Advancing Accessibility through Rigorous Mathematical Models for Cross-Sensory Translation
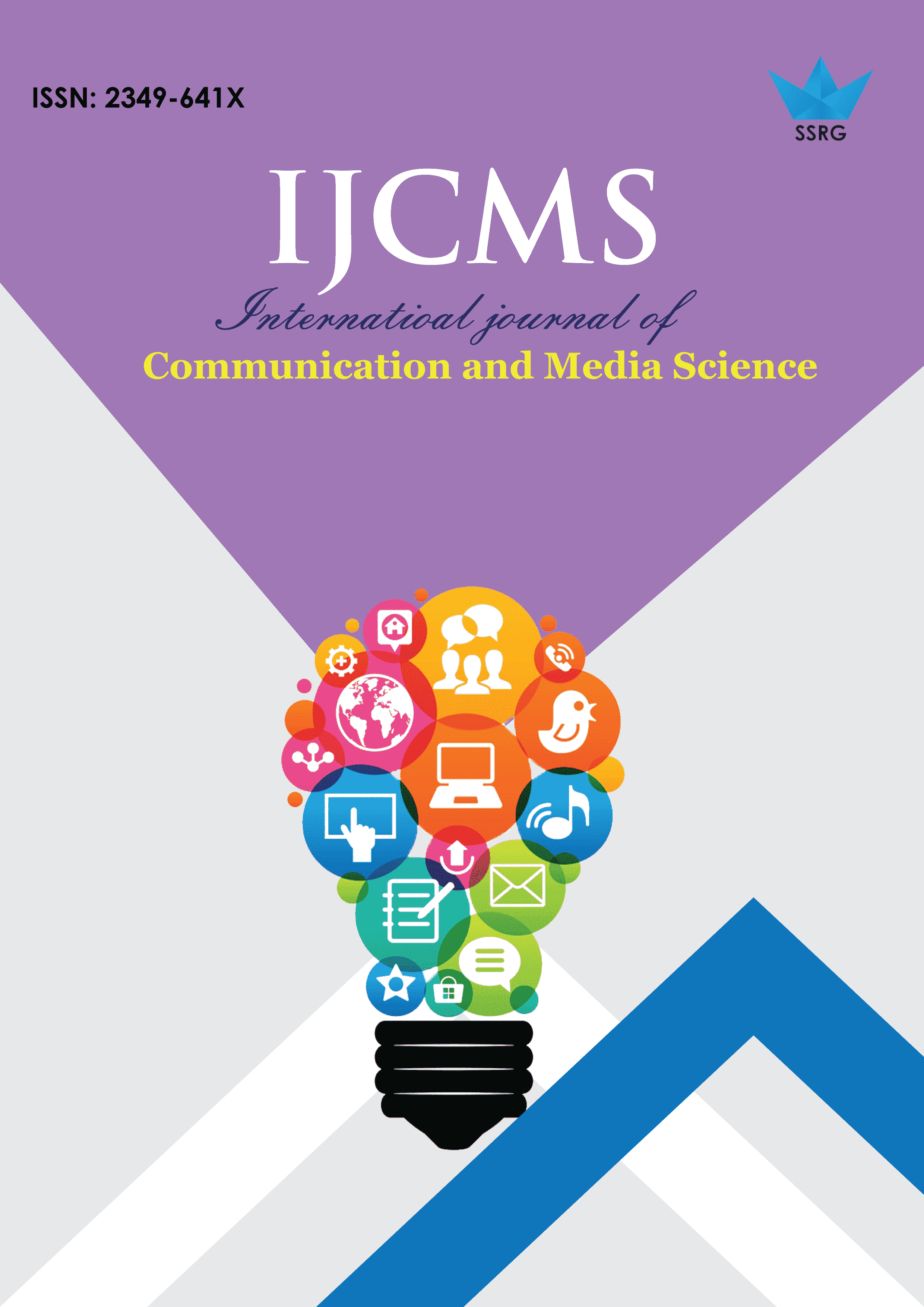
International Journal of Communication and Media Science |
© 2023 by SSRG - IJCMS Journal |
Volume 10 Issue 3 |
Year of Publication : 2023 |
Authors : Taarush Grover |
How to Cite?
Taarush Grover, "Advancing Accessibility through Rigorous Mathematical Models for Cross-Sensory Translation," SSRG International Journal of Communication and Media Science, vol. 10, no. 3, pp. 39-45, 2023. Crossref, https://doi.org/10.14445/2349641X/IJCMS-V10I3P104
Abstract:
This study investigates the crucial relationship between mathematical modelling and accessibility, concentrating on creating and using accurate mathematical models for cross-sensory translation. Accessibility is a vital human right, yet giving people with sensory impairments equal access to knowledge and experiences is ongoingly difficult. A detailed analysis of cross-sensory translation models is paramount to advancing the field, enabling us to fully comprehend the depth of their impact. Transferring information from one sensory modality to another, known as cross-sensory translation, is essential for ensuring inclusivity in both the physical and digital worlds. The theoretical underpinnings, practical uses, difficulties, and prospects of mathematical models in enhancing accessibility through cross-sensory translation are explored in this work. We look at how these models can improve sensory experiences, provide people with sensory impairments more control, and foster an inclusive society.
