Detection of Brain Cancer using Machine Learning Techniques a Review
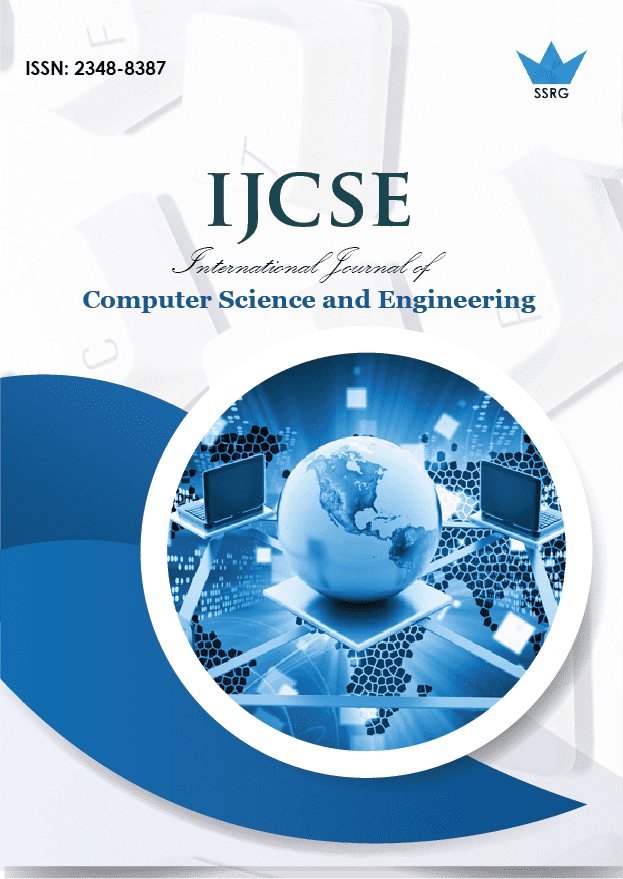
International Journal of Computer Science and Engineering |
© 2022 by SSRG - IJCSE Journal |
Volume 9 Issue 9 |
Year of Publication : 2022 |
Authors : G. R. Meghana, Suresh Kumar Rudrahithlu, K. C. Shilpa |
How to Cite?
G. R. Meghana, Suresh Kumar Rudrahithlu, K. C. Shilpa, "Detection of Brain Cancer using Machine Learning Techniques a Review," SSRG International Journal of Computer Science and Engineering , vol. 9, no. 9, pp. 12-18, 2022. Crossref, https://doi.org/10.14445/23488387/IJCSE-V9I9P102
Abstract:
The segmentation and prediction of a brain tumour in medical image processing is a critical step. Early detection of brain tumours is critical for enhancing treatment options and boosting patient survival rates. Manual segmentation of brain tumours for cancer detection is challenging and time-consuming from enormous amounts of MRI data obtained in clinical practice. There is a demand for automated brain tumour detection. Classification and segmentation of brain tumours using MRI data is the focus of this work. Deep learning and machine learning approaches for automated segmentation and prediction have recently gained popularity since they provide cutting-edge results and are more suited to dealing with this challenge. MRI-based image data may also be processed efficiently and objectively using deep learning approaches. This paper surveys the thirty papers, including various machine and deep learning methods that can predict the brain tumor.
Keywords:
Brain tumor, MRI, Machine learning, Deep learning.
References:
[1] Lisa M. DeAngelis, “Brain Tumors,” The New England Journal of Medicine, vol. 344, no. 2, pp. 114-23, 2001.
[CrossRef] [Google Scholar] [Publisher Link]
[2] S. Deepak, and P.M. Ameer, “Brain Tumor Classification Using Deep CNN Features Via Transfer Learning,” Computers in Biology and Medicine, vol. 111, 2019.
