The Impact, Advancements and Applications of Generative AI
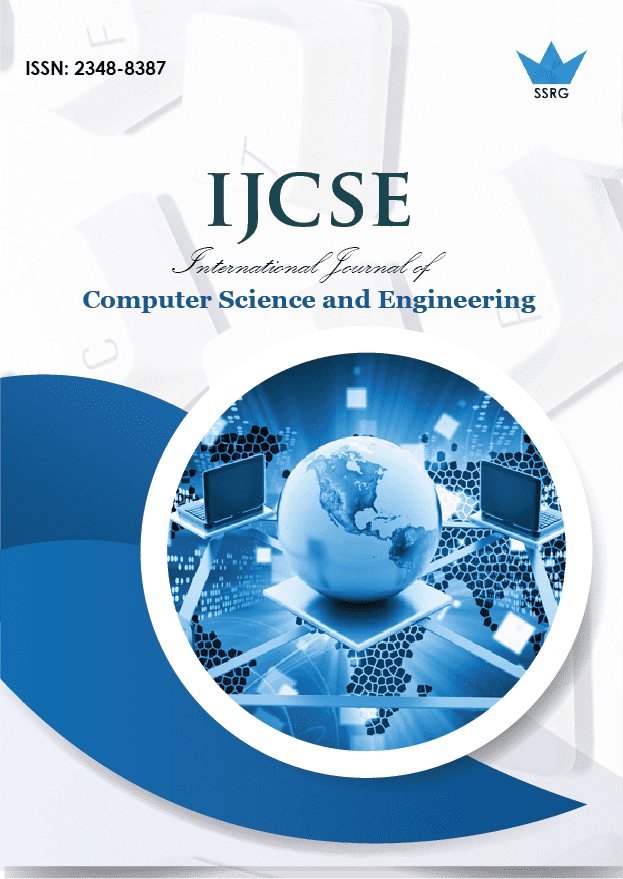
International Journal of Computer Science and Engineering |
© 2023 by SSRG - IJCSE Journal |
Volume 10 Issue 6 |
Year of Publication : 2023 |
Authors : Balagopal Ramdurai, Prasanna Adhithya |
How to Cite?
Balagopal Ramdurai, Prasanna Adhithya, "The Impact, Advancements and Applications of Generative AI," SSRG International Journal of Computer Science and Engineering , vol. 10, no. 6, pp. 1-8, 2023. Crossref, https://doi.org/10.14445/23488387/IJCSE-V10I6P101
Abstract:
Generative AI is a subfield of artificial intelligence ecosystem based on developing systems that can generate creative outputs, such as music, images, text content, and more. By harnessing the power of deep learning techniques, specifically generative models, these systems have the ability to create content that matches human-generated creations autonomously. The key to generative AI lies in its ability to learn from datasets and patterns and generate new content that exhibits similar traits. Generative models, such as generative adversarial networks (GANs) and variational autoencoders (VAEs), form the basis of generative AI. GANs consist of two components : (1) a generator and (2) a discriminator network engaged in a competitive & consistent process of generating and evaluating content. VAEs, employ an encoder-decoder architecture to learn and generate new output. This is research on Generative AI and its impact cutting across all verticals.
Keywords:
ChatGPT, Generative AI, Artificial intelligence, Deep learning, Generative models, Generative adversarial networks (GANs), Variational autoencoders (VAEs).
References:
[1] Diederik P. Kingma, and Max Welling, “Auto-Encoding Variational Bayes,” Machine Learning, 2013.
[CrossRef] [Google Scholar] [Publisher Link]
[2] Ian J. Goodfellow et al., “Generative Adversarial Networks,” Machine Learning, 2014.
[CrossRef] [Publisher Link]
[3] Y. Li et al., “Artificial Intelligence in Fashion: Generative Models for Fashion Design,” IEEE Access, 2019.
[4] David Newton, “Generative Deep Learning in Architectural Design,” Technology| Architecture+ Design, vol. 3, no. 2, pp. 176-189, 2019.
[CrossRef] [Google Scholar] [Publisher Link]
[5] Samer BuHamdan, Aladdin Alwisy, and Ahmed Bouferguene, “Generative Systems in the Architecture, Engineering and Construction Industry: A Systematic Review and Analysis,” International Journal of Architectural Computing, vol. 19, no. 3, pp. 226-249, 2021.
[CrossRef] [Google Scholar] [Publisher Link]
[6] Giuseppe Amato et al., “AI in the Media and Creative Industries,” Artificial Intelligence, 2019.
