Using Machine Learning to Predict Sales Conditional on Bid Acceptance
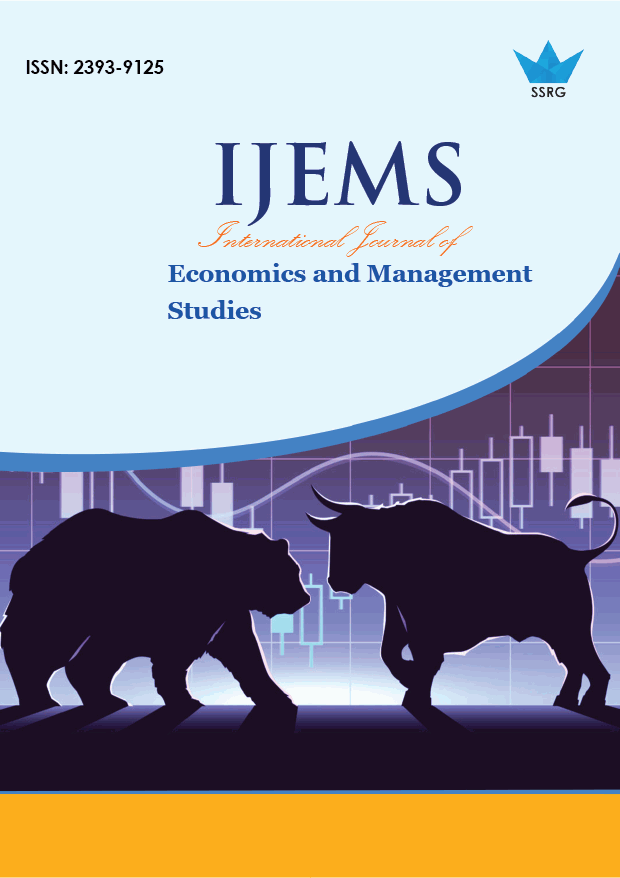
International Journal of Economics and Management Studies |
© 2019 by SSRG - IJEMS Journal |
Volume 6 Issue 11 |
Year of Publication : 2019 |
Authors : Barry E. King, Jason Davidson |
How to Cite?
Barry E. King, Jason Davidson, "Using Machine Learning to Predict Sales Conditional on Bid Acceptance," SSRG International Journal of Economics and Management Studies, vol. 6, no. 11, pp. 1-3, 2019. Crossref, https://doi.org/10.14445/23939125/IJEMS-V6I11P101
Abstract:
A North American provider of vehicle parking solutions seeks to predict if a bid will be successful and, for those that are successful, what will be the cumulative sales revenue. Both traditional statistical methods and machine learning algorithms were employed. The machine learning techniques performed better than the statistical methods. There is no statistically significant difference between random forest and extreme gradient boosting for either the binary classification task or the regression task.
Keywords:
logistic regression, linear regression, random forest, extreme gradient boosting, Tukey honestly significant test
References:
[1] G. Janson, What Machine Learning Approaches Have Won Most Kaggle Competitions?, Retrieved from https://www.quora.com/What-machine-learning-approacheshave-won-most-Kaggle-competitions.