[CrossRef] [Google Scholar] [Publisher Link]
[3] Eser Sert, Fatih Özyurt, and Akif Doğantekin, “A New Approach for Brain Tumor Diagnosis System: Single Image Super Resolution Based Maximum Fuzzy Entropy Segmentation and Convolutional Neural Network,” Medical Hypotheses, vol. 133, 2019.
[CrossRef] [Google Scholar] [Publisher Link]
[4] Sudipta Roy, and Samir K. Bandyopadhyay, “Detection and Quantification of Brain Tumor from MRI of Brain and It’s Symmetric Analysis,” International Journal of Information and Communication Technology Research, vol. 2, no. 6, pp. 477-483, 2012.
[Google Scholar] [Publisher Link]
[5] Sérgio Pereira et al., “Brain Tumor Segmentation Using Convolutional Neural Networks in MRI Images,” IEEE Transactions on Medical Imaging, vol. 35, no. 5, pp. 1240–1251, 2016.
[CrossRef] [Google Scholar] [Publisher Link]
[6] Arti Tiwari, Shilpa Srivastava, and Millie Pant, “Brain Tumor Segmentation and Classification From Magnetic Resonance Images: Review of Selected Methods From 2014 to 2019,” Pattern Recognition Letters, vol. 131, pp. 244–260, 2020.
[CrossRef] [Google Scholar] [Publisher Link]
[7] T. Gayathri, and K. Sundeep Kumar, “Alexnet – Adaptive Whale Optimization – Multi-Class Support Vector Machine Model for Brain Tumour Classification,” International Journal of Engineering Trends and Technology, vol. 70, no. 5, pp. 309-316, 2022.
[CrossRef] [Publisher Link]
[8] R. Stupp, and F. Roila, “Malignant Glioma: ESMO Clinical Recommendations for Diagnosis, Treatment and Follow-Up,” Annals of Oncology, vol. 20, pp. 126-128, 2009.
[CrossRef] [Google Scholar] [Publisher Link]
[9] Nilesh Bhaskarrao Bahadure et al., “Image Analysis for MRI Based Brain Tumor Detection and Feature Extraction Using Biologically Inspired BWT and SVM,” International Journal of Biomedical Imaging, vol. 2017, 2017.
[CrossRef] [Google Scholar] [Publisher Link]
[10] [Online]. Available: https://Figshare.Com/Articles/Dataset/Brain_Tumor_Dataset/1512427
[11] [Online]. Available: https://Brainweb.Bic.Mni.Mcgill.Ca/Cgi/Brainweb1
[12] [Online]. Available: https://Ieee-Dataport.Org/Competitions/Brats-Miccai-Brain-Tumor-Dataset
[13] [Online]. Available: https://www.Med.Upenn.Edu/Sbia/Brats2018/Data.html
[14] Wei Chen et al., “Automatic Brain Tumor Segmentation Based on Features of Separated Local Square,” Chinese Automation Congress (CAC), pp. 6489-6493, 2017.
[CrossRef] [Google Scholar] [Publisher Link]
[15] Tímea Fülöp et al., “Brain Tumor Segmentation From Multi-Spectral MRI Data Using Cascaded Ensemble Learning,” IEEE 15th International Conference of System of Systems Engineering (SOSE), pp. 531-536, 2020.
[CrossRef] [Google Scholar] [Publisher Link]
[16] Jindong Sune et al., “Segmentation of the Multimodal Brain Tumor Image Used the Multi-Pathway Architecture Method Based on 3D FCN,” Neurocomputing, vol. 423, pp. 34–45, 2021.
[CrossRef] [Google Scholar] [Publisher Link]
[17] Mostefa Ben naceur et al., “Fully Automatic Brain Tumor Segmentation with Deep Learning-Based Selective Attention Using Overlapping Patches and Multi-Class Weighted Cross-Entropy,” Medical Image Analysis, vol. 63, 2020.
