An Innovative Design of The Internet of Things For Supply Chain Management of Fresh Agricultural Products
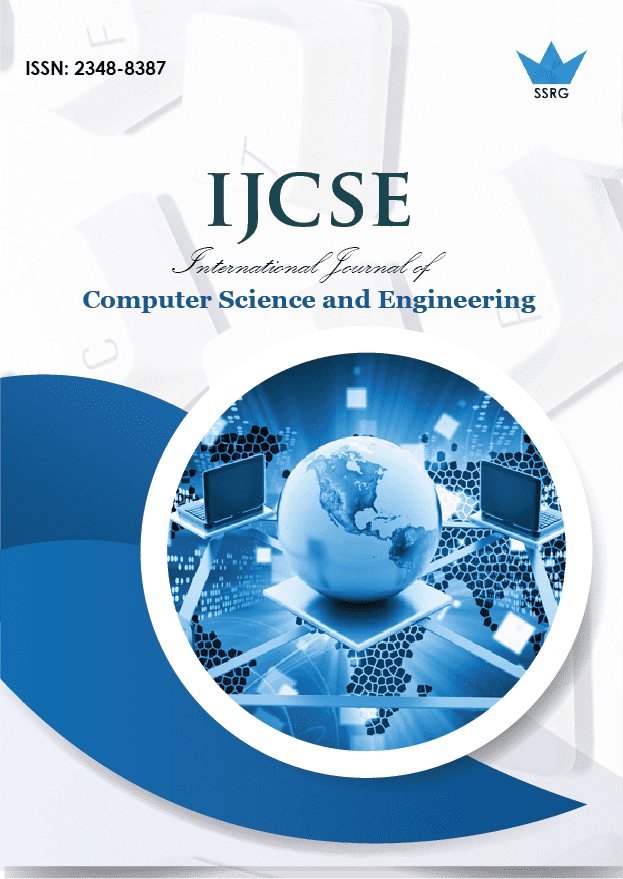
International Journal of Computer Science and Engineering |
© 2020 by SSRG - IJCSE Journal |
Volume 7 Issue 12 |
Year of Publication : 2020 |
Authors : Han-Yu XUE, Shang XIANG, Jun LI |
How to Cite?
Han-Yu XUE, Shang XIANG, Jun LI, "An Innovative Design of The Internet of Things For Supply Chain Management of Fresh Agricultural Products," SSRG International Journal of Computer Science and Engineering , vol. 7, no. 12, pp. 1-4, 2020. Crossref, https://doi.org/10.14445/23488387/IJCSE-V7I12P101
Abstract:
Relevant theories of supply chain management (SCM) and information sharing are summarized, and current situations of SCM of fresh agricultural products are studied. On this basis, an innovative design scheme for the Internet of things (IoT) for SCM of fresh agricultural products is proposed. According to the IoT design framework, an information-sharing mode on the IoT is built. Moreover, the data-collection layer, shearing layer, and application layer in the IoT system for SCM of fresh agricultural products are analyzed. The Markov theory is used to establish the information flow model for the supply chain. Finally, the performance of the established IoT for SCM of fresh agricultural products is analyzed through simulation.
Keywords:
The Internet of Things, Agricultural Products
References:
[1] Xiang S, Xing LN, Wang L, et al. Comprehensive Learning Pigeon-Inspired Optimization with Tabu List, Science China - Information Sciences, 62(7) (2019) Article Number: 070208
[2] Zhang JW, Wang L, Xing LN. Large-scale medical examination scheduling technology based on intelligent optimization. Journal of Combinatorial Optimization, 37(1) (2019) 385-404.
[3] Wang R, Lai SM, Wu GH, et al. Multi-clustering via evolutionary multi-objective optimization, Information Sciences, , 450: (2018) 128-140.
[4] Yi JH, Xing LN, Wang GG, et al. Behavior of Crossover Operators in NSGA-III for Large-scale Optimization Problems, Information Science, (2019), https://doi.org/10.1016/j.ins. 2018.10.005
[5] Gong WY, Wang Y, Cai ZH, et al. Finding multiple roots of nonlinear equation systems via a repulsion-based adaptive differential evolution. IEEE Transactions on Systems, Man, and Cybernetics: Systems. 2018, DOI: 10.1109/TSMC.2018.2828018
[6] Gong WY, Cai ZH. Parameter extraction of solar cell models using repaired adaptive differential evolution. Solar Energy. 94 (2013) 209-220.
[7] Yan XS, Yang KW, Hu CY, Gong WY. Pollution source positioning in a water supply network based on expensive optimization. Desalination and Water Treatment, 110: (2018) 308-318.
[8] Yu PF, Yan XS. Stock price prediction based on deep neural network. Neural Computing & Applications. (2019) DOI: 10.1007/s00521-019-04212-x
[9] Ren T, Li S, Zhang X, & Liu L. Maximum and minimum solutions for a nonlocal p -Laplacian fractional differential system from eco-economical processes. Boundary Value Problems, 2017, (8) (2017) 1-15.
