
[18] Ying Zhang et al., “Fuzzy-Logic Based Distributed Energy-Efficient Clustering Algorithm for Wireless Sensor Networks,” Sensors, vol. 17, no. 7, pp. 1-21, 2017.
[CrossRef] [Google Scholar] [Publisher Link]
[19] Deepak Sharma, and Amol P. Bhondekar, “An Improved Cluster Head Selection in Routing for Solar Energy-Harvesting MultiHeterogeneous Wireless Sensor Networks,” Wireless Personal Communications, vol. 108, pp. 2213-2228, 2019.
[CrossRef] [Google Scholar] [Publisher Link]
[20] Aman Kansal et al., “Power Management in Energy Harvesting Sensor Networks,” ACM Transactions on Embedded Computing Systems (TECS), vol. 6, no. 4, 2007.
[CrossRef] [Google Scholar] [Publisher Link]
[21] Carlo Bergonzini, Davide Brunelli, and Luca Benini, “Algorithms for Harvested Energy Prediction in Batteryless Wireless Sensor Networks,” 2009 3rd International Workshop on Advances in Sensors and Interfaces, Trani, Italy, pp. 144-149, 2009.
[CrossRef] [Google Scholar] [Publisher Link]