Comparative Study of Mathematical Models for the Strength of Bacterial Concrete Using Multiple Regression Analysis and Artificial Neural Network
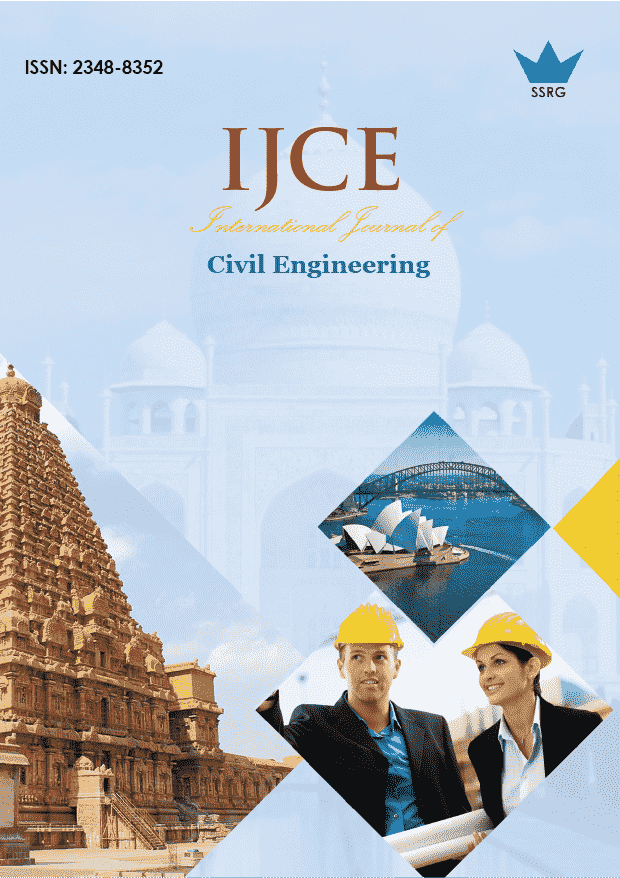
International Journal of Civil Engineering |
© 2023 by SSRG - IJCE Journal |
Volume 10 Issue 12 |
Year of Publication : 2023 |
Authors : C.M. Meera, Subha Vishnudas |
How to Cite?
C.M. Meera, Subha Vishnudas, "Comparative Study of Mathematical Models for the Strength of Bacterial Concrete Using Multiple Regression Analysis and Artificial Neural Network," SSRG International Journal of Civil Engineering, vol. 10, no. 12, pp. 1-8, 2023. Crossref, https://doi.org/10.14445/23488352/IJCE-V10I12P101
Abstract:
This study advocates the adoption of Multiple Regression Analysis (MRA) and Artificial Neural Network (ANN) techniques for predicting concrete behaviour. It underscores these statistical prediction tools’ expeditious and reliable nature, offering valuable insights for subsequent mass concreting endeavours through a swift assessment of concrete behaviour. The research endeavours to construct predictive models using a raw dataset curated from specific studies conducted by various researchers, employing Multiple Regression Analysis tools. In addition to MRA, the study incorporates Artificial Neural Network techniques to train the raw dataset. Subsequently, it utilizes various regression analyses to predict a new model derived from this updated dataset. The study’s primary objective is to discern the combined influence of bacterial addition and concrete grade on the compressive strength of concrete. This holistic approach aims to advance our understanding of the intricate interactions among these variables, contributing to the enhancement of predictive models for concrete behaviour in the context of mass concreting projects. The study also contributes to exploring new dimensions in the research field of bacterial concrete.
Keywords:
Artificial Neural Network, Bacillus subtilis, Bacterial concrete, Behavior prediction, Multiple Regression Analysis.
References:
[1] Linwei Li et al., “Bacterial Technology-Enabled Cementitious Composites: A Review,” Composite Structures, vol. 225, 2019.
[CrossRef] [Google Scholar] [Publisher Link]
[2] Fadi Almohammed et al., “Assessment of Soft Computing Techniques for the Prediction of Compressive Strength of Bacterial Concrete,” Materials, vol. 15, no. 2, pp. 1-17, 2022.
[CrossRef] [Google Scholar] [Publisher Link]
[3] E. Tziviloglou et al., “Bacteria-Based Self-Healing Concrete to Increase Liquid Tightness of Cracks,” Construction and Building Materials, vol. 122, pp. 118-125, 2016.
[CrossRef] [Google Scholar] [Publisher Link]
[4] Salmabanu Luhar, Ismail Luhar, and Faiz Uddin Ahmed Shaikh, “A Review on the Performance Evaluation of Autonomous Self-Healing Bacterial Concrete: Mechanisms, Strength, Durability, and Microstructural Properties,” Journal of Composites Science, vol. 6, no. 1, pp.1- 35, 2022.
[CrossRef] [Google Scholar] [Publisher Link]
[5] Shradha Jena et al., “Impact of Bacillus Subtilis Bacterium on the Properties of Concrete,” Materials Today: Proceedings, vol. 32, pp. 651-656, 2020.
[CrossRef] [Google Scholar] [Publisher Link]
[6] Hassan Amer Algaifi et al., “Insight into the Role of Microbial Calcium Carbonate and the Factors Involved in Self-Healing Concrete,” Construction and Building Materials, vol. 254, 2020.