[CrossRef] [Google Scholar] [Publisher Link]
[7] Diane M. Korngiebel, and Sean D. Mooney, “Considering the Possibilities and Pitfalls of Generative Pre-trained Transformer 3 (GPT-3) in Healthcare Delivery,” NPJ Digital Medicine, vol. 4, 2021.
[CrossRef] [Google Scholar] [Publisher Link]
[8] Adam Bohr, and Kaveh Memarzadeh, “Chapter 2 – The Rise of Artificial Intelligence in Healthcare Applications,” Artificial Intelligence in Healthcare, pp. 25-60, 2020.
[CrossRef] [Google Scholar] [Publisher Link]
[9] J. Smith, A. Johnson, and M. Davis, “Generative Adversarial Networks: A Novel Approach for Personalized Advertising,” Journal: Journal of Marketing Analytics, 2020.
[10] R. Johnson, E. Smith, and M. Anderson, “Generative AI for Financial Forecasting: Enhancing Accuracy and Decision-Making,” Journal: Journal of Financial Analytics, 2021.
[11] A. Wilson, L. Roberts, and M. Davis, “Deep Art: Exploring the Intersection of Deep Learning and Artistic Creativity,” Journal: Journal of Artificial Intelligence in Creative Arts, 2022.
[12] R. Thompson, S. Johnson, and L. Brown, “RunwayML: An Empowering Tool for Artists and Designers in the Age of AI,” Journal: International Journal of Creative Computing, 2021.
[13] H. Chen et al., “NVIDIA GauGAN: Bridging the Gap between Artists and AI in Visual Content Generation,” Journal: ACM Transactions on Graphics, 2019.
[14] Min-Yuh Day, Jian-Ting Lin, and Yuan-Chih Chen, “Artificial Intelligence for Conversational Robo-Advisor,” 2018 IEEE/ACM International Conference on Advances in Social Networks Analysis and Mining (ASONAM), 2018.
[CrossRef] [Google Scholar] [Publisher Link]
[15] Deep Ganguli et al., “Predictability and Surprise in Large Generative Models,” 2022 ACM Conference on Fairness, Accountability, and Transparency, pp. 1747–1764, 2022.
[CrossRef] [Google Scholar] [Publisher Link]
[16] Philipp Hacker, Andreas Engel, and Marco Mauer, “Regulating ChatGPT and other Large Generative AI Models,” Computers and Society, 2023.
[CrossRef] [Google Scholar] [Publisher Link]
[17] Tian Jipeng, P. Suma, and T.C. Manjunath, “AI, ML and The Eye Disease Detection,” SSRG International Journal of Computer Science and Engineering, vol. 7, no. 4, pp. 1-3, 2020.
[CrossRef] [Publisher Link]
[18] Ian Goodfellow et al., “Generative Adversarial Networks,” Communication of the ACM, vol. 63, no. 11, pp. 139–144, 2020.
[CrossRef] [Google Scholar] [Publisher Link]
[19] Kunfeng Wang et al., “Generative Adversarial Networks: Introduction and Outlook,” IEEE/CAA Journal of Automatica Sinica, vol. 4, no. 4, pp. 588-598, 2017. [CrossRef] [Google Scholar] [Publisher Link]
[20] Isabela Albuquerque et al., “Multi-objective Training of Generative Adversarial Networks with Multiple Discriminators,” Proceedings of the 36th International Conference on Machine Learning, pp. 202-211, 2019.
[Google Scholar] [Publisher Link]
[21] Khaled Elbehiery, and Hussam Elbehiery, “Millennial National Security’s Cornerstones 5G, Cloud Technology, and Artificial Intelligence,” SSRG International Journal of Electronics and Communication Engineering, vol. 6, no. 8, pp. 44-54, 2019.
[CrossRef] [Publisher Link]
[22] Shiva Mayahi, and Marko Vidrih, “The Impact of Generative AI on the Future of Visual Content Marketing,” Human-Computer Interaction, 2022.
[CrossRef] [Google Scholar] [Publisher Link]
[23] Antonia Creswell et al., “Generative Adversarial Networks: An Overview,” IEEE Signal Processing Magazine, vol. 35, no. 1, pp. 53-65, 2018.
[CrossRef] [Google Scholar] [Publisher Link]
[24] Divya Saxena, and Jiannong Cao et al., “Generative Adversarial Networks (GANs): Challenges, Solutions, and Future Directions,” ACM Computing Surveys, vol. 54, no. 3, pp. 1-42, 2021.
[CrossRef] [Google Scholar] [Publisher Link]