The Sorting and Grading of Red Chilli Peppers (Capsicum annuum L.) Using Digital Image Processing
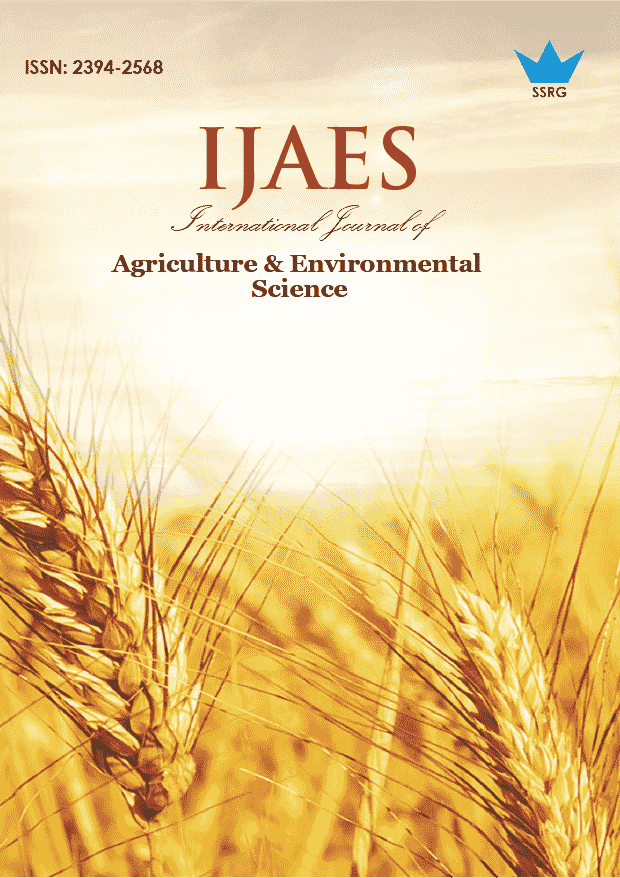
International Journal of Agriculture & Environmental Science |
© 2019 by SSRG - IJAES Journal |
Volume 6 Issue 4 |
Year of Publication : 2019 |
Authors : Nafis Khuriyati, Agung Putra Pamungkas, Anggraito Agung P. |
How to Cite?
Nafis Khuriyati, Agung Putra Pamungkas, Anggraito Agung P., "The Sorting and Grading of Red Chilli Peppers (Capsicum annuum L.) Using Digital Image Processing," SSRG International Journal of Agriculture & Environmental Science, vol. 6, no. 4, pp. 17-23, 2019. Crossref, https://doi.org/10.14445/23942568/IJAES-V6I4P104
Abstract:
Sorting of red chilli pepper manually makes the result unobjective and inconsistent. By using digital image processing and Artificial Neural Network (ANN), we can minimize the subjectivity and inconsistency. The aim of this research was to design ANN architecture for sorting and grading red chilli pepper based on image analyses. Red chilli pepper cv. Helix image was processed using digital image processing and classified using ANN. Images from the two sides of a red chilli that were 20 samples taken in a closed box equipped with a smartphone. The images of red chillies were processed by using MATLAB application that was used to transform RGB image to obtain mark of colour features and texture features. Subsequently, colour and texture feature were chosen to get variables for composing ANN. It was discovered that red, energy, and correlation are variables to compose ANN. This ANN had 3 input cells, 22 hidden layers cells, and 5 output cells and could classify 20 samples of red chilli peppers with 84.46% level of accuracy.
Keywords:
Artificial neural network, digital image processing, grading, red chilli peppers, sorting
References:
[1] FAO, 2017. Food and Agriculture Organization of the United Nations Statistic Division website. [Online]. Available: http://www.fao.org.
[2] Farid, M. & Subekti, N. A., 2012. Tinjauan terhadap Produksi, Konsumsi, Distribusi dan Dinamika Harga Cabe di Indonesia. Buletin Ilmiah Litbang Perdagangan, VOL. 6, No. 2: 211-234.
[3] Alif, S. M. 2017. Kiat Sukses Budidaya Cabai Keriting. Yogyakarta: Bio Genesis.
[4] Gunasekaran, S. 1996. Computer Vision Technology for Food Quality Assurance. Trends in Food Science & Technology, 7 (8), 245-256.
[5] Sandra. 2005. Aplikasi jaringan syaraf tiruan untuk pendugaan mutu mangga megar mecara non-destruktif. Jurnal Teknologi Pertanian,Vol. 6 No. 1 (April 2005) 66-72
[6] Meruliya, T., Dhameliya, P., Patel, J., Panchal, D., Kadam, P., Naik, S., 2015. Image Processing for Fruit Shape and Texture Feature Extraction-Review. International Journal Comput. Appl. (0975-8887) Vol. 129, No. 8: 30-33.
[7] León, K., Mery, D., Pedreschi, F., León, J., 2006. Color measurement in L∗a∗b∗ units from RGB digital images. Food Res. Int. 39, 1084–1091
[8] Payne, A.B., Walsh, K.B., Subedi, P.P., Jarvis, D., 2013. Estimation of mango crop yield using image analysis – segmentation method. Comput. Electron. Agric. 91, 57–64.
[9] Shamili, M., 2019. The estimation of mango fruit total soluble solids using image processing technique. Scientia Holticulture Vol. 294: 383-389.
[10] Ahmad, U. 2010. Aplikasi teknik pengolahan citra dalam analisis non destruktif produk pangan. Jurnal Pangan Vol. 19 No. 1 Maret 2010.
[11] Chung, S., Bereshears L., E., Yoon, J., 2018. Smartphone Near Infrared Monitoring of Plant Stress. Comput Ekectron Agriculture Vol. 154: 93-98
[12] Shrivastava, S., Lee, W., Lee, N., 2018. Culture-free, highly sensitive, quantitative detection of bacteria from minimally processed samples using fluorescence imaging by smartphone. Biosens. Bioelectron. Vol. 109: 90–97.
[13] Hosseinpour, S., Ali H. I., Mortaza., A., 2019. An Intelegent machine vision-based Smartphone App for Beef Quality. Journal Food Engineerig 248: 9-22.
[14] Hermawan, A. 2006. Jaringan Saraf Tiruan Teori dan Aplikasi. Yogyakarta: Penerbit Andi.
[15] Widjanarko, D. 2014. Program Aplikasi Untuk Identifikasi Kematangan Buah Pisang Mas (Musa Paradisiaca l) Berdasarkan Parameter Citra dengan Teknologi Pengolahan Citra Digital. Skripsi Teknologi Industri Pertanian. Universitas Gadjah Mada: Yogyakarta.
[16] Bennedsen, B. S., D. L. Peterson and A. Tabb. 2007. Identifying apple surface defects using principal components analysis and artificial neural networks. American Society of Agricultural and Biological Engineers Vol. 50(6): 2257-2265J.