Cancer Prediction Using Mining Gene Expression Data
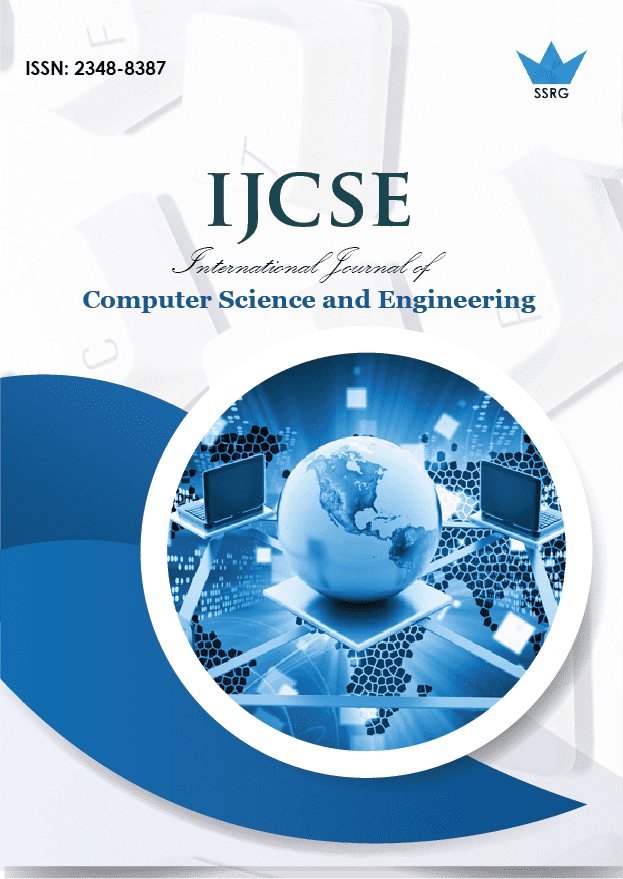
International Journal of Computer Science and Engineering |
© 2015 by SSRG - IJCSE Journal |
Volume 2 Issue 2 |
Year of Publication : 2015 |
Authors : Mr. S.Sivakumar, D.Viji |
How to Cite?
Mr. S.Sivakumar, D.Viji, "Cancer Prediction Using Mining Gene Expression Data," SSRG International Journal of Computer Science and Engineering , vol. 2, no. 2, pp. 22-28, 2015. Crossref, https://doi.org/10.14445/23488387/IJCSE-V2I2P105
Abstract:
Cancer is a major cause of all natural mortalities and morbidities throughout the world.Pointed out the exact tumour types provides an optimized solution for the better treatment and toxicity minimization due to medicines on the patients. To get a clear picture on the insight of a problem, a clear cancer classification analysis system needs to be pictured followed by a systematic approach to analyse global gene expression which provides an optimized solution for the identified problem area. Molecular diagnostics provides a promising option of systematic human cancer classification, but these tests are not widely applied because characteristic molecular markers for most solid tumor save yet to be identified. Recently, DNA microarray-based tumor gene expression profiles have been used for cancer diagnosis. Existing system focussed in ranging from old nearest neighbour analysis to support vector machine manipulation for the learning portion of the classification model. We don’t have a clear picture of supervised classifier (Supervised Multi Attribute Clustering Algorithm) which can manage knowledge attributes coming two different knowledge streams. Our proposed system takes the input from multiple source, create an ontological store, cluster the data with attribute match association rule and followed by classification with the knowledge acquired
Keywords:
DNA Microarray, Gene expression,Ontology, supervised multi attribute clustering.
