"An Application in Islamic Financial Sector using Data Mining"
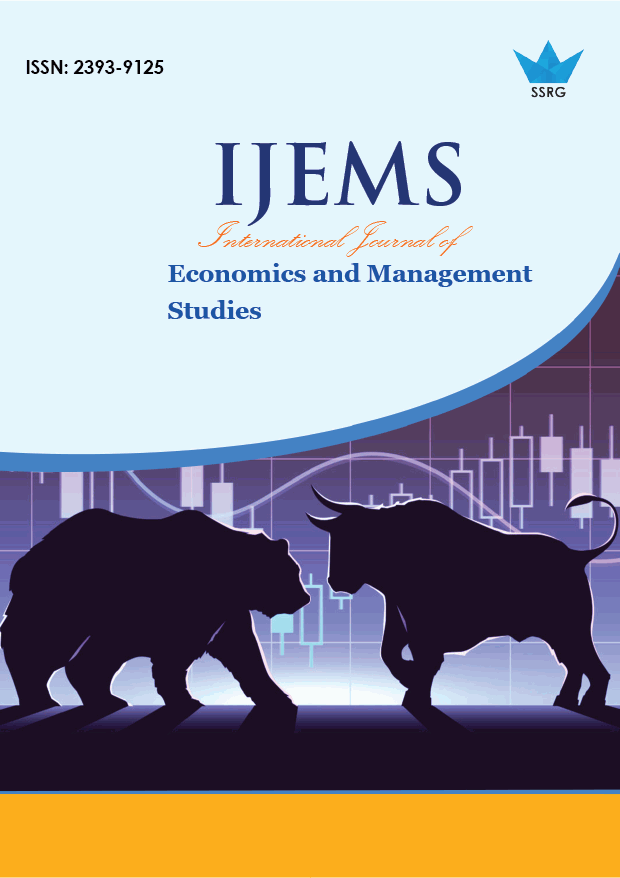
International Journal of Economics and Management Studies |
© 2019 by SSRG - IJEMS Journal |
Volume 6 Issue 11 |
Year of Publication : 2019 |
Authors : Dr. Hassabelrasul Yousuf AL Tom Shihabeldeen |
How to Cite?
Dr. Hassabelrasul Yousuf AL Tom Shihabeldeen, ""An Application in Islamic Financial Sector using Data Mining"," SSRG International Journal of Economics and Management Studies, vol. 6, no. 11, pp. 107-111, 2019. Crossref, https://doi.org/10.14445/23939125/IJEMS-V6I11P112
Abstract:
Islamic finance and capital market is one of the fastest growing segments of international financial markets. Recent innovations in Islamic finance and
capital market have changed the terrain of the landscape of the financial industry. One of them is Islamic securities which are known as Sukuk. The use of Sukuk as the alternative to the existing conventional bond, has become increasingly popular in the last few years. They are used as a means of raising government finance through sovereign Sukuk issues, and means through which companies raise funds by issuing corporate Sukuk. In addition,
theoretically there should be some differences in rating methodologies for bond and Sukuk because these two instruments are different in nature. Thus, it
is the aim of this study to identify the important determinants in Sukuk Rating using data mining approach. The final model is then implemented into
web application, called HZ-RateR.
Keywords:
data mining; decision tree; applications; sukuk; Islamic financial sector
References:
[1] Islamic International Rating Agency (IIRA). (2013). Firduciary Rating. Available: http://www.iirating.com/RatingMethodologiesNavigation.aspx
[2] AAOIFI., Accounting and Auditing Organization of IslamicFinancial Institutions: Shari’ah Standards,2005.
[3] F. Ratings., Fitch’s Approach to Rating Sukuk, 2007.
[4] Malaysian Rating Corporation Berhad (MARC), "Rating Approach To Sukuk: A MARC Perspective," MARC Rating Methodology, Kuala Lumpur2008.
[5] RAM Rating Service Berhad, "Rating of Financial Institutions: Criteria and Methodology," Kuala Lumpur 2008.
[6] R. West, "An Alternative Approach to Predicting Corporate Bond Rating," Journal of Accounting Research, vol. 7, 1970.
[7] G. Pinches and K. Mingo, "A Multivariate Analysis of Industrial Bond Ratings," Journal of Finance,1973.
[8] A. Belkaoui, "Industrial bond ratings: A new look," Financial Management, vol. Autumn 99, 1980.
[9] S. Dutta and S. Shekar, "Bond Rating: A Non-Conservative Application of Neural Networks," IEEE International Conference on Neural Networks, vol. 2, 1988.
[10] R. Chaveesuk, C. Srivaree-ratana, and E. Smith, "Alternative Neural Network Approaches to Corporate Bond Rating," Journal of Engineering Valuation and Cost Analysis, 1997.
[11] H. J., "The Determination of Long Term Credit Sharing With Financial Ratios," Journal of Accounting Research, vol. 4, pp. 44-62, 1966.
[12] J. C. Singleton and A. J. Surkan, "Bond rating with neural networks," in Neural networks in the capital markets, A. Refens, Ed., ed: John Wiley, 1990.
[13] Y. S. Kwon, I. Han, and K. C. Lee, "Ordinal Pairwise Partitioning (OPP) Approach to Neural Networks Training in Bond Rating: Intelligent Systems in Accounting," Finance and Management, vol. 6, 1997.
[14] Z. Huang, H. Chen, C.-J. Hsu, W.-H. Chen, and S. Wu, "Credit Rating Analysis with Support Vector Machine and Neural Networks: A Market Comparative Study," Decision Support System, vol. 37, 2004.
[15] L. Cao, Kian, L. and Z. Jingqing, "Bond rating using support vector machine," Intelligent Data Analysis, vol. 10, 2006.
[16] Y. S. Kwon, I. Han, and K. C. Lee, "Ordinal Pairwise Partitioning (OPP) Approach to Neural Networks Training in Bond Rating," Intelligent Systems in Accounting, Finance and Management, vol. 6, 1997.
[17] P. Hájek and V. Olej, "Credit rating modelling by kernelbased approaches with supervised and semi-supervised learning," Neural Computing and Applications, vol. 20, pp.761-773, 2011.
[18] P. Hajek and K. Michalak, "Feature selection in corporate credit rating prediction," Knowledge-Based Systems, vol. 51,2013.
[19] T. Arundina and M. A. Omar, "Determinant of Sukuk Rating; The Case of Malaysia," Thesis Kulliyah of Economics and Management Science, International Islamic University Malaysia, 2010.
[20] H. Whitten and E. Frank, "Data Mining: Practical Machine Learning Tools and Techniques," 2nd ed: Morgan Kaufman,2005.
[21] D. Delen, C. Kuzey, and A. Uyar, "Measuring firm performance using financial ratios: A decision tree approach," Expert Systems with Applications, vol. 40, 2013.
[22] H. E. Frydman, E. I. Altman, and D.-L. Kao, "Introducing recursive partitioning for financial classification: the case of financial distress.," Journal of Finance, 1985.
[23] Bastos, "Credit scoring with boosted decision trees," Munich Personal RePEc Archive2008.
[24] M. Novotná, "The use of different approaches for credit rating prediction and their comparison," in 6th International
Scientific Conference Managing and Modelling of Financial Risks Ostrava, 2012,.
[25] A. Arzy Soltan and M. Mehrabioun Mohammadi, "A hybrid model using decision tree and neural network for credit scoring problem," Management Science Letters, vol. 2, 2012.
[26] S. Murugiah, "Malaysian Sukuk market hit US$163.5b in 2013, largest in the world, says ADB report," in The Edge Malaysian ed. Kuala Lumpur, 2014.