How do Media Releases Affect Netflix's Stock
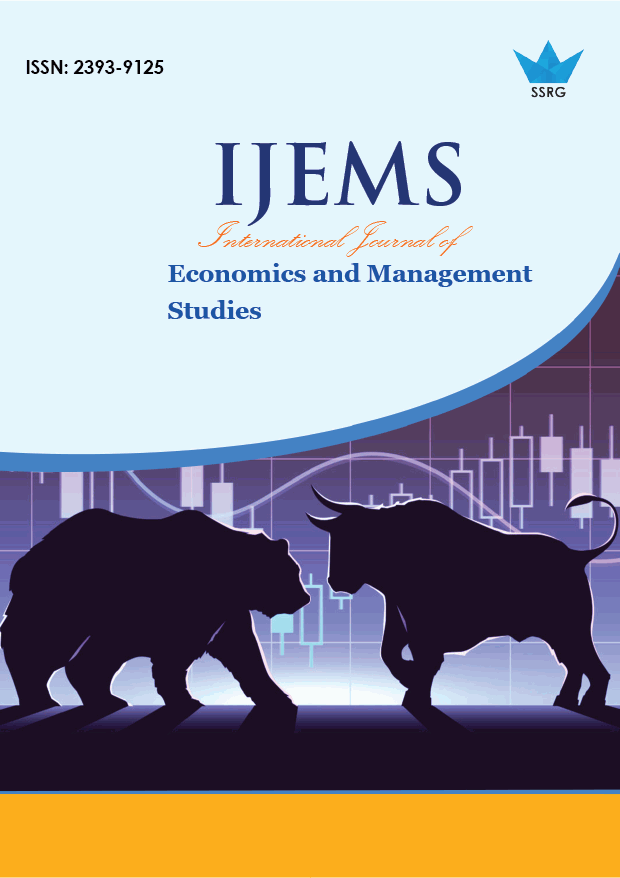
International Journal of Economics and Management Studies |
© 2024 by SSRG - IJEMS Journal |
Volume 11 Issue 12 |
Year of Publication : 2024 |
Authors : Raajvir Vijay |
How to Cite?
Raajvir Vijay, "How do Media Releases Affect Netflix's Stock," SSRG International Journal of Economics and Management Studies, vol. 11, no. 12, pp. 55-68, 2024. Crossref, https://doi.org/10.14445/23939125/IJEMS-V11I12P106
Abstract:
This study investigates the relationship between media releases and Netflix's stock performance, extending the analysis to competitors in the streaming industry. Using data from 2012 to 2024, we examine the popularity of Netflix's content releases, measured through Google search trends, ratings, and social media activity, and correlates with its stock price movements. Our methodology employs a linear regression model, incorporating variables such as show release dates, Google search volumes, and S&P 500 returns. The results reveal a weak positive correlation between Netflix's show releases and its stock returns, but competitor show releases showed negligible correlation. The S&P 500 returns demonstrated the strongest relationship with Netflix's stock movements, underscoring the importance of broader market trends. As measured by Google search volume, public interest showed a minimal negative relationship with stock returns. These findings suggest that while content releases and public interest play a role in Netflix's stock performance, macroeconomic factors and overall market conditions are more influential. This research contributes to the understanding of media influence on financial markets and offers insights for investors and streaming platforms in evaluating the impact of content strategies on stock performance.
Keywords:
Google trends, Media releases, Netflix, Stock returns, Streaming industry.
References:
[1] Number of Netflix Paid Subscribers Worldwide from 1st Quarter 2013 to 3rd Quarter 2024(In Millions), Statista, 2023. [Online]. Available: www.statista.com/statistics/250934/quarterly-number-of-netflix-streaming-subscribers-worldwide.
[2] Johan Bollen, Huina Mao, and Xiaojun Zeng, “Twitter Mood Predicts the Stock Market,” Journal of Computational Science, vol. 2, no. 1, pp. 1-8, 2011.
[CrossRef] [Google Scholar] [Publisher Link]
[3] David Opeoluwa Oyewola, and Emmanuel Gbenga Dada, “Machine Learning Methods for Predicting the Popularity of Movies,” Journal of Artificial Intelligence and Systems, vol. 4, no. 1, pp. 65-82, 2022.
[CrossRef] [Google Scholar] [Publisher Link]
[4] Paul C. Tetlock, “Giving Content to Investor Sentiment: The Role of Media in the Stock Market,” The Journal of Finance, vol. 62, no. 3, pp. 1139-1168, 2007.
[CrossRef] [Google Scholar] [Publisher Link]
[5] Werner Antweiler, and Murray Z. Frank, “Is All That Talk Just Noise? The Information Content of Internet Stock Message Boards,” The Journal of Finance, vol. 59, no. 3, pp. 1259-1294, 2004.