[10] Ren T, Xiao HL, Zhou ZB, et al. The Iterative scheme and the convergence analysis of a unique solution for a singular fractional differential equation from the eco-economic complex system's co-evolution process. Complexity, 2019, (9) (2019) 1-15.
[11] Richards P, Reardon T, Tschirley D, et al. Cities and the future of agriculture and food security: a policy and programmatic roundtable. Food Security, 8(4) (2016) 871~877.
[12] Frayne B, McCordic C. Planning for food secure cities: Measuring the influence of infrastructure and income on household food security in Southern African cities. GEOFORUM, 65: (2015) 1-11.
[13] Eigenbrod C, Gruda N. Urban vegetable for food security in cities, a review. Agronomy for Sustainable Development, 35(2) (2015) 483-498.
[14] Shi N, Chen Y, Huang H, et al. Characteristics of food safety supervision system in foreign countries and its implications for China. Science & Technology of Food Industry, 38(16): (2017) 239-241, 252
[15] Chen TQ, Wang L, Wang JN. Transparent Assessment of the Supervision Information in China's Food Safety: A Fuzzy-ANP Comprehensive Evaluation Method. Journal of Food Quality, (2017) 1-14.
[16] Chen HG. Review on safety supervision of food supply chain. Science & Technology of Food Industry, 34(2) (2013) 49-53, 86.
[17] Jin HS, Liu YS. Risk Identification and Safety Supervision and Management of Agricultural Product Supply Chains. Food Science, 36(13) (2015) 265-271
[18] Verdouw CN, Wolfert J, Beulens AJM, et al. Virtualization of food supply chains with the Internet of things. Journal of Food Engineering, , 176 (2016) 128-136.
[19] Ji GJ, Hu LM, Tan KH. A study on the decision-making of the food supply chain based on big data. Journal of Systems Science and Systems Engineering, 26(2) (2017) 183-198.
[20] Bruzzone AG, Massei M, Longo F, et al. Simulation-Based Design of Innovative Quick Response Processes in Cloud Supply Chain Management for 'Slow Food' Distribution. Communications in Computer and Information Science, 645 (2016) 25-34.
[21] Tu MR. An exploratory study of Internet of Things (IoT) adoption intention in logistics and supply chain management: A mixed research approach. International Journal of Logistics Management, 29(1): (2018) 131-151.
[22] Li B, Li Y.L. Internet of things drives supply chain innovation: a research framework. International Journal of Organizational Innovation, 9(3): (2017) 71-92
[23] Yan B, Wu XH, Ye B, et al. Three-level supply chain coordination of fresh agricultural products on the Internet of Things. Industrial Management & Data Systems, 117(9) (2017) 1842-1865.
[24] Ringwall K. Estimates of the effectiveness of current beef cattle tracking systems. Journal of Animal Science, 83(2) (2005) 118-119.
[25] Trevarthen A. The national livestock identification system: the importance of traceability in e-business. Journal of Theoretical and Applied Electronic Commerce Research, 2(2) (2007) 49-62
[26] Badia-Melis R, Mishra P, Ruiz-García L. Food traceability: New trends and recent advances. A review. Food Control, 57 (2015) 393-401.
[27] Yan R. Optimization approach for increasing revenue of perishable product supply chain with the Internet of Things. Industrial Management & Data Systems, 117(4) (2017) 729-741.
[28] Verdouw CN, Wolfert J, Beulens AJM, et al. Virtualization of food supply chains with the Internet of things. Journal of Food Engineering, 176 (2016) 128-136
[29] Choi TM. A System of Systems Approach for Global Supply Chain Management in the Big Data Era. IEEE Engineering Management Review, 46(1) (2018) 91-97.
[30] Ji GJ, Hu LM, Tan KH. A study on the decision-making of the food supply chain based on big data. Journal of Systems Science and Systems Engineering, 26(2) (2017) 183-198.
[31] Zhao R, Liu YY, Zhang N, et al. An optimization model for green supply chain management uses a big data analytic approach. Journal of Cleaner Production, 142(S.I.) (2017) 1085~1097.
[32] Hu Y. A genetic-algorithm-based remnant grey prediction model for energy demand forecasting. Plos One, 12(10) (2017) 1-11.
[33] Liu Y, Ju W, Zhao J, et al. Product lifecycle-based demand forecasting by using artificial bee colony algorithm optimized two-stage polynomial fitting. Journal of Intelligent & Fuzzy Systems, 31(2) (2016) 825-836.
[34] Adamowski JF. Peak Daily Water Demand Forecast Modeling Using Artificial Neural Networks. Journal of Water Resources Planning & Management, 134(2) (2008) 119-128.
[35] Wang G. Research On Supply Chain Demand Prediction Based On BP Neural Network Algorithm. Inmateh - Agricultural Engineering, 40(2) (2013) 27-34.