Smart Recommendation for Unanimous People
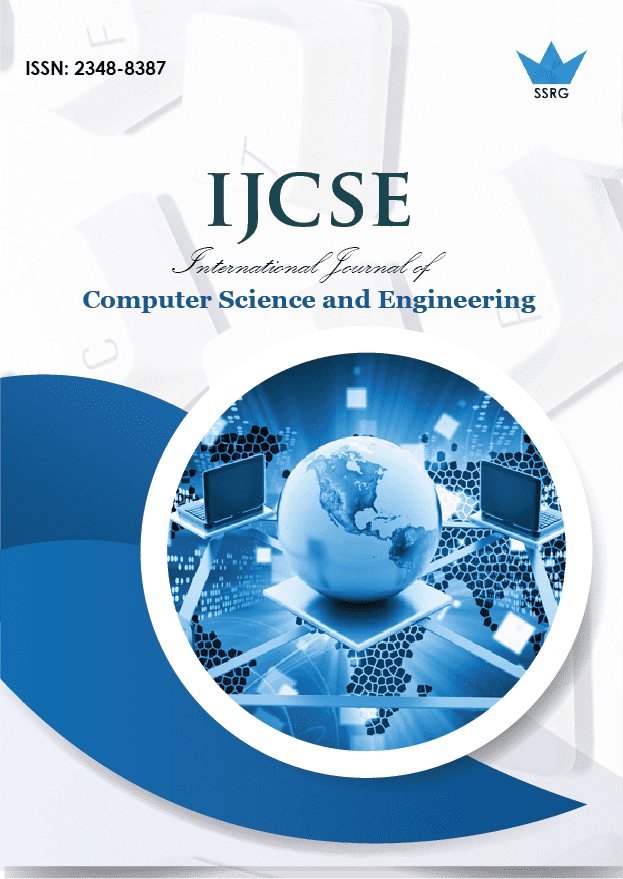
International Journal of Computer Science and Engineering |
© 2023 by SSRG - IJCSE Journal |
Volume 10 Issue 7 |
Year of Publication : 2023 |
Authors : G. Abhilash, M. Karthik, S. Preetha |
How to Cite?
G. Abhilash, M. Karthik, S. Preetha, "Smart Recommendation for Unanimous People," SSRG International Journal of Computer Science and Engineering , vol. 10, no. 7, pp. 1-5, 2023. Crossref, https://doi.org/10.14445/23488387/IJCSE-V10I7P101
Abstract:
Creativity has become one of the optimal technologies to solve human life problems, and technology isused for facilitating human needs. People always seek and be more comfortable based on a similar mindset. Which in return helps them to build new ideas and thoughts. With the popularity of social networks and social media, many users like to share their reviews, ratings, experiences, and images. The factors that are most considered by social media platforms, like influence, search content and interest based on friends, bring connectivity for smart recommendation systems to establish the relation between the users with the help of the data collected from the users. Social factors like interpersonal interest similarity, interpersonal influence, and personal interest these factors are taken into consideration before recommending friends to users. This smart recommendation model, which we have used, is based on Latent Dirichlet Allocation (LDA) algorithm. The category of personal interest will help the users to club together and can recommend people to the user based on individualities. The interpersonal influence helps users to connect based on theirinterest towards learning and innovation; they can connect and discuss regarding their common interests.
Keywords:
Interpersonal interest similarity, Interpersonal influence, Smart recommendation, Personal interest.
References:
[1] [Online]. Available: https://ai.facebook.com/blog/powered-by-ai-instagrams-explore-recommender-system/
[2] Xiwang Yang, Harald Steck, and Yong Liu, “Circle-Based Recommendation in Online Social Networks,” Proceedings of the 18th ACM SIGKDD International Conference on Knowledge Discovery and Data Mining, pp. 1267-1275, 2012.
[CrossRef] [Google Scholar] [Publisher Link]
[3] Weimin Li et al., “Social Recommendation based on Trust and Influence in SNS Environments,” Multimedia Tools and Applications, vol. 76, pp. 11585-11602, 2017.
[CrossRef] [Google Scholar] [Publisher Link]
[4] Fangyuan Zhao et al., “Latent Dirichlet Allocation Model Training with Differential Privacy,” IEEE Transactions on Information Forensics and Security, vol. 16, pp. 1290-1305, 2021.
[CrossRef] [Google Scholar] [Publisher Link]
[5] Xiang Wang, “Exploiting Cross-Channel Information for Personalized Recommendation,” National University of Singapore, 2019.
[Google Scholar] [Publisher Link]
[6] Michael Jahrer, Andreas Töscher, and Robert Legenstein, “Combining predictions for Accurate Recommender Systems,” Proceedings of the 16th ACM SIGKDD International Conference on Knowledge Discovery and Data Mining, pp. 693–702, 2010.
[CrossRef] [Google Scholar] [Publisher Link]
[7] Xiwang Yang, Yang Guo, and Yong Liu, “Bayesian-Inference Based Recommendation in Online Social Networks,” IEEE Transactions on Parallel and Distributed Systems, Shanghai, China, vol. 24, no. 4, pp. 642-651, 2013.
[CrossRef] [Google Scholar] [Publisher Link]
[8] Meng Jiang et al., “Social Contextual Recommendation,” Proceedings of the 21st ACM International Conference on Information and Knowledge Management, pp. 45-54, 2012.
[CrossRef] [Google Scholar] [Publisher Link]
[9] David M. Blei, Andrew Y. Ng, and Michael I. Jordan, “Latent Dirichlet Allocation,” Journal of Machine Learning Research, vol. 3, pp. 993-1022, 2003.
[Google Scholar] [Publisher Link]
[10] Kratika Sharma, and Nitin Jaiswal, "Human Capital Management: An Emerging Human Resource Management Practice," SSRG International Journal of Economics and Management Studies, vol. 5, no. 3, pp. 36-41, 2018.
[Google Scholar] [Publisher Link]
[11] Mohsen Jamali, and Martin Ester, “A Matrix Factorization Technique with Trust Propagation for Recommendation in Social Networks,” Proc. ACM Conf. RecSys, Barcelona, Spain, pp. 135-142, 2010.
[CrossRef] [Google Scholar] [Publisher Link]
[12] Andriy Mnih, and Russ R. Salakhutdinov, “Probabilistic Matrix Factorization,” NeurIPS Proceedings, 2008.
[Google Scholar] [Publisher Link]
[13] G. Adomavicius, and A. Tuzhilin, “Toward the Next Generation of Recommender Systems: A Survey of the State-of-the-Art and Possible Extensions,” IEEE Transactions on Knowledge and Data Engineering, vol. 17, no. 6, pp. 734-749, 2005.
[CrossRef] [Google Scholar] [Publisher Link]