Bias in AI: A Comprehensive Examination of Factors and Improvement Strategies
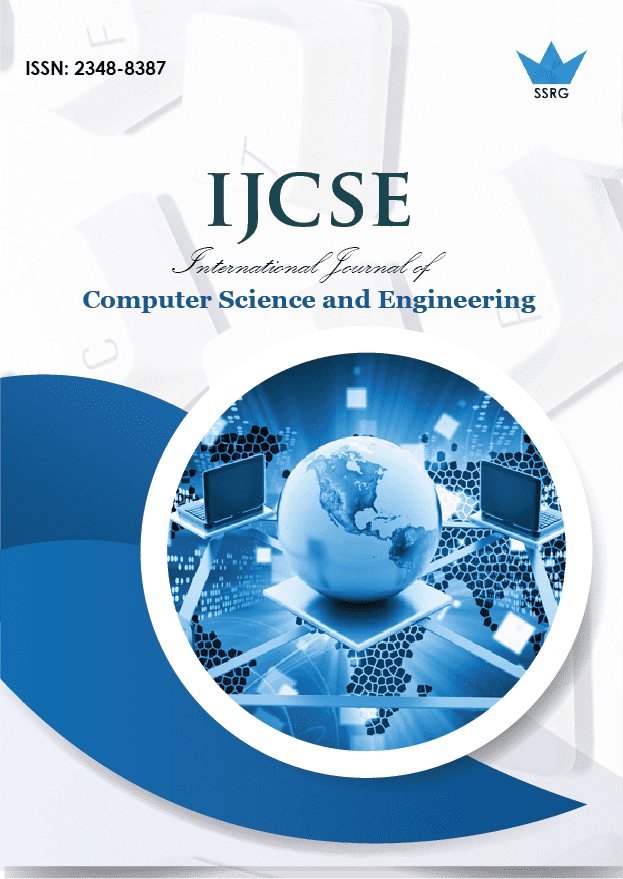
International Journal of Computer Science and Engineering |
© 2023 by SSRG - IJCSE Journal |
Volume 10 Issue 6 |
Year of Publication : 2023 |
Authors : Amey Bhandari |
How to Cite?
Amey Bhandari, "Bias in AI: A Comprehensive Examination of Factors and Improvement Strategies," SSRG International Journal of Computer Science and Engineering , vol. 10, no. 6, pp. 9-14, 2023. Crossref, https://doi.org/10.14445/23488387/IJCSE-V10I6P102
Abstract:
Artificial intelligence is becoming extremely popular in our lives, being used in every sector, from job applications to medical diagnoses. AI is often biased due to various factors, ranging from biased training data to a lack of diversity and the designing and modeling team. Bias in AI is this research paper’s focus, which starts by discussing AI development and a basic understanding of how AI models work. Later, bias in AI and its reasons are discussed with examples, along with a comparison of bias in different AI models. Image generation AI models such as Stable Diffusion and DALL-E 2, along with text generation AIs such as ChatGPT, are analyzed. Bias in AI in different respects, such as Gender, Religion, and Race, has been explored in detail. Towards the end, steps that have been taken to mitigate bias have been discussed.
Keywords:
Artificial Intelligence, Bias, Computational intelligence sensitive features, Training data.
References:
[1] Abubakar Abid, Maheen Farooqi, and James Zou, “Large Language Models Associate Muslims with Violence,” Nature Machine Intelligence, vol. 3, pp. 461-463, 2021. [CrossRef] [Google Scholar] [Publisher Link]
[2] Marvin Van Bekkum, and Frederik Zuiderveen Borgesius, “Using Sensitive Data to Prevent Discrimination by Artificial Intelligence: Does the GDPR Need a New Exception?,” Computer Law and Security Review, vol. 48, p. 105770, 2023.
[CrossRef] [Google Scholar] [Publisher Link]
[3] Abeba Birhane, Vinay Uday Prabhu, and Emmanuel Kahembwe, “Multimodal Datasets: Misogyny, Pornography, and Malignant Stereotypes,” Computers and Society, 2021.
[CrossRef] [Google Scholar] [Publisher Link]
[4] Sumon Biswas, and Hridesh Rajan, “Do the Machine Learning Models on a Crowd Sourced Platform Exhibit Bias? An Empirical Study on Model Fairness,” Proceedings of the 28th ACM Joint Meeting on European Software Engineering Conference and Symposium on the Foundations of Software Engineering, pp. 642-653, 2020.
