Separation from Brain Magnetic Resonance images (MRI) using Multistage Thresholding Technique
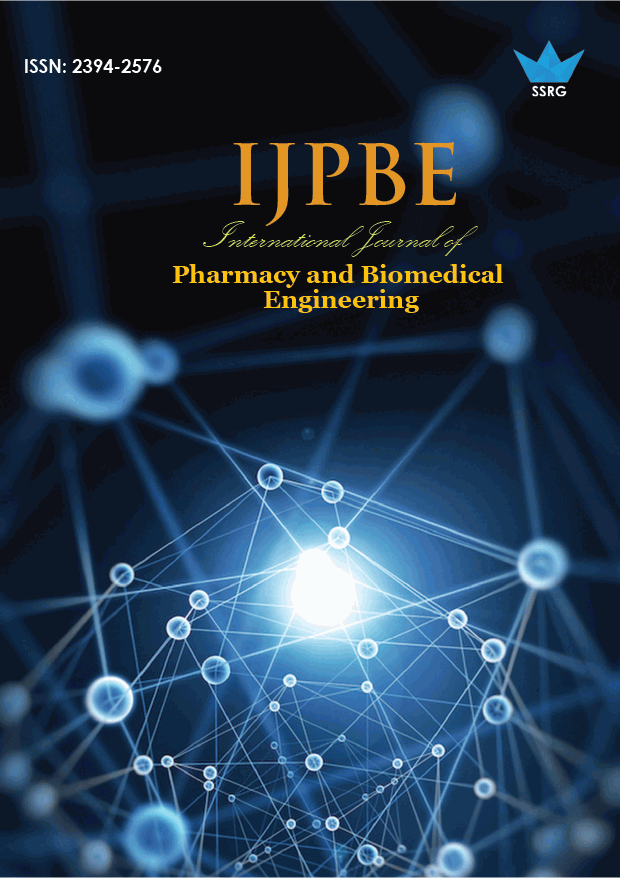
International Journal of Pharmacy and Biomedical Engineering |
© 2015 by SSRG - IJPBE Journal |
Volume 2 Issue 3 |
Year of Publication : 2015 |
Authors : Siyabonga and Shira |
How to Cite?
Siyabonga and Shira, "Separation from Brain Magnetic Resonance images (MRI) using Multistage Thresholding Technique," SSRG International Journal of Pharmacy and Biomedical Engineering, vol. 2, no. 3, pp. 9-13, 2015. Crossref, https://doi.org/10.14445/23942576/IJPBE-V2I3P103
Abstract:
Image separation is a significant task concerned in dissimilar areas from image dispensation to picture examination. One of the simplest methods for image segmentation is thresholding. However, many thresholding methods are based on a bi-level thresholding process. These methods can be extended to form multi-level thresholding. Still, they become computationally expensive since a large number of iterations would be necessary for computing the most select threshold values. To conquer this difficulty, a new process based on a Shrinking Search Space (3S) algorithm is proposed in this paper. The method is applied on statistical bi-level thresholding approaches including Entropy, Cross-entropy, Covariance, and Divergent Based Thresholding (DBT), to attain multilevel thresholding and used for separation from brain MRI images. The paper demonstrates that the collision of the proposed 3S method on the DBT method is more important than the other bi-level thresholding approaches. Comparing the results of using the proposed approach against those of the Fuzzy C-Means (FCM) clustering method demonstrates a better segmentation performance by improving the comparison index from 0.58 in FCM to 0.68 in the 3S method. Also, this method has a lower calculation impediment of around 0.37s with admiration to 157s dispensation time in FCM. In addition, the FCM approach does not always guarantee the convergence, whilst the 3S method always converges to the optimal result.
Keywords:
Medical image processing; Magnetic resonance imaging; Separation; Thresholding; Brain images.
References:
[1] Sezgin M (2004) “Survey over image thresholding techniques and quantitative performance evaluation” Journal of Electronic Imaging 13: 146-165.
[2] Kapur JN, Sahoo PK, Wong AKC (1985) “A new method for grey-level picture thresholding using the entropy of the histogram”. Computer Vision, Graphics, and Image Processing 29: 273-285.
[3] Otsu N (1979) “Threshold selection from grey-level histograms”. IEEE Transactions on Systems, Man, and Cyberbetics 9: 62-66.
[4] Chowdhury MH, Little WD (1995) “Image thresholding techniques”. IEEE Pacific RIM Conference on Communications, Computers, and Signal Processing, Proceedings, Victoria, BC, Canada.
[5] Al-Attas R, El-Zaart A (2007) “Thresholding of medical images using minimum cross-entropy”. IFMBE Proceedings 15: 296-299.
[6] Sahoo PK, Soltani S, Wong AKC, Chen YC (1988) “A survey of thresholding techniques”. Computer Vision, Graphics, and Image Processing 41: 233-260.
[7] Fan SKS, Lin Y (2007) “A multi-level thresholding approach using a hybrid optimal estimation algorithm”. Pattern Recognit Lett 28: 662-669.
[8] Liao PS, Chen TS, Chung PC (2001) “A fast algorithm for multi-level thresholding”. J Inf Sci Eng 17: 713-727.
[9] Huang DY, Wang CH (2009) “Optimal multi-level thresholding using a two-stage Otsu optimization approach”. Pattern Recognit Lett 30: 275-284.
[10] Bezdek JC (1981) “Pattern recognition with fuzzy objective function algorithms”. Norwell, MA: Kluwer, USA