[CrossRef] [Google Scholar] [Publisher Link]
[18] Mohamed A.Naser, and M. Jamal Deen, “Brain Tumor Segmentation and Grading of Lower-Grade Glioma Using Deep Learning in MRI Images,” Computers in Biology and Medicine, vol. 121, 2020.
[CrossRef] [Google Scholar] [Publisher Link]
[19] M.Monica Subashini et al., “A Non-Invasive Methodology for the Grade Identification of Astrocytoma Using Image Processing and Artificial Intelligence Techniques,” Expert System with Applications, vol.43, pp. 186-196, 2016.
[CrossRef] [Google Scholar] [Publisher Link]
[20] K. Mohana Priya, S. Kavitha, and B. Bharathi, “Brain Tumor Types and Grades Classification Based on Statistical Features Set Using Support Vector Machine,” 10th International Conference on Intelligent Systems and Control (ISCO), pp. 1-8, 2016.
[CrossRef] [Google Scholar] [Publisher Link]
[21] N. Nandha Gopal, and M. Karnan, “Diagnose Brain Tumor Through MRI Using Image Processing Clustering Algorithms Such as Fuzzy C Means Along with Intelligent Optimisation Techniques,” IEEE International Conference on Computational Intelligence and Computing Research, pp. 1-4, 2010.
[CrossRef] [Google Scholar] [Publisher Link]
[22] R. Surendiran et al., “Exploring the Cervical Cancer Prediction by Machine Learning and Deep Learning with Artificial Intelligence Approaches,” International Journal of Engineering Trends and Technology, vol. 70, no. 7, pp. 94-107, 2022.
[CrossRef] [Publisher Link]
[23] V.K. Sheela, and S. Suresh Babu, “Pre-Processing Technique for Brain Tumor Detection and Segmentation,” International Research Journal of Engineering and Technology (IRJET), vol. 2, no. 3, pp. 1208-1212, 2015.
[Google Scholar] [Publisher Link]
[24] Multimodal Brain Tumor Segmentation Challenge 2020, (CBICA)Center for Biomedical Image Computing & Analytics, 2020. [Online]. Available: https://www.med.upenn.edu/cbica/brats2020/data.html
[25] Limali Sahoo et al., “Detection and Classification of Brain Tumor Using Magnetic Resonance Images,” Advances in Electrical Control and Signal Systems, vol. 665, pp. 429–441, 2020.
[CrossRef] [Google Scholar] [Publisher Link]
[26] T. Kalaiselvi et al., “Deriving Tumor Detection Models Using Convolutional Neural Networks From MRI of Human Brain Scans,” International Journal of Information Technology, vol. 12, pp. 403-408, 2020.
[CrossRef] [Google Scholar] [Publisher Link]
[27] R. V. Kurup, V. Sowmya, and K. P. Soman, “Effect of Data Pre-Processing on Brain Tumor Classification Using Capsulenet,” ICICCT 2019 – System Reliability, Quality Control, Safety, Maintenance and Management, pp. 110-119, 2020.
[CrossRef] [Google Scholar] [Publisher Link]
[28] MesutToğaçar, Burhan Ergen, and Zafer Cömert, “BrainMRNet: Brain Tumor Detection Using Magnetic Resonance Images with a Novel Convolutional Neural Network Model,” Medical Hypotheses, vol. 134, 2020.
[CrossRef] [Google Scholar] [Publisher Link]
[29] Javaria Amin et al., “Brain Tumor Classification Based on DWT Fusion of MRI Sequences Using Convolutional Neural Network,” Pattern Recognition Letters, vol. 129, pp. 115–122, 2020.
[CrossRef] [Google Scholar] [Publisher Link]
[30] B. Anilkumar, and P. Rajesh Kumar, ‘Tumor Classification Using Block-Wise Fine Tuning and Transfer Learning of Deep Neural Network and KNN Classifier on MR Brain Images,” International Journal of Emerging Trends in Engineering Research, vol. 8, no. 2, pp. 574–583, 2020.
