Review The Breast Cancer Detection Technique Using Hybrid Machine Learning
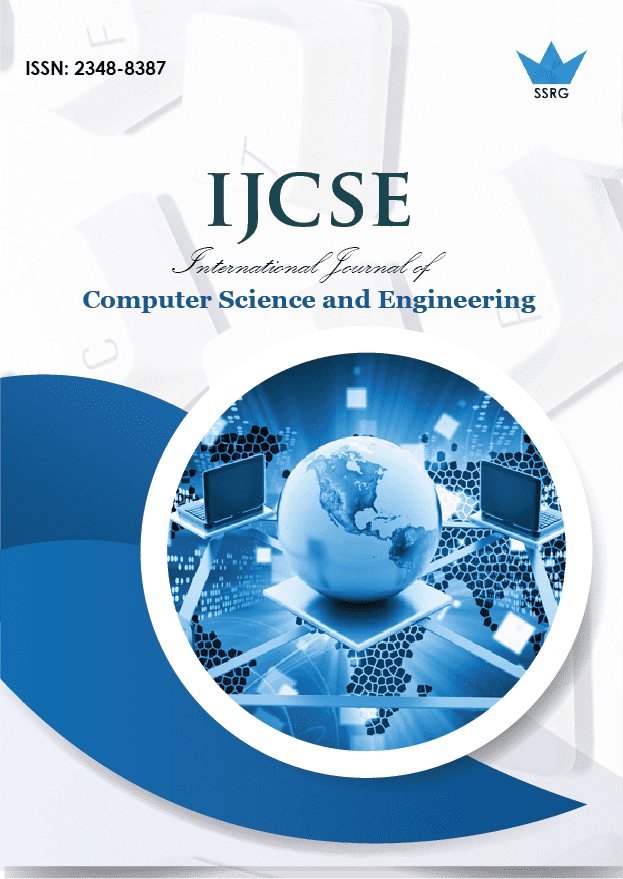
International Journal of Computer Science and Engineering |
© 2021 by SSRG - IJCSE Journal |
Volume 8 Issue 6 |
Year of Publication : 2021 |
Authors : Nidhi Mongoriya, Vinod Patel |
How to Cite?
Nidhi Mongoriya, Vinod Patel, "Review The Breast Cancer Detection Technique Using Hybrid Machine Learning," SSRG International Journal of Computer Science and Engineering , vol. 8, no. 6, pp. 5-8, 2021. Crossref, https://doi.org/10.14445/23488387/IJCSE-V8I6P102
Abstract:
They used different algorithms to identify and diagnose breast cancer; proficient high values incorrectness. Furthest of the proposed algorithms separate were intent in objective detection and diagnosis of breast cancer through appropriate treatment for the corresponding case of breast cancer didn't occupy into their accounts. To perceive and diagnose breast cancer, physicians or radiologists have been used dissimilar images that are distributed by expanding an unusual device called modality accountable to screen somewhat organs of the human body like the brain and breast. These modalities are Digital; respectively, the device issues only one type of image. Practically a big number of the studied papers just contract with one kind of image. Few papers transaction with two types of images but not completely type images that are used in breast cancer. Dissimilar methods already exist to detect breast cancer, like soft computing and simulation techniques, but the greatest precise results were increased by machine learning techniques. Many methods are used, for example, logistic regression, k-Nearest-Neighbor (KNN), support vector machine (SVM); care is therefore being taken about how computational costs for breast cancer diagnoses can be reduced. A creative assembly method is being suggested, in other words (Breast Cancer)
Keywords:
Breast Cancer Detection, hybrid machine learning, Support vector machine
References:
[1] N. Fatima, L. Liu, S. Hong, and H. Ahmed., Prediction of Breast Cancer, Comparative Review of Machine Learning Techniques, and Their Analysis, in IEEE Access, vol. 8, 150360-150376, (2020)doi: 10.1109/ACCESS.2020.3016715.
[2] Sadhukhan, Subham, NityasreeUpadhyay, and PreranaChakraborty., Breast Cancer Diagnosis Using Image Processing and Machine Learning., Emerging Technology in Modelling and Graphics. Springer, Singapore, (2020) 113-127.
[3] Wan, Nathan, et al., Machine learning enables detection of early-stage colorectal cancer by whole-genome sequencing of plasma cell-free DNA., BMC Cancer 19.1 (2019) 832.
[4] Abdel-Ilah, Layla, and Hana Šahinbegović., Using machine learning tool in the classification of breast cancer., CMBEBIH 2017. Springer, Singapore, (2017) 3-8.
[5] Abdel-Zaher, Ahmed M., and Ayman M. Eldeib., Breast cancer classification using deep belief networks., Expert Systems with Applications 46 (2016) 139-144
[6] Jeyanthi, K., and S. Mangai., Ensembled Population Rescaled Differential Evolution with Weighted Boosting for Early Breast Cancer Detection., Mobile Networks and Applications (2019) 1-15.
[7] Aydin, EmineAvşar, and Mümine Kaya Keleş., UWB Rectangular Microstrip Patch Antenna Design in Matching Liquid and Evaluating the Classification Accuracy in Data Mining Using Random Forest Algorithm for Breast Cancer Detection with Microwave, Journal of Electrical Engineering & Technology (2019) 1-10.
[8] Keatmanee, Chadaporn, et al., Automatic initialization for active contour model in breast cancer detection utilizing conventional ultrasound and Color Doppler., 2017 39th Annual International Conference of the IEEE Engineering in Medicine and Biology Society (EMBC). IEEE, (2017).
