Speaker Change Detection Using Teaser-Kaiser Energy Operator and Wavelet Transform
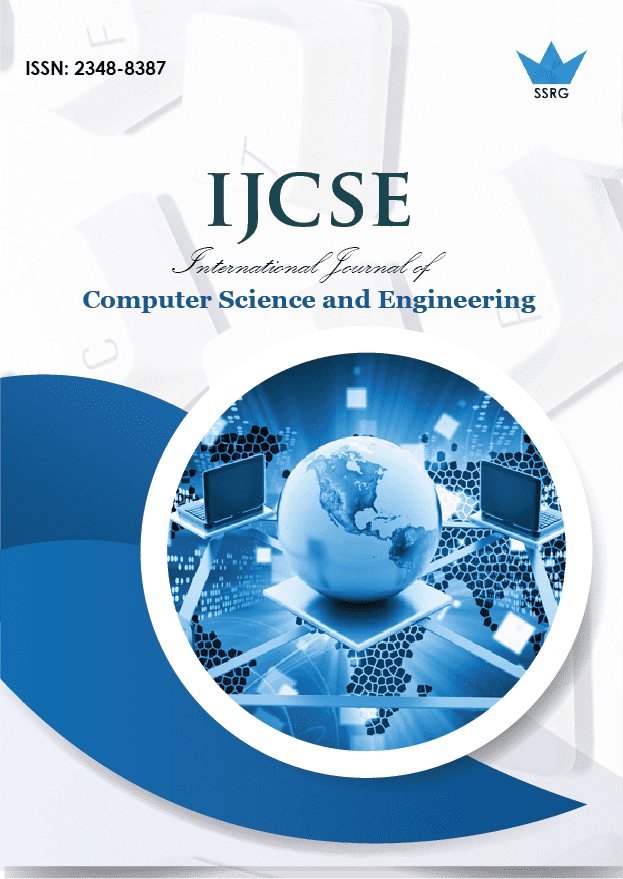
International Journal of Computer Science and Engineering |
© 2017 by SSRG - IJCSE Journal |
Volume 4 Issue 7 |
Year of Publication : 2017 |
Authors : Sukhvinder Kaur, J. S. Sohal |
How to Cite?
Sukhvinder Kaur, J. S. Sohal, "Speaker Change Detection Using Teaser-Kaiser Energy Operator and Wavelet Transform," SSRG International Journal of Computer Science and Engineering , vol. 4, no. 7, pp. 14-18 , 2017. Crossref, https://doi.org/10.14445/23488387/IJCSE-V4I7P103
Abstract:
The aim of this paper is to present an efficient, fast and optimized system that detects speaker change points in multispeaker speech data. It can be used in captioning of TV shows, movies and to split an audio stream into acoustically homogeneous segments, so that every segment ideally contains only one speaker. In this proposed technique, the daubechies 40-wavelet transform is used to compress the audio stream in the ratio of 1:4 with 99% of energy; their features are extracted by 5 level discrete wavelet transform and Teaser Kaiser Energy Operator (TKEO). This method relies on amplitude and frequency variation of the speech signal. Finally, sudden changes of energy in the output of TKEO that corresponds to the speaker change point, is detected by using sliding window. The results are evaluated by F-measure and shows that the proposed method gives fast and better results as compared to traditional method without using discrete wavelet transform.
Keywords:
F-Measure, Segmentation, Sliding Window, Speaker Change Point, Teaser Kaiser Energy Operator, Wavelet Transform.
References:
1. Margarita Kotti, Vassiliki Moschou, Constantine Kotropoulos, “ Review Speaker segmentation and clustering” Signal Processing 88 pp 1091–1124, 2008.
2. Z. Tufekci and J.N. Gowdy, “Feature extraction using discrete wavelet transform for speech recognition,” in Proc. IEEE Southeastcon, USA, pp. 116-123, 2000.
3. Da Wu, J and B Fu Lin, “Speaker Identification using discrete wavelet packet transform technique with irregular decomposition”, Expert System with Applications 36, pp 3136- 3143, 2009, DOI:10.1016/j.eswa.2008.01.038
4. I. Daubechies, “Orthonormal bases of compactly supported wavelets”, Commun. on Pure and Appl. Math., Vol. 41, pp. 909- 996, Nov. 1988.
5. J.F. Kaiser, “On a simple algorithm to calculate the ‘energy’ of a signal”, Proceedings of the IEEE ICASSP-90, Albuquerque, NM, pp-381-384, April 1990.
6. R. Agarwal and J. Gotman, “Adaptive Segmentation of Electroencephalographic Data Using a Nonlinear Energy Operator” Proc. IEEE International Symposium on Circuits and Systems (ISCAS'99), vol. 4, pp. 199-202, 1999.
7. Jitendra Ajmera, Iain Mccowan, And Hervé Bourlard, “Robust Speaker Change Detection”, IEEE Signal Processing Letters,Vol. 11, No. 8, pp 649-651, August 2004