[CrossRef] [Google Scholar] [Publisher Link]
[31] Ischemic Stroke Lesion Segmentation Challenge, [Online]. Available: http://www.Isleschallenge.org/ISLES2016/
[32] Mallikarjun Mudda, R. Manjunath, and N. Krishnamurthy, “Brain Tumor Classification Using Enhanced Statistical Texture Features,” IETE Journal of Research, vol. 68, no. 5, pp. 1–12, 2020.
[CrossRef] [Google Scholar] [Publisher Link]
[33] OASIS (Open Access Series of Imaging Studies). [Online]. Available: https://www.Oasis-Brains.org/
[34] B. Anilkumar, and P. Rajesh Kumar, “Tumor Classification Using Block-Wise Fine Tuning and Transfer Learning of Deep Neural Network and KNN Classifier on MR Brain Images,” International Journal of Emerging Trends in Engineering Research, vol. 8, no. 2, pp. 574–583, 2020.
[CrossRef] [Google Scholar] [Publisher Link]
[35] Keith A. Johnson, and J. Alex Becker, The Whole Brain Atlas. [Online]. Available: http://www.Med.Harvard.Edu/AANLIB.
[36] Ranjeet Kaur, and Amit Doegar, “Localisation and Classification of Brain Tumor Using Machine Learning & Deep Learning Techniques,” The International Journal of Innovative Technology and Exploring Engineering, vol. 8, no. 9S, pp. 59–66, 2019.
[CrossRef] [Publisher Link]
[37] The Cancer Imaging Archive (TCIA). [Online]. Available: https://www.cancerimagingarchive.net/collections/
[38] Mohammed S. H. Al-Tamimi, and Ghazali Sulong, “Tumor Brain Detection Through MR Images: A Review of Literature,” Journal of Theoretical and Applied Information Technology, vol. 62, no. 2, pp. 387–403, 2014.
[Google Scholar] [Publisher Link]
[39] Adriano Pinto et al., “Random Decision Forests for Automatic Brain Tumor Segmentation on Multimodal MRI Images,” IEEE 4th Portuguese Meeting on Bioengineering (ENBENG), pp. 1-5, 2015.
[CrossRef] [Google Scholar] [Publisher Link]
[40] Mahmoud KhaledAbd-Ellah et al., “A Review on Brain Tumor Diagnosis From MRI Images: Practical Implications, Key Achievements, and Lessons Learned,” Magnetic Resonance Imaging, vol. 61, pp. 300– 318, 2019.
[CrossRef] [Google Scholar] [Publisher Link]
[41] Regina Pohle, and Klaus D. Toennies, “Segmentation of Medical Images Using Adaptive Region Growing,” Medical Imaging 2001: Image Processing, vol. 4322, 2001.
[CrossRef] [Google Scholar] [Publisher Link]
[42] Nameirakpam Dhanachandra, Khumanthem Manglem, and Yambem Jina Chanu, “Image Segmentation Using K -Means Clustering Algorithm and Subtractive Clustering Algorithm,” Procedia Computer Science, vol. 54, pp. 764–771, 2015.
[CrossRef] [Publisher Link]
[43] Shah Rukh Khan et al., “IOMT-Based Computational Approach for Detecting Brain Tumor,” Future Generation Computer Systems, vol. 109, pp. 360–367, 2020.
[CrossRef] [Google Scholar] [Publisher Link]
[44] Umit Ilhan, and Ahmet Ilhan, “Brain Tumor Segmentation Based on a New Threshold Approach,” Procedia Computer Science, vol. 120, pp. 580–587, 2017.
[CrossRef] [Google Scholar] [Publisher Link]
[45] Deimling A. Gliomas, Recent Results in Cancer Research, Berlin: Springer, vol. 171, 2009.
[Publisher Link]
[46] Muhammad Sharif et al., “An Integrated Design of Particle Swarm Optimization (PSO) with Fusion of Features for Detection of Brain Tumor,” Pattern Recognition Letters, vol. 129, pp. 150–157, 2020.
