Classification of Chlorophyll Concentrations in Coastal Water Using Linear Regression with THEOS Imagery
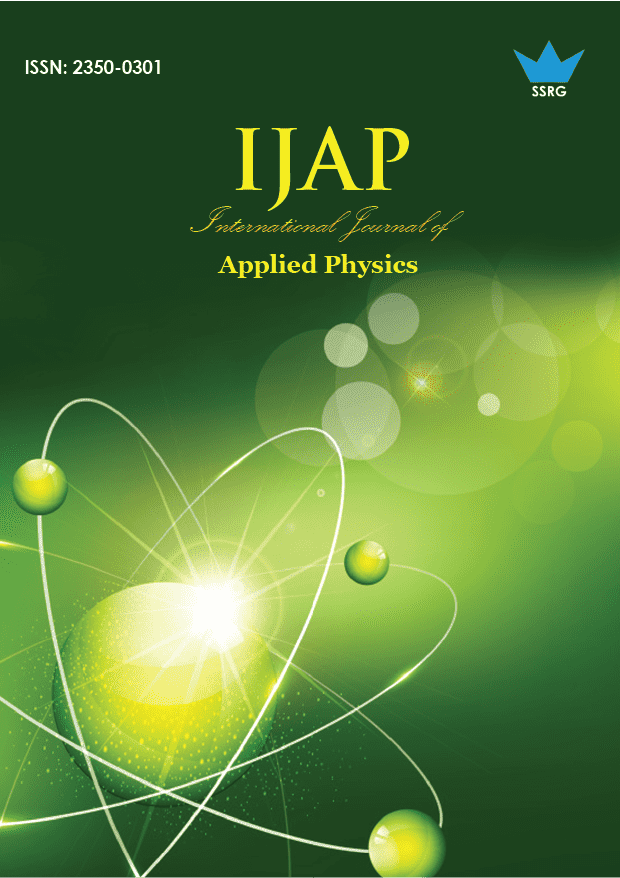
International Journal of Applied Physics |
© 2020 by SSRG - IJAP Journal |
Volume 7 Issue 1 |
Year of Publication : 2020 |
Authors : Ahmed Asal Kzar, Hayder Saad Abdulbaqi, M. Z. MatJafri, H. S. Lim |
How to Cite?
Ahmed Asal Kzar, Hayder Saad Abdulbaqi, M. Z. MatJafri, H. S. Lim, "Classification of Chlorophyll Concentrations in Coastal Water Using Linear Regression with THEOS Imagery," SSRG International Journal of Applied Physics, vol. 7, no. 1, pp. 142-150, 2020. Crossref, https://doi.org/10.14445/23500301/IJAP-V7I1P120
Abstract:
The increasing of water pollutants in the coastline has caused environment problem by the widely usage of the region’s resources for their life. The waters surrounding areas is one of the most effected from these areas especially by chlorophyll. In this study, using of the linear regression for classification then mapping of chlorophyll concentrations through finding the relationship between the chlorophyll concentration and the digital number of an individual band represented by red or green or blue of the adopted satellite (THEOS) for Penang strait, Penang, Malaysia. The validation data were measured from the study area simultaneously with the satellite image acquisition. The results were by selecting one of the three relationships of the three bands. As result, the red band had the best relationship through given the highest coefficient of determination (0.967) that calculated between the two groups of the chlorophyll concentrations: measured (validation data) and calculated by the linear regression. In addition, we tested the new algorithm with noise, it has proved its steadfastness with noisy images over a range of noise levels. Therefore, chlorophyll mapping of water pollution in the adopted study area can be achieved by the linear regression with the based THEOS satellite image.
Keywords:
Chlorophyll; environment; THEOS; image classification; water quality mapping.
References:
[1] Dekker, A.G., Vos, R., and Peters, S.: ‘Analytical algorithms for lake water TSM estimation for retrospective analyses of TM and SPOT sensor data’, International journal of remote sensing, 2002, 23, (1), pp. 15-35
[2] Doxaran, D., Froidefond, J.-M., Lavender, S., and Castaing, P.: ‘Spectral signature of highly turbid waters: Application with SPOT data to quantify suspended particulate matter concentrations’, Remote sensing of Environment, 2002, 81, (1), pp. 149-161
[3] Tassan, S.: ‘An improved in-water algorithm for the determination of chlorophyll and suspended sediment concentration from Thematic Mapper data in coastal waters’, International Journal of Remote Sensing, 1993, 14, (6), pp. 1221-1229
[4] Su, Y.-F., Liou, J.-J., Hou, J.-C., Hung, W.-C., Hsu, S.-M., Lien, Y.-T., Su, M.-D., Cheng, K.-S., and Wang, Y.-F.: ‘A multivariate model for coastal water quality mapping using satellite remote sensing images’, Sensors, 2008, 8, (10), pp. 6321-6339
[5] Syahreza, S., San, L.H., Jafri, M.Z.M., and Mustapha, M.R.: ‘Monitoring surface water quality in coastal area of Penang’, International Proceedings of Chemical, Biological and Environmental Engineering, 2011, 8, pp. 297-300
[6] Asadpour, R., San, L.H., Alashloo, M.M., and Moussavi, S.Y.: ‘A statistical model for mapping spatial distribution of total suspended solid from THEOS satellite imagery over Penang Island, Malaysia’, Journal of Applied Sciences Research, 2012, 8, (1), pp. 271-276
[7] Kzar, A.A., Jafri, M.M., San, L.H., Al-Zuky, A.A., Mutter, K.N., and Al-Saleh, A.H.: ‘A feed-forward Hopfield neural network algorithm (FHNNA) with a colour satellite image for water quality mapping’, in Editor (Ed.)^(Eds.): ‘Book A feed-forward Hopfield neural network algorithm (FHNNA) with a colour satellite image for water quality mapping’ (IOP Publishing, 2016, edn.), pp. 012075
