Auto-Scalability in Cloud: A Surveyof Energy and Sla Efficient Virtual Machine Consolidation
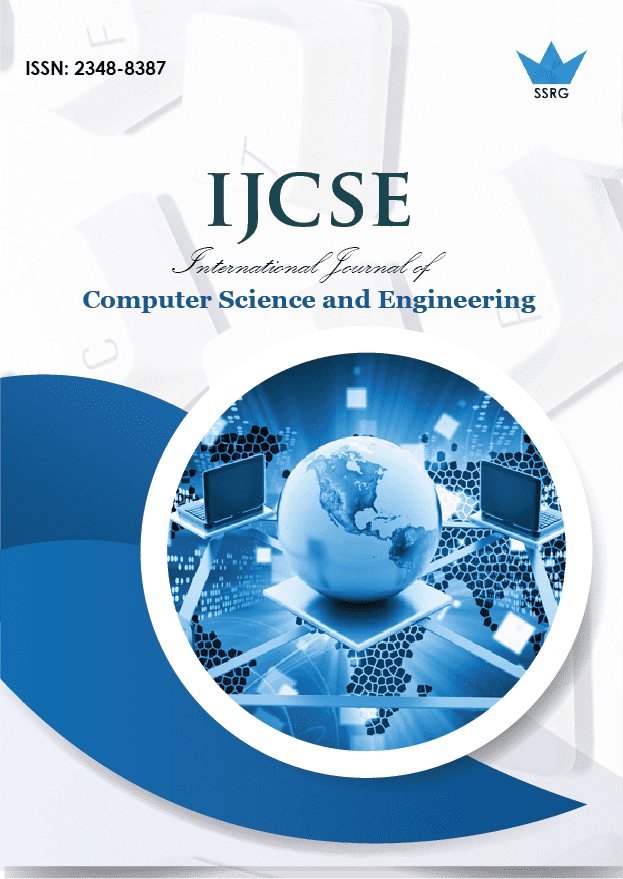
International Journal of Computer Science and Engineering |
© 2016 by SSRG - IJCSE Journal |
Volume 3 Issue 11 |
Year of Publication : 2016 |
Authors : A.Richard William, Dr.J.Senthilkumar |
How to Cite?
A.Richard William, Dr.J.Senthilkumar, "Auto-Scalability in Cloud: A Surveyof Energy and Sla Efficient Virtual Machine Consolidation," SSRG International Journal of Computer Science and Engineering , vol. 3, no. 11, pp. 7-11, 2016. Crossref, https://doi.org/10.14445/23488387/IJCSE-V3I11P102
Abstract:
In cloud computing, the modern cloud data centers are hosting a variety of advanced applications and the IT infrastructure over the recent years because of the demand for computational power infrastructure which are widely used by some of the applications increasing rapidly. Due to the enormous amount of electrical energy consumed by the huge cloud data centers, the operating cost and the emission of carbon dioxide (Co2) produces the high value as a result. In order to reduce the energy consumption and to increase the physical resource utilization in data centers, the most effective way used is a dynamic consolidation of virtual machines (VMs). The main purpose of this paper is to provide a novel method which is used in dynamic virtual machine consolidation. This proposed novel method has outperformed the existing policies in terms of energy consumption, SLA violation and VM migration time by surveying the determination of under loaded hosts, determination of overloaded hosts, and selection of VM and placement of the migrating VMs
Keywords:
cloud computing, consolidation, energy consumption, SLA violation
References:
[1]Jingqi Yang, Chuanchang Liu, Yanlei Shang, Bo Cheng, Zexiang Mao, Chunhong Liu, LishaNiu, Junliang Chen, "A costaware auto-scaling approach using the workload prediction in service clouds",Information System Frontiers,2014.
[2] Samuel A. Ajila, Akindele A. Bankole,"Cloud Client Prediction Models Using Machine Learning Techniques", In Proceedings of 2013 IEEE 37th Annual Computer Software and Applications Conference.
[3] Wang, W., Chen, H., Chen, X, "An availability-aware approach to resource placement of dynamic scaling in clouds", In Proceedings of the 2012 IEEE 5th international conference on cloud computing (pp. 930–931).
[4] John J. Prevost, KranthiManojNagothu, Brian Kelley, Mo Jamshidi, "Prediction of Cloud Data Center Networks Loads Using Stochastic and Neural Models", In Proceedings of the 2011 IEEE 6th international conference on System of Systems Engineering.
[5] ProdipGhosh, Sudip Das, DebabrataSarddar, "Future Load Prediction of Cloud Data Center using Neural Networks", International Journal of Latest Trends in Engineering and Technology, 2015
[6] Baltagi, B.H. (1998). Econometrics (pp. 41-69). Berlin: Springer
[7]Smola, A.J and Scholkopf, B., “A Tutorial on Support Vector Regression” in Statistics and Computing vol 14, pp. 199 – 222, 2004.
[8]Chih-Wei, H. et al, “A practical guide to support vector classification”. Technical report, Department of Computer Science and Information Engineering, National Taiwan University, Taipei, 2003.
[9] Dashevskiy, M. and Luo, Z. "Time series prediction with performance guarantee". IET Communications. Vol. 5, Issue 8, pp. 1044–1051, 2010.
[10] Han, R., Guo, L., Ghanem, M.M., Guo, Y. (2012). Lightweight resource scaling for cloud applications.In Proceedings of the 12th IEEE/ACM international symposium on cluster, cloud and grid computing (pp. 644–651).