[CrossRef] [Google Scholar] [Publisher Link]
[47] OmidTarkhaneh, and Haifeng Shen, “An Adaptive Differential Evolution Algorithm to Optimal Multi-Level Thresholding for MRI Brain Image Segmentation,” Expert Systems with Applications, vol. 138, 2019.
[CrossRef] [Google Scholar] [Publisher Link]
[48] Itzel Aranguren et al., “Improving the Segmentation of Magnetic Resonance Brain Images Using the LSHADE Optimization Algorithm,” Biomed Signal Processing Control, vol. 64, 2021.
[CrossRef] [Google Scholar] [Publisher Link]
[49] A. Renugambal, and K. Selva Bhuvaneswari, “Image Segmentation of Brain MR Images Using Otsu’s Based Hybrid WCMFO Algorithm,” Computers, Materials and Continua, vol. 64, no. 2, pp. 681–700, 2020.
[CrossRef] [Google Scholar] [Publisher Link]
[50] Mahmoud KhaledAbd-Ellah et al., “A Review on Brain Tumor Diagnosis From MRI Images: Practical Implications, Key Achievements, and Lessons Learned,” Magnetic Resonance Imaging, vol. 61, pp. 300– 318, 2019.
[CrossRef] [Google Scholar] [Publisher Link]
[51] Atiq Islam et al., “Multifractal Texture Estimation for Detection and Segmentation of Brain Tumors,” IEEE Tranaction on Biomedical Engineering, vol 60, no.11, pp. 3204-3215, 2013.
[CrossRef] [Google Scholar] [Publisher Link]
[52] Khan M Iftekharuddin et al., “Fractual Based Brain Tumor Detection in Multimodal MRI,” Applied Mathematics and Computation, vol. 207, no. 1, pp. 23-41, 2009.
[CrossRef] [Google Scholar] [Publisher Link]
[53] Sneha Khare, Neelesh Gupta, and Vibhanshu Srivastava, “Optimization Technique, Curve Fitting and Machine Learning Used to Detect Brain Tumor in MRI,” IEEE International Conference on Computer Communication and Systems, pp. 254-259, 2014.
[CrossRef] [Google Scholar] [Publisher Link]
[54] Francisco Javier Díaz-Pernas et al., “A Deep Learning Approach for Brain Tumor Classification and Segmentation Using a Multiscale Convolutional Neural Network,” Healthcare, Multidisciplinary Digital Publishing Institute, vol. 9. no. 2, p. 153, 2021.
[CrossRef] [Google Scholar] [Publisher Link]
[55] Emrah Irmak, “Multi-Classification of Brain Tumor MRI Images Using Deep Convolutional Neural Network with Fully Optimised Framework,” Iranian Journal of Science and Technology, Transactions of Electrical Engineering, vol. 45, pp. 1015–1036, 2021.
[CrossRef] [Google Scholar] [Publisher Link]
[56] Wu Deng et al., “Deep Learning-Based HCNN and CRF-RRNN Model for Brain Tumor Segmentation,” IEEE Access, vol. 8, pp. 26665–26675, 2020.
[CrossRef] [Google Scholar] [Publisher Link]
[57] Chirodip Lodh Choudhury et al., “Brain Tumor Detection and Classification Using Convolutional Neural Network and Deep Neural Network,” International Conference on Computer Science, Engineering and Applications (ICCSEA), IEEE, 2020.
[CrossRef] [Google Scholar] [Publisher Link]
[58] A. Keerthana et al., “Brain Tumour Detection Using Machine Learning Algorithm,” Journal of Physics: Conference Series, vol. 1937, no. 1, 2021.
[CrossRef] [Google Scholar] [Publisher Link]
[59] [Online]. Available: http://www.internationaljournalssrg.org/ssrg-journals.html