[CrossRef] [Google Scholar] [Publisher Link]
[6] Bin Weng, et al., “Predicting Short-Term Stock Prices Using Ensemble Methods and Online Data Sources,” Expert Systems with Applications, vol. 112, pp. 258-273, 2018.
[CrossRef] [Google Scholar] [Publisher Link]
[7] Sean McNally, Jason Roche, and Simon Caton, “Predicting the Price of Bitcoin Using Machine Learning,” 26th Euromicro International Conference on Parallel, Distributed and Network-Based Processing (PDP), Cambridge, UK, pp. 339-343, 2018.
[CrossRef] [Google Scholar] [Publisher Link]
[8] Michael Siering, “Investigating the Impact of Media Sentiment and Investor Attention on Financial Markets,” Enterprise Applications and Services in the Finance Industry, Barcelona, Spain, pp. 3-19, 2013.
[CrossRef] [Google Scholar] [Publisher Link]
[9] Brad M. Barber, and Terrance Odean, “All That Glitters: The Effect of Attention and News on the Buying Behavior of Individual and Institutional Investors,” The Review of Financial Studies, vol. 21, no. 2, pp. 785-818, 2008.
[CrossRef] [Google Scholar] [Publisher Link]
[10] Jeffrey A. Busse and, T. Clifton Green, “Market Efficiency in Real Time,” Journal of Financial Economics, vol. 65, no. 3, pp. 415-437, 2002.
[CrossRef] [Google Scholar] [Publisher Link]
[11] Zhi Da, Joseph Engelberg, and Pengjie Gao, “In Search of Attention,” The Journal of Finance, vol. 66, no. 5, pp. 1461-1499, 2011.
[CrossRef] [Google Scholar] [Publisher Link]
[12] Thomas Chemmanur, and An Yan, “Product Market Advertising and New Equity Issues,” Journal of Financial Economics, vol. 92, no. 1, pp. 40-65, 2009.
[CrossRef] [Google Scholar] [Publisher Link]
[13] Axel Groß-Klußmann, and Nikolaus Hautsch, “When Machines Read the News: Using Automated Text Analytics to Quantify High Frequency News-Implied Market Reactions,” Journal of Empirical Finance, vol. 18, no. 2, pp. 321-340, 2011.
[CrossRef] [Google Scholar] [Publisher Link]
[14] Steven L. Heston, and Nitish Ranjan Sinha, “News vs. Sentiment: Predicting Stock Returns from News Stories,” Financial Analysts Journal, vol. 73, no. 3, pp. 67-83, 2017.
[CrossRef] [Google Scholar] [Publisher Link]
[15] Eugene F. Fama, and Kenneth R. French, “Common Risk Factors in the Returns on Stocks and Bonds,” Journal of Financial Economics, vol. 33, no. 1, pp. 3-56, 1993.
[CrossRef] [Google Scholar] [Publisher Link]
[16] Joseph E. Engelberg, and Christopher A. Parsons, “The Causal Impact of Media in Financial Markets,” The Journal of Finance, vol. 66, no. 1, pp. 67-97, 2011.
[CrossRef] [Google Scholar] [Publisher Link]
[17] Timm O. Sprenger et al., “Tweets and Trades: The Information Content of Stock Microblogs,” European Financial Management, vol. 20, no. 5, pp. 926-957, 2014.
[CrossRef] [Google Scholar] [Publisher Link]
[18] J. Durbin, and G.S. Watson, “Testing for Serial Correlation in Least Squares Regression: I,” Biometrika, vol. 37, pp. 409-428, 1950.
[CrossRef] [Google Scholar] [Publisher Link]
[19] T.S. Breusch, and A.R. Pagan, “A Simple Test for Heteroscedasticity and Random Coefficient Variation,” Econometrica, vol. 47, no. 5, pp. 1287-1294, 1979.
[CrossRef] [Google Scholar] [Publisher Link]
[20] Robert M. O’brien, “A Caution Regarding Rules of Thumb for Variance Inflation Factors,” Quality and Quantity, vol. 41, pp. 673-690, 2007.
[CrossRef] [Google Scholar] [Publisher Link]
[21] Google Trends, Google, 2023, [Online]. Available: https://trends.google.com/trends/
[22] Google Ads Dashboard, Google, 2023, [Online]. Available: https://ads.google.com/home/
[23] Audience Score, Rotten Tomatoes, 2023, [Online]. Available: https://www.rottentomatoes.com/
[24] IMDb Ratings, IMDb, 2023, [Online]. Available: https://www.imdb.com/
[25] Netflix Original Series Release Dates, Netflix Media Center, Netflix, 2023, [Online]. Available: https://media.netflix.com/en/
[26] Historical Data:Netflix, Inc. (NFLX), Yahoo Finance, Yahoo, 2023, [Online]. Available: https://finance.yahoo.com/quote/NFLX/history/