Urban Remote Sensing Image Segmentation using Dense U-Net+
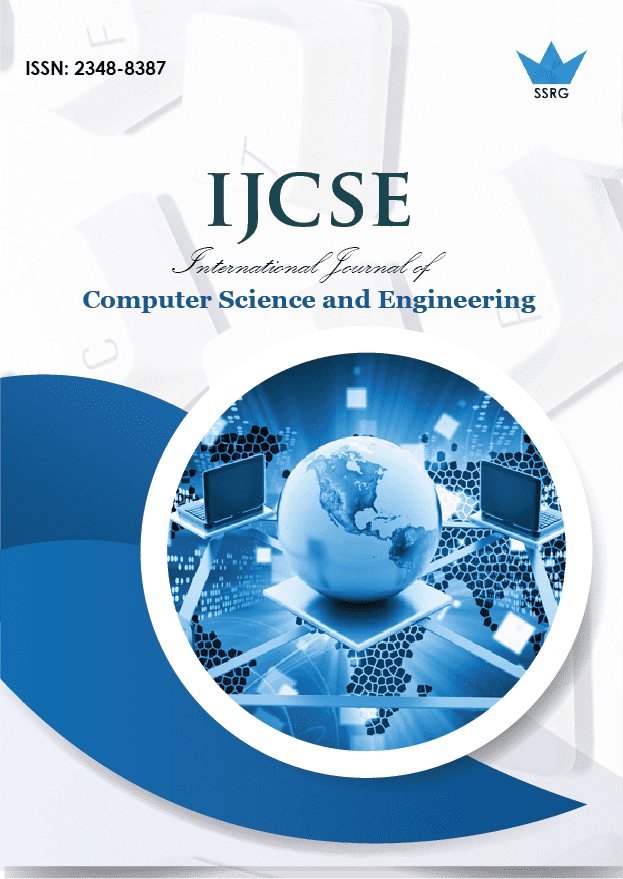
International Journal of Computer Science and Engineering |
© 2022 by SSRG - IJCSE Journal |
Volume 9 Issue 3 |
Year of Publication : 2022 |
Authors : Keerti Maithil, Tasneem Bano Rehman |
How to Cite?
Keerti Maithil, Tasneem Bano Rehman, "Urban Remote Sensing Image Segmentation using Dense U-Net+," SSRG International Journal of Computer Science and Engineering , vol. 9, no. 3, pp. 21-28, 2022. Crossref, https://doi.org/10.14445/23488387/IJCSE-V9I3P103
Abstract:
For a long time, man has been dreaming that we should make such a machine with human-like intelligence, the power to understand like a human and can think like a human. One of the fascinating ideas was to give computers the ability to see and interpret the world around them. The concept of computer vision is based on training a computer, which processes an image to understand and analyze it at a pixel level. Technically, machines attempt to retrieve visual information, handle it, and interpret results through special software algorithms. An important subject within computer vision is image segmentation. Image Segmentation is a process of identifying objects or boundaries to simplify an image and efficiently analyzing it by dividing the image into different regions based on the characteristics of pixels. The existing U-net-based segmentation model and its other variants are the deep learning module design, especially for biomedical image segmentation; initially, it was proposed for cell segmentation. This work finds a new application area: Urban Remote Sensing Image Segmentation using the Dense U-Net+ model. DenseU-Net+ is a powerful form of the U–net architecture inspired by DenseNet. The imbalance is a serious problem in the remote sensing image segmentation class. Another one is that segmentation of large objects in the image is easy, but for small objects, segmentation causes difficulties.
Keywords:
Image segmentation, Computer vision, DenseNet, U-Net, DenseU-Net.
References:
[1] Peng Shuai Yin et al., “Deep Guidance Network for Biomedical Image Segmentation,” IEEE Access, vol. 8, 2020.
[CrossRef] [Google Scholar] [Publisher Link]
[2] Rongsheng Dong, Xiaoquan Pan, and Fengying Li, “Dense U-Net-Based Semantic Segmentation of Small Objects in Urban Remote Sensing Images,” IEEE Access, vol. 7, 2019.
[CrossRef] [Google Scholar] [Publisher Link]
[3] Mahnoor Ali et al., “Brain Tumor Image Segmentation using Deep Networks,” IEEE Access, vol. 8, 2020.
[CrossRef] [Google Scholar] [Publisher Link]
[4] Takafumi Nemoto et al., “Efficacy Evaluation of 2D, 3D U-Net Semantic Segmentation and Atlas-Based Segmentation of Normal Lungs Excluding the Trachea and Main Bronchi,” Journal of Radiation Research, vol. 61, no. 2, pp. 257–264, 2020.
[CrossRef] [Google Scholar] [Publisher Link]
[5] Wei Guo et al., “Double U-Nets for Image Segmentation by Integrating the Region and Boundary Information,” IEEE Access, vol. 9, 2021.
[CrossRef] [Google Scholar] [Publisher Link]
[6] Santosh Jangid, and P S Bhatnagar, “Semantic Image Segmentation Using Deep Convolutional Neural Networks and Super-Pixels,” International Journal of Applied Engineering Research, vol. 13, no. 20, pp. 14657-14663, 2018.