[CrossRef] [Google Scholar] [Publisher Link]
[7] Palika Chopra, R.K. Sharma, and Maneek Kumar, “Regression Models for Predicting Compressive Strength of Concrete with & without Fly Ash,” International Journal of Latest Trends in Engineering and Technology (IJLTET), vol. 3, no. 4, pp. 400-406, 2014.
[Google Scholar] [Publisher Link]
[8] Keith A. Marill, “Advanced Statistics: Linear Regression, Part II: Multiple Linear Regression,” Academic Emergency Medicine, vol. 11, no. 1, pp. 94-102, 2004.
[CrossRef] [Google Scholar] [Publisher Link]
[9] M.F.M. Zain, and S.M. Abd, “Multiple Regression Model for Compressive Strength Prediction of High-Performance Concrete,” Journal of Applied Sciences, vol. 9, no. 1, pp. 155-160, 2009.
[CrossRef] [Google Scholar] [Publisher Link]
[10] Chereddy Sonali Sri Durga et al., “Performance Studies on the Rate of Self-Healing in Bio Concrete,” Materials Today: Proceedings, vol. 27, pp. 158-162, 2020.
[CrossRef] [Google Scholar] [Publisher Link]
[11] Neela Deshpande, Shreenivas Londhe, and Sushma Kulkarni, “Modeling Compressive Strength of Recycled Aggregate Concrete by Artificial Neural Network, Model Tree and Nonlinear Regression,” International Journal of Sustainable Built Environment, vol. 3, no. 2, pp. 187-198, 2014.
[CrossRef] [Google Scholar] [Publisher Link]
[12] U. Atici., “Prediction of the Strength of Mineral Admixture Concrete Using Multivariable Regression Analysis and An Artificial Neural Network,” Expert Systems with Applications, vol. 38, no. 8, pp. 9609-9618, 2011.
[CrossRef] [Google Scholar] [Publisher Link]
[13] S. Sunil Pratap Reddy et al., “Performance of Standard Grade Bacterial Concrete,” Asian Journal of Civil Engineering (Building and Housing), vol. 11, no. 1, pp. 43-55, 2010.
[Google Scholar] [Publisher Link]
[14] Jasira Bashir et al., “Bio Concrete-the Self-Healing Concrete,” Indian Journal of Science and Technology, vol. 9, no. 47, pp. 1-5, 2016.
[CrossRef] [Google Scholar] [Publisher Link]
[15] M.V. Seshagiri Rao, V. Srinivasa Reddy, and Ch. Sasikala, “Performance of Microbial Concrete Developed Using Bacillus Subtilus JC3,” Journal of the Institution of Engineers (India): Series A, vol. 98, pp. 501-510, 2017.
[CrossRef] [Google Scholar] [Publisher Link]
[16] C. Venkata Siva Rama Prasad, and T.V.S. Vara Lakshmi, “Experimental Investigation on Bacterial Concrete Strength with Bacillus Subtilis and Crushed Stone Dust Aggregate Based on Ultrasonic Pulse Velocity,” Materials Today: Proceedings, vol. 27, pp. 1111-1117, 2020.
[CrossRef] [Google Scholar] [Publisher Link]
[17] Chereddy Sonali Sri Durga et al., “Performance Studies on the Rate of Self-Healing in Bio Concrete,” Materials Today: Proceedings, vol. 27, pp. 158-162, 2020.
[CrossRef] [Google Scholar] [Publisher Link]
[18] Partheeban Pachaivannan et al., “Experimental Analysis of Self Healing Properties of Bacterial Concrete,” Materials Today: Proceedings, vol. 33, pp. 3148-3154, 2020.
[CrossRef] [Google Scholar] [Publisher Link]
[19] G.F. Kheder, A.M. Al Gabban, and S.M. Abid, “Mathematical Model for the Prediction of Cement Compressive Strength at the Ages of 7 and 28 Days within 24 Hours,” Materials and structures, vol. 36, pp. 693-701, 2003.
[CrossRef] [Google Scholar] [Publisher Link]
[20] I.-C. Yeh, “Modeling of Strength of High-Performance Concrete Using Artificial Neural Networks,” Cement and Concrete Research, vol. 28, no. 12, pp. 1797-1808, 1998.
[CrossRef] [Google Scholar] [Publisher Link]
[21] James L. Rogers, “Simulating Structural Analysis with the Neural Network,” Journal of Computing in Civil Engineering, vol. 8, no. 2, pp. 252-265, 1994.
[CrossRef] [Google Scholar] [Publisher Link]
[22] Faeze Khademi et al., “Predicting the Strength of Recycled Aggregate Concrete Using Artificial Neural Network, Adaptive Neuro-Fuzzy Inference System and Multiple Linear Regression,” International Journal of Sustainable Built Environment, vol. 5, no. 2, pp. 355-369, 2016.
[CrossRef] [Google Scholar] [Publisher Link]
[23] Ahmet ÖztaĆ et al., “Predicting the Compressive Strength and Slump of High Strength Concrete Using Neural Network,” Construction and Building Materials, vol. 20, no. 9, pp. 769-775, 2006.
[CrossRef] [Google Scholar] [Publisher Link]