Water Quality Analysis of Different Water Sources in Kerala, India
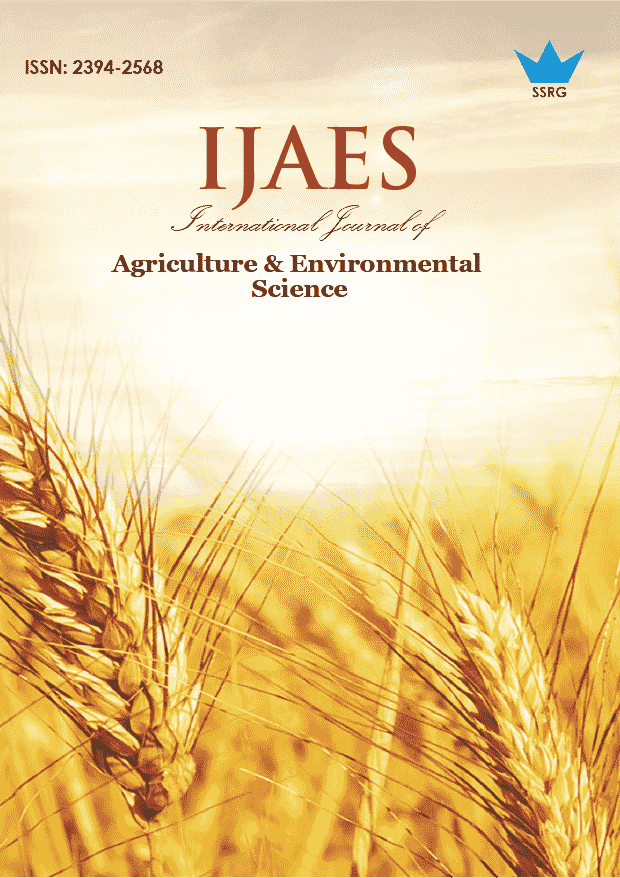
International Journal of Agriculture & Environmental Science |
© 2022 by SSRG - IJAES Journal |
Volume 9 Issue 4 |
Year of Publication : 2022 |
Authors : Remya R S, Ebin Antony |
How to Cite?
Remya R S, Ebin Antony, "Water Quality Analysis of Different Water Sources in Kerala, India," SSRG International Journal of Agriculture & Environmental Science, vol. 9, no. 4, pp. 6-11, 2022. Crossref, https://doi.org/10.14445/23942568/IJAES-V9I4P102
Abstract:
This paper aimed to classify the quality of different water sources in Kerala. All major rivers, lakes, and reservoirs are included in this study. The quality of water is classified into different classes based on the level of pH, Biochemical Oxygen Demand (BOD), Dissolved Oxygen, Electrical Conductivity, and concentration of Total Coliforms in the water sample. For classification purposes, different classification models are proposed. Among these classification models, Naïve Bayes scored 78.79 % accuracy. SVM scored 82.83 % accuracy. The decision Tree Classifier's accuracy in this case study is 93.94 %, XG Boost classifier scored 94.95 % accuracy. Random Forest scored the highest accuracy, i.e., 95.96 %. Also, classification reports of these models are evaluated. On evaluating these results, it can be concluded that Random Forest gives the best results.
Keywords:
Decision Tree, Random Forest, Support Vector Machine, Water Quality Analysis, XG Boost.
References:
[1] Ahmad Z, Rahim N, Bahadori A, Zhang J, “Improving Water Quality Index Prediction in Perak River Basin Malaysia through a Combination of Multiple Neural Networks,” Int. J. River Basin Manag, vol. 15, pp. 79–87, 2017.
[2] Abyaneh H.Z, “Evaluation of Multivariate Linear Regression and Artificial Neural Networks in Prediction of Water Quality Parameters,” J. Environ. Health Sci. Eng, vol. 12, pp. 40, 2014.
[3] Ali M, Qamar A.M, “Data Analysis, Quality Indexing and Prediction of Water Quality for the Management of Rawal Watershed in Pakistan,” In Proceedings of the Eighth International Conference on Digital Information Management (ICDIM), Islamabad, Pakistan, vol. 10, no. 12, pp. 108-113, 2013.
[4] A Cˇ omic´ L, “Neural Network Modeling of Dissolved Oxygen in the Gruža Reservoir,” Serbia, Ecol, Model, vol. 221, pp. 1239– 1244, 2010.
[5] Barzegar, Rahim, Mohammad Taghi, Aalami, Jan, Adamowski, “Short-Term Water Quality Variable Prediction Using a Hybrid CNN– LSTM Deep Learning Model,” Stochastic Environmental Research and Risk Assessment, pp. 1–19, 2020.
[6] Oladipo, Johnson O. et al., “Comparison between Fuzzy Logic and Water Quality Index Methods: A Case of Water Quality Assessment in Ikare Community, Southwestern Nigeria,” Environmental Challenges, vol. 3, pp. 100038, 2021.
[7] Asadollah, Seyed Babak, Seyed, Haji, et al., “River Water Quality Index Prediction And Uncertainty Analysis: A Comparative Study Of Machine Learning Models,” Journal of Environmental Chemical Engineering, vol. 9, no. 1, pp. 104599, 2021.
[8] Ebin Antony, N S Sreekanth, R K Sunil Kumar, Nishanth T, “Data Preprocessing Techniques for Handling Time Series Data for Environmental Science Studies”, International Journal of Engineering Trends and Technology, vol. 69, no. 5, pp. 196-207, 2021. Doi:10.14445/22315381/IJETT-V69I5P227
[9] Rahulraj P V and Ebin Antony, "Changes in Atmospheric Air Quality in the Wake of a Lockdown Related to Covid – 19 in the Capital City of Southern State of India, Kerala – Thiruvananthapuram," SSRG International Journal of Agriculture & Environmental Science, vol. 9, no. 3, pp. 17-24, 2022. Crossref, https://doi.org/10.14445/23942568/IJAES-V9I3P103
[10] Szafron, Duane & Greiner, Russ & Lu, Paul & Wishart, David & Macdonell, Cam & Anvik, John & Poulin, Brett & lu, Zhiyong & Eisner, Roman & Ca, Eisner@cs, Explaining Naïve Bayes Classifications, 2003.
[11] Osuna, Edgar & Freund, Robert & Girosi, Federico, “Support Vector Machines: Training and Applications,” Tech Rep A.I. Memo, no. 1602, 1970.
[12] Bahzad Taha Jijo, “Classification Based on Decision Tree Algorithm for Machine Learning”
[13] Cutler, Adele & Cutler, David & Stevens, John, Random Forests, 2011. Doi: 10.1007/978-1-4419-9326-7_5.
[14] Bentéjac, Candice & Csörgő, Anna & Martínez-Muñoz, Gonzalo, “A Comparative Analysis of XGBoost,” 2019.