Detection of Sarcasm through Tone Analysis on video and Audio files: A Comparative Study On Ai Models Performance
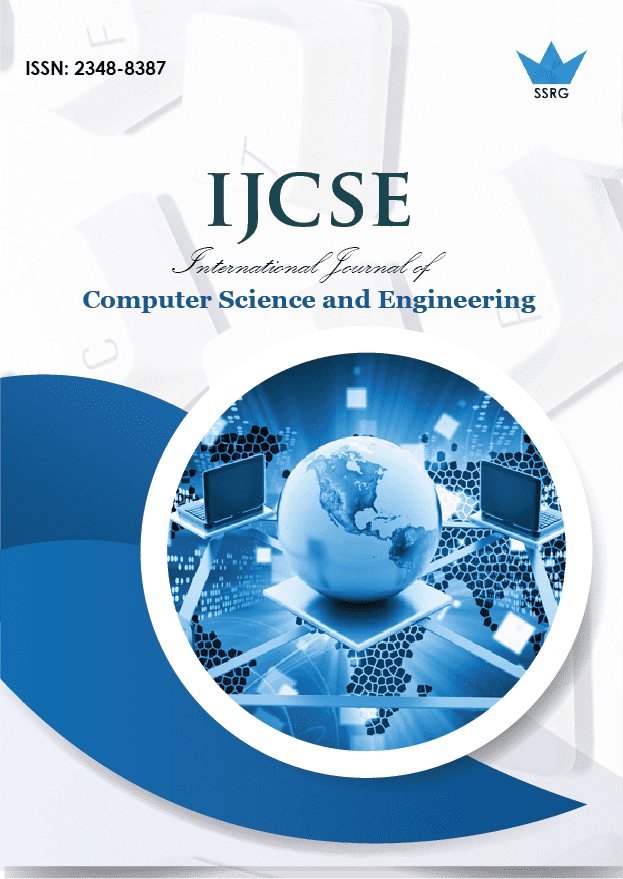
International Journal of Computer Science and Engineering |
© 2021 by SSRG - IJCSE Journal |
Volume 8 Issue 12 |
Year of Publication : 2021 |
Authors : Ayush Jain, Prathamesh Patil, Ganesh Masud, Prof. Sunantha Krishnan, Prof. Vijaya Bharathi Jagan |
How to Cite?
Ayush Jain, Prathamesh Patil, Ganesh Masud, Prof. Sunantha Krishnan, Prof. Vijaya Bharathi Jagan, "Detection of Sarcasm through Tone Analysis on video and Audio files: A Comparative Study On Ai Models Performance," SSRG International Journal of Computer Science and Engineering , vol. 8, no. 12, pp. 1-5, 2021. Crossref, https://doi.org/10.14445/23488387/IJCSE-V8I12P101
Abstract:
During the past few years, there has been a lot of increase in interest in the field of Sentiment Analysis. Sentiment Analysis is used to analyze a given data and help us to understand the sentiment behind the multimedia data, namely text, audio, and video. Unstructured Big Data has its own challenges, and Detection of Sarcasm is one of the major challenges in it. Sarcasm normally signifies the opposite in order to mock or convey contempt, a definition in the Oxford dictionary. Although there has been a lot of research on sarcasm, most of it is on text data, and very few are over audio and video data. Many times, the word or a sentence may not be sarcastic but are spoken sarcastically with a change in the tone or in pitch. In this system, we propose a mechanism to detect sarcasm in the speech by analyzing the audio using pitch frequency, the stress in the pronunciation as a major parameter as an input to the CNN, LSTM, and Bi-Directional LSTM models for audio recognition and classification. This system can be used in social media websites like Twitter, Facebook, Instagram, and YouTube to help users to identify non-sarcastic videos or audios before actually playing or listening to them and wasting their bandwidth.
Keywords:
Sarcasm, Audio mining, Speech recognition, MFCC, Contempt.
References:
[1] Mathur, V. Saxena and S. K. Singh,―Understanding sarcasm in speech using Mel- cepstral frequency coefficient, 2017 7 th International Conference on Cloud Computing, Data Science & Engineering Confluence, Noida, (2017) 728-732. doi: 10.1109/CONFLUENCE.2017.7943246, 2017.
[2] Castro, S., Hazarika, D., Pérez-Rosas, V., Zimmermann, R., Mihalcea, R., and Poria, S., (2019). Towards Multimodal Sarcasm Detection (An_Obviously_ Perfect Paper). [online] arXiv.org. Available at: [Accessed 20 May 2020].
[3] Swami, S., Khandelwal, A., Singh, V., Akhtar, S., and Shrivastava, M., (2018). A Corpus of English-Hindi Code- Mixed Tweets for Sarcasm Detection. [online] arXiv.org. Available at: [Accessed 20 May 2020].
[4] Rakov, R. & Rosenberg, A., Sure, I did the right thing": A system for sarcasm detection in speech. Proceedings of the Annual Conference of the International Speech Communication Association, INTERSPEECH., (2013) 842-846.
[5] X, L. and X, V., (2019). Sarcasm Detection. [online] Web.stanford.edu. Available at:
[Accessed 9 April 2021].
[6] J. Jody, How I Understood: What features to consider while training audio files?, Medium, (2021). [Online]. Available: https://towardsdatascience.com/how-i-understood-what- features-to-consider-while-training-audio-files- eedfb6e9002b. [Accessed: 28- Apr- 2021].