Review of Fuzzy Decision Tree: An improved Decision Making Classifier
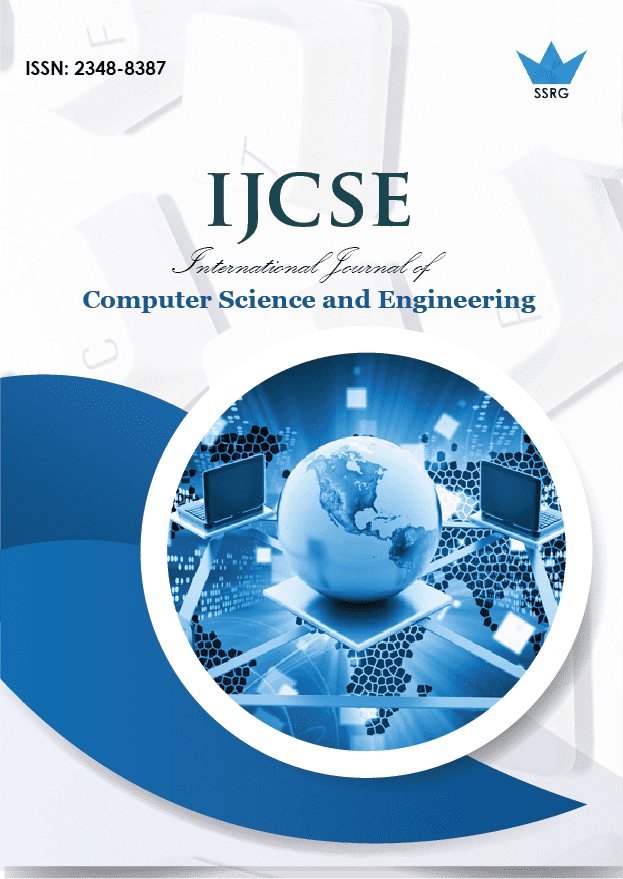
International Journal of Computer Science and Engineering |
© 2014 by SSRG - IJCSE Journal |
Volume 1 Issue 9 |
Year of Publication : 2014 |
Authors : Vinita A. Gupta, Sunita Soni |
How to Cite?
Vinita A. Gupta, Sunita Soni, "Review of Fuzzy Decision Tree: An improved Decision Making Classifier," SSRG International Journal of Computer Science and Engineering , vol. 1, no. 9, pp. 1-5, 2014. Crossref, https://doi.org/10.14445/23488387/IJCSE-V1I9P106
Abstract:
Over the years, various methodologies have been investigated and proposed to deal with continuous or numeric data which is very common in any application. With the increasing popularity of fuzzy representation, researchers have proposed to utilize fuzzy logic in decision trees to deal with the situations. This paper presents a survey of current methodology to design FDT (Fuzzy Decision Tree), various issues and applications. The author conclude that fuzzy decision making using decision tree is an emerging technique in terms of applications and there is a enough scope of research in this area.
Keywords:
Continuous data, Decision tree, Fuzzy logic, Fuzzy decision tree, numeric data,
References:
1.Umano, M., Okamoto, H., Hatono, I., Tamura, H., Kawachi, F., Umedzu, S. Kinoshita, J Fuzzy decision trees by fuzzy ID3 algorithm and its application to diagnosis systems, Fuzzy Systems, IEEE World Congress on Computational Intelligence., Proceedings of the Third IEEE Conference on 1994.
2. Tao Wang, Zhoujun Li, Yuejin Yan, Huowang Chen, A Survey of Fuzzy Decision Tree Classifier Methodology, Fuzzy Information and Engineering Advances in Soft Computing Volume 40, 2007, pp 959-968.
3. Cezary Z. Janikow, Fuzzy Decision Trees: Issues and Methods , Fuzzy Decision Trees: Issues and Methods.
4. Daveedu Raju Adidela, Jaya Suma. , Lavanya Devi. G, Construction of Fuzzy Decision Tree using Expectation Maximization Algorithm, International Journal of Computer Science and Management Research Vol 1 Issue 3 October 2012, ISSN 2278-733X.
5. A.P. Dempster, N.M. Laird, and D. B. Rubin, “Maximum likelihood from incomplete data via the EM algorithm,” Journal of the Royal Statistical Society, Series B, vol. 39, no. 1, pp. 1-38, 1977. 6. Umer Khan, Hyunjung Shin, Jong Pill Choi, Minkoo Kim, wFDT - Weighted Fuzzy Decision Trees for Prognosis of Breast Cancer Survivability
7. Vitaly Levashenko, Elena Zaitseva, Fuzzy Decision Trees in Medical Decision Making Support System, Proceedings of the Federated Conference on Computer Science and Information Systems pp. 213–219
8. Khan MU, Predicting breast cancer survivability using fuzzy decision trees for personalized healthcare, , Conf Proc IEEE Eng Med Biol Soc. 2008;2008:5148-51.
9. J. F. Baldwin, Dong (Walter) Xie, Simple Fuzzy Logic Rules Based on Fuzzy Decision Tree for Classification and Prediction Problem, Intelligent Information Processing II, Volume 163, 2005, pp 175-184.
10. Sushmita Mitra, , Kishori M. Konwar, and Sankar K. Pal, Fuzzy Decision Tree, Linguistic Rules and Fuzzy Knowledge-Based Network: Generation and Evaluation , IEEE TRANSACTIONS ON SYSTEMS, MAN, AND CYBERNETICS—PART C: APPLICATIONS AND REVIEWS, VOL. 32, NO. 4, NOVEMBER 2002.
11. Kavita Sachdeva, Madasu Hanmandlu and Amioy Kumar, Real Life Applications of Fuzzy Decision Tree. International Journal of Computer Applications 42(10):24-28, March 2012. Published by Foundation of Computer Science, New York, USA.
12. Abu-halaweh, N.M, Harrison, R.W., Practical fuzzy decision trees, Computational Intelligence and Data Mining, 2009. CIDM '09. IEEE Symposium on Page(s):211 – 216, 2009.
13. Karol MATIAŠKO, Ján BOHÁČIK, Vitaly LEVASHENKO, Štefan KOVALÍK, Learning Fuzzy Rules From Fuzzy Decision Trees, Journal of Information, Control and Management Systems, Vol. 4, (2006), No. 2143.