References:
[1] Alfredo Benso, Stefano Di Carlo, and Gianfranco Politano‖ A cDNA Microarray Gene Expression Data Classifier for Clinical Diagnostics Based on Graph Theory‖ IEEE/ACM Transactions On Computational Biology And Bioinformatics, Vol. 8, No. 3, May/June 2011
[2] Anirban Mukhopadhyay, Senior Member, IEEE, Ujjwal Maulik, Senior Member, IEEE, and Sanghamitra Bandyopadhyay, Senior Member, IEEE, ―An Interactive Approach to Multiobjective Clustering of Gene Expression Patterns‖ IEEE Transactions On Biomedical Engineering, Vol. 60, No. 1, February 2013
[3] Cosmin Lazar, Jonatan Taminau, Stijn Meganck, David Steenhoff, Alain Coletta, David Y. Weiss Solı´s, Colin Molter, Robin Duque, Hugues Bersini, and Ann Nowe ―GENESHIFT: A Nonparametric Approach for Integrating Microarray Gene Expression Data Based on the Inner Product as a Distance Measure between the Distributions of Genes‖ IEEE/Acm Transactions On Computational Biology And Bioinformatics, Vol. 10, No. 2, March/April 2013
[4] Daxin Jiang, Chun Tang, and Aidong Zhang,‖ Cluster Analysis for Gene Expression Data: A Survey‖ IEEE Transactions On Knowledge And Data Engineering, Vol. 16, No. 11, November 2004
[5] Elrasheid A.H. Kheirelseid, Nicola Miller, Kah Hoong Chang, Mary Nugent, Michael J. Kerin,‖ Clinical applications of gene expression in colorectal cancer‖, Submitted Jan 24, 2013. Accepted for publication Feb 27, 2013. doi: 10.3978/j.issn.2078-6891.2013.010
[6] KM Fedorka1, WE Winterhalter1 and TA Mousseau2,‖ The evolutionary genetics of sexual size dimorphism in the cricket Allonemobius socius‖ Heredity (2007) 99, 218–223 & 2007 Nature Publishing Group All rights reserved 0018-067X/07
[7] Kristopher L. Patton, David J. John, James L. Norris, Daniel R. Lewis, and Gloria K. Muday ―Hierarchical Probabilistic Interaction Modeling for Multiple Gene Expression Replicates‖ IEEE/ACM Transactions On Computational Biology And Bioinformatics, Vol. 11, No. 2, March/April 2014
[8] Lipo Wang, Feng Chu, and Wei Xie ―Accurate Cancer Classification Using Expressions of Very Few Genes‖ IEEE/ACM Transactions On Computational Biology And Bioinformatics, Vol. 4, No. 1, February -March 2007
[9] Robert S. H. Istepanian , Senior Member, IEEE, Ala Sungoor, and Jean-Christophe Nebel, Senior Member, IEEE ‖ Comparative Analysis of Genomic Signal Processing for Microarray Data Clustering ‖ IEEE Transactions On Nanobioscience, Vol. 10, No. 4, December 2011
[10] Ujjwal Maulik∗, Senior Member, IEEE, Anirban Mukhopadhyay∗, Senior Member, IEEE, and Debasis Chakraborty ―Gene-Expression-Based Cancer Subtypes Prediction Through Feature Selection and Transductive SVM‖ Ieee Transactions On Biomedical Engineering, Vol. 60, No. 4, April 2013
vv [11] S. Dudoit, J. Fridlyand, and T. P. Speed, ―Comparison of discrimination methods for the classification of tumors using gene expression data,‖ J. Amer. Statist. Assoc., vol. 97, no. 457, pp. 77–87, Mar. 2002.
[12] G.-M. Elizabeth and P. Giovanni, (2004, Dec.). ―Clustering and classification methods for gene expression data analysis.‖ Johns Hopkins Univ., Dept. of Biostatist. Working Papers. Working Paper 70.
[13] Shaurya Jauhari and S.A.M. Rizvi Mining Gene Expression Data Focusing Cancer Therapeutics: A Digest IEEE/ACM Transactions On Computational Biology And Bioinformatics, Vol. 11, No. 3, May/June 2014
[14] A. Ben-Dor, N. Friedman, and Z. Yakhini, ―Class Discovery in Gene Expression Data,‖ Proc. Fifth Ann. Int‘l Conf. ComputationalMolecular Biology (RECOMB 2001), pp. 31-38, 2001.
[15] A. Ben-Dor, R. Shamir, and Z. Yakhini, ―Clustering Gene Expression Patterns,‖ J. Computational Biology, vol. 6, nos. 3/4, pp. 281-297, 1999.
[16] Harleen Kaur and Siri Krishan Wasan, Empirical Study on Applications of Data Mining Techniques in Healthcare, Journal of Computer Science 2 (2): 194- 200, 2006ISSN 1549-3636.
[17] N. Pasquier, C. Pasquier, L. Brisson, and M. Collard, (2008). ―Mining gene expression data using domain knowledge,‖ Int. J. Softw. Informat, vol. 2, no. 2, pp. 215–231, [Online] Available: http://www.ijsi.org/1673- 7288/2/215.pdf.
[18] Y. Su, T. M. Murali, V. Pavlovic, M. Schaffer, and S. Kasif, (2003) ―RankGene: Identification of diagnostic genes based on expression data,‖ Bioinformatics, vol. 19, no. 12, pp. 1578–1579, [Online] Avaialble: http://bioinformatics.oxfordjournals.org/content/19/12/1 578.full.pdf.
[19] K. M Williams, ―Statistical Methods for analysing microarray data: Detection of differentially expressed genes‖ Inst. Signal Process.,Tampere Univ. Technol. Tampere, Finland, Dep. Biology, Univ. York, York, U.K., 2004.
[20] B. Collard, ―An ontology driven data mining process‖ Inst. TELECOM, TELECOM Bretagne, CNRS FRE 3167 LAB-STICC,Technopole Brest-Iroise, France & Univ. Nice Sophia Antipolis, France, 2008.
[21] T. Kohonen, Self-Organization and Associative Memory. Berlin: Spring-Verlag, 1984.