[9] Khan, A. A., and A. S. Arora., Breast Cancer Detection Through Gabor Filter Based Texture Features Using Thermograms Images., 2018 First International Conference on Secure Cyber Computing and Communication (ICSCCC). IEEE, (2018).
[10] S. Mojrian et al., Hybrid Machine Learning Model of Extreme Learning Machine Radial basis function for Breast Cancer Detection and Diagnosis; a Multilayer Fuzzy Expert System, 2020 RIVF International Conference on Computing and Communication Technologies (RIVF), Ho Chi Minh, Vietnam, (2020) 1-7, doi: 10.1109/RIVF48685.2020.9140744.
[11] K. Polat and U. Sentürk., A Novel ML Approach to Prediction of Breast Cancer: Combining of mad normalization, KMC based feature weighting, and AdaBoostM1 classifier, 2018 2nd International Symposium on Multidisciplinary Studies and Innovative Technologies (ISMSIT), Ankara, (2018) 1-4, doi: 10.1109/ISMSIT.2018.8567245.
[12] A. I. Ahmed and M. M. Hasan., A hybrid approach for decision making to detect breast cancer using data mining and autonomous agent-based on human-agent teamwork, 2014 17th International Conference on Computer and Information Technology (ICCIT), Dhaka, (2014) 320-325, doi: 10.1109/ICCITechn.2014.7073116.
[13] S. Sharma, A. Aggarwal, and T. Choudhury., Breast Cancer Detection Using Machine Learning Algorithms, 2018 International Conference on Computational Techniques, Electronics and Mechanical Systems (CTEMS), Belgaum, India, (2018) 114-118, doi: 10.1109/CTEMS.2018.8769187.Liyang Wei,
[14] Yongyi Yang, R. M. Nishikawa, M. N. Wernick, and A. Edwards., Relevance vector machine for automatic detection of clustered microcalcifications, in IEEE Transactions on Medical Imaging, 24(10) (2005) 1278-1285, doi: 10.1109/TMI.2005.855435.
[15] A. Das, M. S. Nair, and S. D. Peter., Sparse Representation Over Learned Dictionaries on the Riemannian Manifold for Automated Grading of Nuclear Pleomorphism in Breast Cancer, in IEEE Transactions on Image Processing,28(3) (2019) 1248-1260, doi: 10.1109/TIP.2018.2877337.
[16] K. Barhanpurkar, A. S. Rajawat, P. Bedi and O. Mohammed., Detection of Sleep Apnea & Cancer Mutual Symptoms Using Deep Learning Techniques, 2020 Fourth International Conference on I-SMAC (IoT in Social, Mobile, Analytics, and Cloud) (I-SMAC), Palladam, India, (2020) 821-828, doi: 10.1109/I-SMAC49090.2020.9243488.
[17] M. Saha and C. Chakraborty., Her2Net: A Deep Framework for Semantic Segmentation and Classification of Cell Membranes and
Nuclei in Breast Cancer Evaluation, in IEEE Transactions on Image Processing, 27(5) (2018) 2189-2200 doi: 10.1109/TIP.2018.2795742.
[18] M. J. Gangeh, H. Tadayyon, L. Sannachi, A. Sadeghi-Naini, W. T. Tran and G. J. Czarnota., Computer-Aided Theragnosis Using Quantitative Ultrasound Spectroscopy and Maximum Mean Discrepancy in Locally Advanced Breast Cancer, in IEEE Transactions on Medical Imaging, 35(3) 778-790 (2016) doi: 10.1109/TMI.2015.2495246.
[19] A. S. Rajawat, O. Mohammed and P. Bedi., FDLM: Fusion Deep Learning Model for Classifying Obstructive Sleep Apnea and Type 2 Diabetes, 2020 Fourth International Conference on I-SMAC (IoT in Social, Mobile, Analytics, and Cloud) (I-SMAC), Palladam, India, (2020) 835-839, doi: 10.1109/I-SMAC49090.2020.9243553.
[20] A. Li et al., Association Rule-Based Breast Cancer Prevention and Control System, in IEEE Transactions on Computational Social Systems, 6(5) (2019)1106-1114, doi: 10.1109/TCSS.2019.2912629.
[21] Y. M. George, H. H. Zayed, M. I. Roushdy and B. M. Elbagoury., Remote Computer-Aided Breast Cancer Detection and Diagnosis System Based on Cytological Images, in IEEE Systems Journal, 8(3) (2014) 949-964, doi: 10.1109/JSYST.2013.2279415.
[22] S. Sharma and A. S. Rajawat., A secure privacy preservation model for vertically partitioned distributed data, 2016 International Conference on ICT in Business Industry & Government (ICTBIG), Indore, (2016) 1-6, doi: 10.1109/ICTBIG.2016.7892653.
[23] A. S. Rajawat and A. R. Upadhyay., Web Personalization Model Using Modified S3VM Algorithm For developing Recommendation Process, 2nd International Conference on Data, Engineering and Applications (IDEA), Bhopal, India, (2020), 1-6, doi: 10.1109/IDEA49133.2020.9170701.
[24] Rohan K. Gajre, Savita A. Lothe, Santosh G. Vishwakarma, Identification of Brain Tumor using Image Processing Technique: Overviews of Methods, SSRG International Journal of Computer Science and Engineering 3(10) (2016) 48-52.