[Google Scholar] [Publisher Link]
[7] Ameya Wagh et al., “Semantic Segmentation of Smartphone Wound Images: Comparative Analysis of AHRF and CNN-Based Approaches,” IEEE Access,vol. 8, 2020. [CrossRef] [Google Scholar] [Publisher Link]
[8] Shuchao Chen et al., “U-Net Plus: Deep Semantic Segmentation for Esophagus and Esophageal Cancer in Computed Tomography Images,” IEEE Access, vol. 7, 2019.
[CrossRef] [Google Scholar] [Publisher Link]
[9] Xiaoqiang WU, and Lng Zhao, “Study on Iris Segmentation Algorithm Based on Dense U-Net,” IEEE Access, vol. 7, 2019.
[CrossRef] [Google Scholar] [Publisher Link]
[10] Wataru Ohyama, Masakazu Suzuki, and Seiichi Uchida, “Detecting Mathematical Expressions in Scientific Document Images Using a U-Net Trained on A Diverse Dataset,” IEEE Access, vol. 7, pp. 144030 – 144042, 2019.
[CrossRef] [Google Scholar] [Publisher Link]
[11] Nhian Siddique et al., “U-Net and Its Variants for Medical Image Segmentation: A Review of Theory and Applications,” IEEE Access, vol. 9, 2021.
[CrossRef] [Google Scholar] [Publisher Link]
[12] Leilei Xu et al., “HA U-Net: Improved Model for Building Extraction From High-Resolution Remote Sensing Imagery,” IEEE Access, vol. 9, 2020.
[CrossRef] [Google Scholar] [Publisher Link]
[13] Neil Micallef, Dylan Seychell, and Claude J. Bajada, “Exploring the U-Net++ Model for Automatic Brain Tumor Segmentation,” IEEE Access, vol. 9, 2021.
[CrossRef] [Google Scholar] [Publisher Link]
[14] Yaohui Liu et al., “Automatic Building Extraction on High-Resolution Remote Sensing Imagery Using Deep Convolutional Encoder-Decoder With Spatial Pyramid Pooling,” IEEE Access, vol. 7, 2019.
[CrossRef] [Google Scholar] [Publisher Link]
[15] Binge Cui, Xin Chen, and Yan Lu, “Semantic Segmentation of Remote Sensing Images Using Transfer Learning and Deep Convolutional Neural Network with Dense Connection,” IEEE Access, vol. 8, 2019.
[CrossRef] [Google Scholar] [Publisher Link]
[16] Teerapong Panboonyue et al., “Transformer-Based Decoder Designs for Semantic Segmentation on Remotely Sensed Images,” Remote Sensing, vol. 13, pp. 5100, 2021.
[CrossRef] [Google Scholar] [Publisher Link]
[17] Tuan Linh Giang et al., “U-Net Convolutional Networks for Mining Land Cover Classification Based on High-Resolution UAV Imagery,” IEEE Access, vol. 8, 2020. [CrossRef] [Google Scholar] [Publisher Link]
[18] Rui Li et al., “MACU-Net for Semantic Segmentation of Fine-Resolution Remotely Sensed Images,” Electrical Engineering and Systems Science, vol. 19, 2022.
[CrossRef] [Publisher Link]
[19] Jian Huang et al., “Object-Level Remote Sensing Image Augmentation Using U-Net-Based Generative Adversarial Networks,” Wireless Communications and Mobile Computing, vol. 2021, 2021.
[CrossRef] [Google Scholar] [Publisher Link]
[20] Hafiz Sami Ullah, Muhammad Hamza Asad, and Abdul Bais, “End to End Segmentation of Canola Field Images Using Dilated U-Net,” IEEE Access, vol. 9, 2021.
[CrossRef] [Google Scholar] [Publisher Link]
[21] Mo Han et al., “Automatic Segmentation of Human Placenta Images with U-Net,” IEEE Access, vol. 7, 2019.
[CrossRef] [Google Scholar] [Publisher Link]
[22] Xiaolong Liu, Zhidong Deng, and Yuhan Yangn, “Recent Progress in Semantic Image Segmentation,” Artificial Intelligence Review, 2018.
[CrossRef] [Google Scholar] [Publisher Link]
[23] Shervin Minaee et al., “Image Segmentation Using Deep Learning: A Survey,” IEEE Transactions on Pattern Analysis and Machine Intelligence, vol. 44, no. 7, 2022.
[CrossRef] [Google Scholar] [Publisher Link]
[24] Edy Irwansyah, and Yaya Heryadi, “Semantic Image Segmentation for Building Detection in Urban Areas with Aerial Photograph Image Using U-Net Models,” 2020 IEEE Asia-Pacific Conference on Geoscience, Electronics and Remote Sensing Technology (AGERS), 2021.
[CrossRef] [Google Scholar] [Publisher Link]
[25] Ruyue Xin, Jiang Zhang, and Yitong Shao, “Complex Network Classification with Convolutional Neural Network,” Tsinghua Science and Technology, vol. 25, no. 4, 2020.
[CrossRef] [Google Scholar] [Publisher Link]