[CrossRef] [Google Scholar] [Publisher Link]
[5] Tom Brown et al., “Language Models are Few-Shot Learners,” Advances in Neural Information Processing Systems, 2020.
[Google Scholar] [Publisher Link]
[6] Curious about what we do? – Common Crawl. [Online]. Available: https://commoncrawl.org/big-picture/what-we-do/
[7] DALL·E 2 Pre-training Mitigations, 2022. [Online]. Available: https://openai.com/research/dall-e-2-pre-training-mitigations
[8] Chris DeBrusk, “The Risk of Machine Learning Bias (And How to Prevent it),” Risk Journal, 2018.
[Google Scholar] [Publisher Link]
[9] Nassim Dehouche, and Kullathida Dehouche, “What’s in a Text-to-Image Prompt? The Potential of Stable Diffusion in Visual Arts Education,” Heliyon, vol. 9, no. 6, 2023.
[CrossRef] [Publisher Link]
[10] Matt Brems, ELI5 (Explain Like I'm 5) CLIP: Beginner's Guide to the CLIP Model, 2021. [Online]. Available:
https://blog.roboflow.com/clip-model-eli5-beginner-guide/
[11] Xavier Ferrer et al., “Bias and Discrimination in AI: A Cross-Disciplinary Perspective,” IEEE Technology and Society Magazine, vol. 40, no. 2, pp. 72-80, 2021. [CrossRef] [Google Scholar] [Publisher Link]
[12] Ayanna Howard, Cha Zhang, and Eric Horvitz, “Addressing Bias in Machine Learning Algorithms: A Pilot Study on Emotion Recognition for Intelligent Systems,” IEEE Workshop on Advanced Robotics and its Social Impacts, 2017.
[CrossRef] [Google Scholar] [Publisher Link]
[13] Faisal Kamiran, Toon Calders, and Mykola Pechenizkiy, “Techniques for Discrimination-Free Predictive Model,” Discrimination and Privacy in the Information Society, pp. 223-239, 2013.
[CrossRef] [Google Scholar] [Publisher Link]
[14] Alexandre Sasha Luccioni et al., Stable Bias: Analyzing Societal Representations in Diffusion Models, 2023.
[Publisher Link]
[15] Vijit Malik et al., “Socially Aware Bias Measurements for Hindi Language Representations,” Computation and Language, 2021.
[CrossRef] [Google Scholar] [Publisher Link]
[16] Elman Mansimov et al., “Generating Images from Captions with Attention,” Machine Learning, 2015.
[CrossRef] [Google Scholar] [Publisher Link]
[17] Eirini Ntoutsi et al., “Bias in Data-driven Artificial Intelligence Systems—An Introductory Survey,” Data Mining and Knowledge Discovery, vol. 10, no. 3, 2020. [CrossRef] [Google Scholar] [Publisher Link]
[18] Ravi B. Parikh, Stephen Teeple, and Amol S. Navathe, “Addressing Bias in Artificial Intelligence in Health Care,” JAMA Network, vol. 322, no. 24, pp. 2377-2378, 2019.
[CrossRef] [Google Scholar] [Publisher Link]
[19] Reducing bias and improving safety in DALL·E 2, OpenAI, 2022. [Online]. Available: https://openai.com/blog/reducing-bias-and-improving-safety-in-dall-e-2
[20] Sigal Samuel, A New AI Draws Delightful and Not-so-Delightful Images, 2022. [Online]. Available: https://www.vox.com/future-perfect/23023538/ai-dalle-2-openai-bias-gpt-3-incentives
[21] The Text-to-image Revolution, Explained, 2022. [Online]. Available: https://youtu.be/SVcsDDABEkM
[22] C. Vaccari, and A. Chadwick, Dalle-2-Preview/System-Card.md, 2022. [Online]. Available: https://github.com/openai/dalle-2-preview/blob/main/system-card.md#bias-and-representation
[23] Brad Dwyer, OpenAI's CLIP is the Most Important Advancement in Computer Vision this Year, 2021. [Online]. Available:
https://blog.roboflow.com/openai-clip/
[24] H. Yu et al., “Building Ethics into Artificial Intelligence,” arXiv Preprint arxiv: 1812.02953, 2018.
[Google Scholar]
[25] Indre Zliobaite, and Bart Custers, “Using Sensitive Personal Data may be Necessary for Avoiding Discrimination in Data-driven Decision Models,” Artificial Intelligence and Law, vol. 24, pp. 183-201, 2016.
[CrossRef] [Google Scholar] [Publisher Link]