Random Forest Regression Model For Estimation of Neonatal Levels In Nigeria
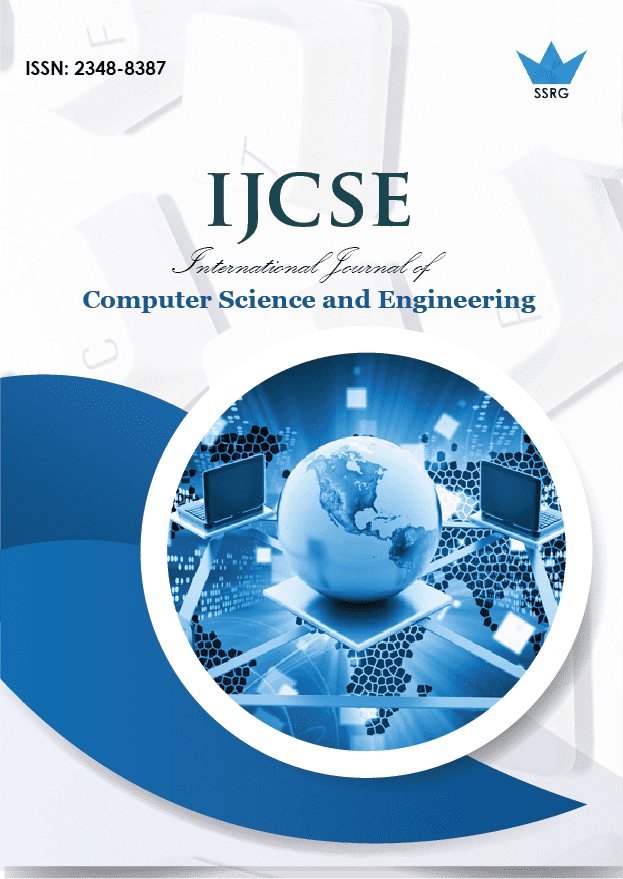
International Journal of Computer Science and Engineering |
© 2020 by SSRG - IJCSE Journal |
Volume 7 Issue 7 |
Year of Publication : 2020 |
Authors : C. Managwu, D.Matthias, N. Nwaibu |
How to Cite?
C. Managwu, D.Matthias, N. Nwaibu, "Random Forest Regression Model For Estimation of Neonatal Levels In Nigeria," SSRG International Journal of Computer Science and Engineering , vol. 7, no. 7, pp. 41-44, 2020. Crossref, https://doi.org/10.14445/23488387/IJCSE-V7I7P107
Abstract:
Health is considered to be a fundamental necessity of all living beings, especially humans, hence its availability is a critical component of the Human Development Index (HDI) measurement of human health. Neonatal (NMR) and infant (IMR) mortality rates are not topics that require too much time or energy for most of us on our day-to-day thinking, but they are also vital to guaranteeing our standard of life. Nigeria's attempts to minimize under-five mortality have been skewed against neglect of neonates in favour of childhood mortality, and as such the literature lacks adequate knowledge
to estimate neonatal rates accurately. Awareness of the rate of neonatal deaths as well as neonatal mortality determinants is important for the design of intervention programs to improve neonatal survival. Hence, this research was conducted to apply Random Forest Regression in predicting neonatal death rates to help better prepare healthcare systems for future occurrences.
This model will be educated using the existing dataset of actual neonatal death rates from 1970 through to 2018.
Keywords:
Neonate, Neonatal Mortality, Big Data, Human Activity Pattern.
References:
[1] Abdulkarim, Kamaluddin & Kajuru, Jibril & Kurfi, Usman & Iliyasu, R. (2017). “Application of Data Mining Technique To Maternal Mortality Prediction in Katsina State, Nigeria”.
[2] Saha, Snehanshu. (2016). “Predicting the direction of stock market prices using Ensemble Learning.” 10.13140/RG.2.1.3202.6482.
[3] Adekitan, A.I. & Salau, Odunayo. (2019). “The impact of engineering students' performance in the first three years on their graduation result using educational data mining”. Heliyon. 5. e01250. 10.1016/j.heliyon.2019.e01250.
[4] Paul, E. ., & Olumide, O. . (2019). “Data Mining Approaches in the Study of the Nigerian Informal Sector”. I. J. Of Advances in Scientific Research and Engineering-IJASRE (ISSN: 2454 - 8006), 5(10), 237-250. https://doi.org/10.31695/IJASRE.2019.33565.
[5] Babbie, Earl R. “The Practice of Social Research. 12th ed. Belmont”, CA: Wadsworth Cengage, 2010; Muijs, Daniel. Doing Quantitative Research in Education with SPSS. 2nd edition. London: SAGE Publications, 2010.
[6] Meyler, Aidan & Kenny, Geoff & Quinn, Terry, 1998. "Forecasting irish inflation using ARIMA models," MPRA Paper 11359, University Library of Munich, Germany.
[7] Box, G. E. P., Jenkins, G. M., & Reinsel, G. C. (1994). “Time series analysis, forecasting and control (3rd Ed.)”. New Jersey: Prentice Hall, Englewood Clifs.
[8] Caiado, J. (2009). “Performance of combined double seasonal univariate time series models for forecasting water consumption”. Munich: Munich University Personal RePEc Archive.
[9] Raschka, S., (2015). “Python Machine Learning”, Packt Publishing Ltd., Birmingham, UK.
[10] Brownlee, J. (2016). "Supervised and Unsupervised Machine Learning Algorithm”. Machine Learning Mastery, Volume 16, Issue 3, March 2016.
[11] Caldwell, John. (1979). “Education as a Factor in Mortality Decline an Examination of Nigerian Data. Population Studies” 33. 10.2307/2173888.
[12] Cech, T. V. (2005). “Principles of water resources: History, development, management, and policy (2nd Ed.)”. Hoboken: John Wiley and Son, Inc,
[13] Cheng, C. (2002). “Study of the inter-relationship between water use and energy conservation for a building”. Energy and Buildings, 34, 261-266.
[14] Chenoweth, J. (2008). “Looming water crisis simply a management problem”. Retrieved on November 20, 2012 from http://www.newscientist.com/ article/mg19926700.100-looming-water-crisis-simply-a-management-problem.html
[15] Cui, F. (2011). “ARIMA models for bank failures: Prediction and comparison”. University of Nevada, Las Vegas.