Brain Tumor Detection Using MRI by Classification and Segmentation
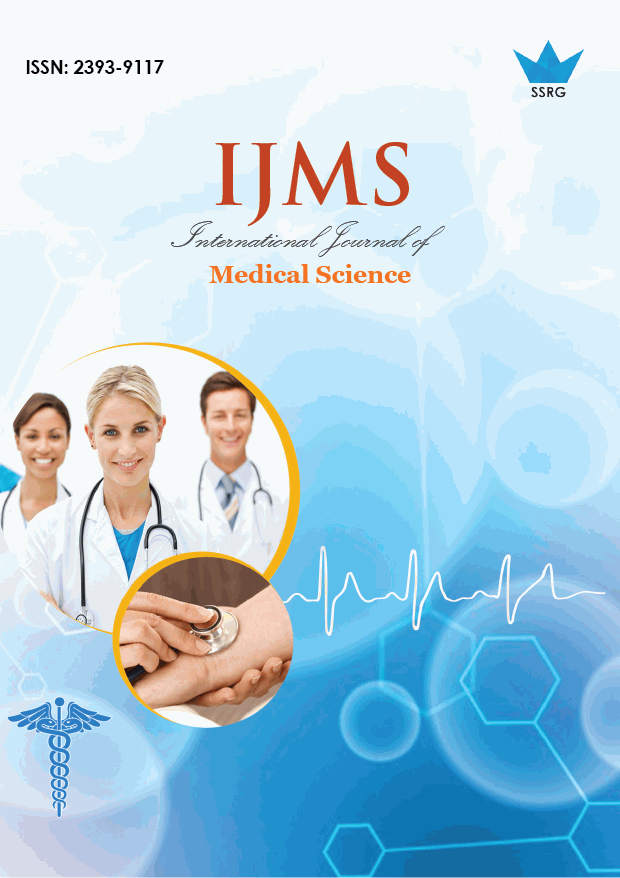
International Journal of Medical Science |
© 2019 by SSRG - IJMS Journal |
Volume 6 Issue 3 |
Year of Publication : 2019 |
Authors : Sindhia, Ramanitharan, Shankar Mahalingam, Soundarya Prithiesh |
How to Cite?
Sindhia, Ramanitharan, Shankar Mahalingam, Soundarya Prithiesh, "Brain Tumor Detection Using MRI by Classification and Segmentation," SSRG International Journal of Medical Science, vol. 6, no. 3, pp. 12-14, 2019. Crossref, https://doi.org/10.14445/23939117/IJMS-V6I3P103
Abstract:
Tumor detection, Image process is employed within the medical tools for detection of tumour, solely MRI pictures aren't able to establish the tumorous region during this paper we tend to ar exploitation K-Means segmentation with pre-processing of image. That contains de-noising by Gaussian filter employed. Conjointly we tend to ar exploitation object labelling for additional elaborate data of tumour region. To form this method associate adjustive we tend to ar exploitation SVM (Support Vector Machine), SVM is employed in unattended manner which is able to use to form and maintain the pattern for future use. Conjointly for patterns we've to seek out the feature to coach SVM. For that here we've decide the feel feature and colour options. It's expected that the experimental results of the projected system can offer higher lead to comparison to different existing systems
Keywords:
Gaussian filter , SVM , GMM , K-MEANS.
References:
[1] E. El-Dahshan, H. Mohsen, K. Revett and A. Salem, "Computer-aided diagnosis of human brain tumor through MRI: A survey and a new algorithm",Expert Systems with Applications, vol. 41, no. 11, pp. 5526-5545, 2014.
[2] N. Nabizadeh and M. Kubat, "Brain tumors detection and segmentation in MR images: Gabor wavelet vs. statistical features",Computers& Electrical Engineering, vol. 45, pp. 286-301, 2015.
[3] R. Gonzalez, R. Woods and B. Masters, "Digital Image Processing, Third Edition",J. Biomed. Opt., vol. 14, no. 2, p. 029901, 2009.
[4] K. Mardia and T. Hainsworth, "A spatial thresholding method for image segmentation", IEEE Transactions on Pattern Analysis and Machine Intelligence, vol. 10, no. 6, pp. 919-927, 1988.
[5] K. Qureshi and S. Hussain, "A Comparative Study of Parallelization Strategies for Fractal Image Compression on a Cluster of Workstations", Int. J. Comput.Methods, vol. 05, no. 03, pp. 463-482, 2008.
[6] M. Wu, C. Lin and C. Chang, "Novel image copy detection with rotating tolerance", Journal of Systems and Software, vol. 80, no. 7, pp. 1057-1069, 2007.
[7] H. Rajini.N and B. Bhavani.R, "Automatic MR Brain Tumor Detection using Possibilistic C-Means and K-Means Clustering with Color Segmentation", International Journal of Computer Applications, vol. 56, no. 2, pp. 11-17, 2012.
[8] B. Sankur, "Survey over image thresholding techniques and quantitative performance evaluation",J. Electron. Imaging, vol. 13, no. 1, p. 146, 2004.
[9] M. Waseem Khan, "A Survey: Image Segmentation Techniques", IJET, pp. 89-93, 2014.
[10] S. Madhukumar and N. Santhiyakumari, "Evaluation of k-Means and fuzzy C-means segmentation on MR images of brain", The Egyptian Journal of Radiology and Nuclear Medicine, vol. 46, no. 2, pp. 475-479, 2015.