Keywords:
Cross-Sensory translation, Mathematical models, Accessibility, Sensory impairments, Multimodal perception.
References:
[1] International Classification of Functioning, Disability and Health (ICF), World Health Organization, pp. 1-299, 2001.
[Google Scholar] [Publisher Link]
[2] Amir Amedi et al., “Shape Conveyed by Visual-to-Auditory Sensory Substitution Activates the Lateral Occipital Complex,” Nature Neuroscience, vol. 10, no. 6, pp. 687-689, 2007.
[CrossRef] [Google Scholar] [Publisher Link]
[3] S. Borgo, S. Carpendale, and M. Cox, “A Pattern-Based Framework for Multivariate Visualization,” IEEE Transactions on Visualization and Computer Graphics, vol. 19, no. 12, pp. 2366-2375, 2013.
[4] I.O. Vasilescu, D. Terzopoulos, and T. Spline, “Analysis, Synthesis, and Perception of Voice: A Tensor Representation,” IEEE Transactions on Speech and Audio Processing, vol. 11, no. 3, pp. 221-237, 2003.
[5] Yann LeCun, Yoshua Bengio, and Geoffrey Hinton, “Deep Learning,” Nature, vol. 521, pp. 436-444, 2015.
[CrossRef] [Google Scholar] [Publisher Link]
[6] Brian A. Wandell, and Stelios M. Smirnakis, “Plasticity and Stability of Visual Field Maps in Adult Primary Visual Cortex,” Nature Reviews Neuroscience, vol. 10, no. 12, pp. 873-884, 2009.
[CrossRef] [Google Scholar] [Publisher Link]
[7] Monica Gori, Giulio Sandini, and David Burr, “Development of Visuo-Auditory Integration in Space and Time,” Frontiers in Integrative Neuroscience, vol. 6, pp. 1-8, 2012.
[CrossRef] [Google Scholar] [Publisher Link]
[8] Jack M. Loomis, Roberta L. Klatzky, and Nicholas A. Giudice, Sensory Substitution of Vision: Importance of Perceptual and Cognitive Processing, 1 st ed., Assistive Technology for Blindness and Low Vision, CRC Press, pp. 231-252, 2013.
[Google Scholar] [Publisher Link]
[9] J. Antolík, and J.A. Bednar, “Optimization of Visual Feature Encoding in a Large Population of Retinal Ganglion Cells,” Journal of Neuroscience, vol. 29, no. 44, pp. 13950-13962, 2009.
[10] Hans W. Gellersen, Albrecht Schmidt, and Michael Beigl, “Multi-Sensor Context-Awareness in Mobile Devices and Smart Artefacts,” Mobile Networks and Applications, vol. 7, no. 5, pp. 341-351, 2002.
[CrossRef] [Google Scholar] [Publisher Link]
[11] Luciano Floridi, and Josh Cowls, “A Unified Framework of Five Principles for AI in Society,” Harvard Data Science Review, vol. 1, no. 1, pp. 1-14, 2019.
[CrossRef] [Google Scholar] [Publisher Link]
[12] Convention on the Rights of Persons with Disabilities (CRPD), United Nations, Department of Economic and Social Affairs, Social Inclusion, 2006. [Online]. Available: https://social.desa.un.org/issues/disability/crpd/convention-on-the-rights-of-persons-with-disabilities-crpd
[13] Mikhail A. Lebedev, and Miguel A.L. Nicolelis, “Brain-Machine Interfaces: Past, Present And Future,” Trends in Neurosciences, vol. 29, no. 9, pp. 536-546, 2006.
[CrossRef] [Google Scholar] [Publisher Link]
[14] M.A. Srinivasan, R.H. LaMotte, and W. Vibrotactile, “Perceptual Channels for Tactile Texture,” Psychophysical Journal of Experimental Psychology, vol. 22, no. 6, pp. 1357-1378, 1996.
[15] Ashish Vaswani et al., “Attention is all you need,” Advances in Neural Information Processing Systems, vol. 30, 2017.
[Google Scholar] [Publisher Link]
[16] Connor Shorten, and Taghi M. Khoshgoftaar, “A Survey on Image Data Augmentation for Deep Learning, Journal of Big Data, vol. 6, no. 1, 2019.
[CrossRef] [Google Scholar] [Publisher Link]
[17] M. Tait, and T. Walsh, “Auditory Representation Fidelity for Head-Tracking Virtual Audio Displays,” The Journal of the Acoustical Society of America, vol. 137, no. 4, pp. 2042-2050, 2015.
[18] T. Baltrušaitis, C. Ahuja, and L.P. Morency, “Multimodal Machine Learning: A Survey and Taxonomy,” IEEE Transactions on Pattern Analysis and Machine Intelligence, vol. 41, no. 2, pp. 423-443, 2019.
[CrossRef] [Google Scholar] [Publisher Link]
[19] Y. Lin, and H. Kim, “Improving Multilingual Named Entity Recognition with Person Names,” Proceedings of the 56th Annual Meeting of the Association for Computational Linguistics, vol. 2, pp. 274-280, 2018.
[20] Anna Jobin, Marcello Ienca, and Effy Vayena, “The Global Landscape of AI Ethics Guidelines,” Nature Machine Intelligence, vol. 1, no. 9, pp. 389-399, 2019.
[CrossRef] [Google Scholar] [Publisher Link]