[8] Huang, J., Li, Q., Pontius, R.G., Klemas, V., and Hong, H.: ‘Detecting the dynamic linkage between landscape characteristics and water quality in a subtropical coastal watershed, Southeast China’, Environmental management, 2013, 51, (1), pp. 32-44
[9] Kzar, A.A., Jafri, M., Zubir, M., Mutter, K.N., and Syahreza, S.: ‘A Modified Hopfield Neural Network Algorithm (MHNNA) Using ALOS Image for Water Quality Mapping’, International journal of environmental research and public health, 2016, 13, (1), pp. 92
[10] Nazeer, M., and Nichol, J.E.: ‘Development and application of a remote sensing-based Chlorophyll-a concentration prediction model for complex coastal waters of Hong Kong’, Journal of Hydrology, 2016, 532, pp. 80-89
[11] Forkuor, G., Hounkpatin, O.K., Welp, G., and Thiel, M.: ‘High resolution mapping of soil properties using remote sensing variables in south-western Burkina Faso: a comparison of machine learning and multiple linear regression models’, PloS one, 2017, 12, (1)
[12] Monolisha, S., Platt, T., Sathyendranath, S., Jayasankar, J., George, G., and Jackson, T.: ‘Optical classification of the coastal waters of the Northern Indian Ocean’, Frontiers in Marine Science, 2018, 5, pp. 87
[13] Yadav, S., Yamashiki, Y., Susaki, J., Yamashita, Y., and Ishikawa, K.: ‘CHLOROPHYLL ESTIMATION OF LAKE WATER AND COASTAL WATER USING LANDSAT-8 AND SENTINEL-2A SATELLITE’, International Archives of the Photogrammetry, Remote Sensing & Spatial Information Sciences, 2019
[14] Zhang, F., Li, J., Shen, Q., Zhang, B., Tian, L., Ye, H., Wang, S., and Lu, Z.: ‘A soft-classification-based chlorophyll-a estimation method using MERIS data in the highly turbid and eutrophic Taihu Lake’, International Journal of Applied Earth Observation and Geoinformation, 2019, 74, pp. 138-149
[15] Cairo, C., Barbosa, C., Lobo, F., Novo, E., Carlos, F., Maciel, D., Flores Júnior, R., Silva, E., and Curtarelli, V.: ‘Hybrid Chlorophyll-a Algorithm for Assessing Trophic States of a Tropical Brazilian Reservoir Based on MSI/Sentinel-2 Data’, Remote Sensing, 2020, 12, (1), pp. 40
[16] Hihi, S., Rabah, Z.B., Bouaziz, M., Chtourou, M.Y., and Bouaziz, S.: ‘Prediction of Soil Salinity Using Remote Sensing Tools and Linear Regression Model’, Advances in Remote Sensing, 2019, 8, (3), pp. 77-88
[17] Rodbard, D., and Frazier, G.: ‘[1] Statistical analysis of radioligand assay data’: ‘Methods in enzymology’ (Elsevier, 1975), pp. 3-22
[18] Neter, J., Kutner, M.H., Nachtsheim, C.J., and Wasserman, W.: ‘Applied linear statistical models’, 1996
[19] Shabalin, A.A.: ‘Matrix eQTL: ultra fast eQTL analysis via large matrix operations’, Bioinformatics, 2012, 28, (10), pp. 1353-1358
[20] van Erp, T.G., Hibar, D.P., Rasmussen, J.M., Glahn, D.C., Pearlson, G.D., Andreassen, O.A., Agartz, I., Westlye, L.T., Haukvik, U.K., and Dale, A.M.: ‘Subcortical brain volume abnormalities in 2028 individuals with schizophrenia and 2540 healthy controls via the ENIGMA consortium’, Molecular psychiatry, 2016, 21, (4), pp. 547-553
[21] Al-Zuky, A.A.D., and Kzar, A.A.: ‘Hit noise reduction in some x-ray images’, Al-Nahrain Journal of Science, 2009, 12, (4), pp. 78-86
[22] Saleh Al-amri, S., Kalyankar, N., and Khamitkar, S.: ‘A Comparative Study of Removal Noise from Remote Sensing Image’, arXiv preprint arXiv:1002.1148, 2010
[23] Lee, J., and Hoppel, K.: ‘Noise modeling and estimation of remotely-sensed images’, in Editor (Ed.)^(Eds.): ‘Book Noise modeling and estimation of remotely-sensed images’ (IEEE, 1989, edn.), pp. 1005-1008
[24] Zhu, X.X., and Bamler, R.: ‘Tomographic SAR inversion by $ L_ {1} $-norm regularization—The compressive sensing approach’, IEEE transactions on Geoscience and Remote Sensing, 2010, 48, (10), pp. 3839-3846