Renewable Wind Energy using IoT-Based Smart Appliances
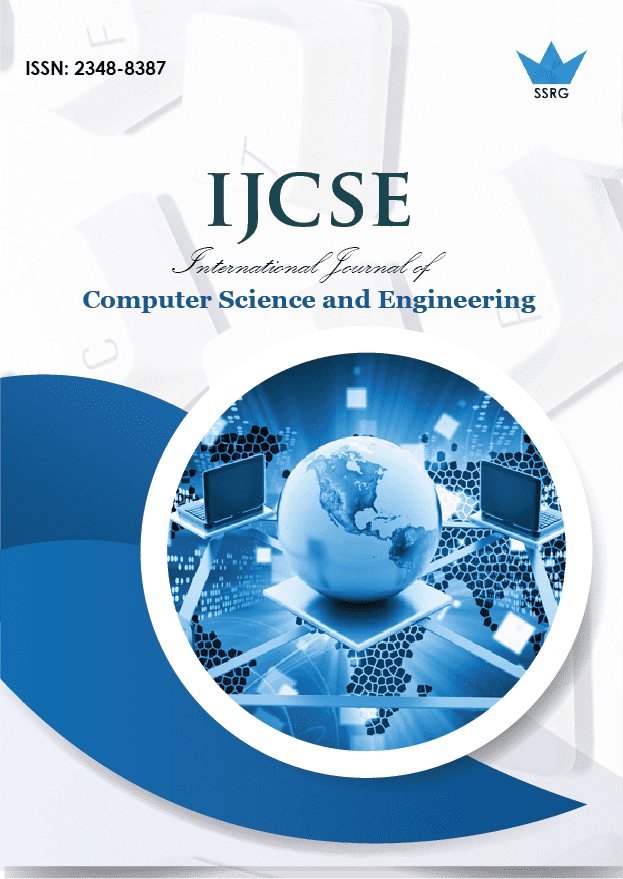
International Journal of Computer Science and Engineering |
© 2022 by SSRG - IJCSE Journal |
Volume 9 Issue 11 |
Year of Publication : 2022 |
Authors : R. Surendiran, M.Thangamani |
How to Cite?
R. Surendiran, M.Thangamani, "Renewable Wind Energy using IoT-Based Smart Appliances," SSRG International Journal of Computer Science and Engineering , vol. 9, no. 11, pp. 1-8, 2022. Crossref, https://doi.org/10.14445/23488387/IJCSE-V9I11P101
Abstract:
Electricity is one of the key factors in human life to survive on the earth. Major of our work requires electricity so it is important to power saving. It is difficult to get power from the rain in the solar panel. So, we proposed an energy-efficient IoT-based smart appliance, renewable wind energy. The term "distributed energy resource system" refers to the fusion of one or more energy sources in a power method. In this research, smart appliances are used to save energy while considering the aforementioned concerns. A detailed comparative results analysis of some Deep Learning (DL) based Smart Appliances in terms of precision, specificity, accuracy and recall. The Convolution Neural Network (CNN) based Smart Appliances method outperformed. The suggested framework is compared with existing methods such as HGPDO, CNN, and ANN in terms of Accuracy, precision, Recall, and Specificity. According to experimental findings, the proposed technique extends smart appliances by 50%, 9.756%, and 26.829%, compared with HGPDO, CNN, and ANN Methods.
Keywords:
Convolution Neural Network, Deep Learning.
References:
[1] Shanshan Zhao, Shancang Li, and Yufeng Yao, “Blockchain Enabled Industrial Internet of Things Technology,” IEEE Transactions on Computational Social Systems, vol. 6, no. 6, pp.1442-1453, 2019.
[CrossRef] [Google Scholar] [Publisher Link]
[2] Can Azkan et al., “A Taxonomy for Data-Driven Services in Manufacturing Industries,” Twenty-Fourth Pacific Asia Conference on Information Systems, pp. 1-147, 2020.
[Google Scholar] [Publisher Link]
[3] Hongming Yang et al., “Distributionally Robust Optimal Dispatch in the Power System with High Penetration of Wind Power Based on Net Load Fluctuation Data,” Applied Energy, vol. 313, 2022.
[CrossRef] [Google Scholar] [Publisher Link]
[4] Niklas Requate, Tobias Meyer, and Rene Hofmann, “From Wind Conditions to Operational Strategy: Optimal Planning of Wind Turbine Damage Progression over its Lifetime,” Wind Energy Science Discussions, pp.1-51, 2022.
[CrossRef] [Google Scholar] [Publisher Link]
[5] Filippo Trevisi et al., “Flight Trajectory Optimization of Fly-Gen Airborne Wind Energy Systems through a Harmonic Balance Method,” Wind Energy Science, vol. 7, no. 5, pp. 2039-2058, 2022.
[CrossRef] [Google Scholar] [Publisher Link]
[6] Ghulam Murtaza Sahito et al., “Design of PI Controller for Wind Energy Conversion System Using MATLAB/Simulink,” International Journal of Electrical Engineering & Emerging Technology, vol. 5, no. 2, pp. 6-10, 2022.
[Google Scholar] [Publisher Link]
[7] Tariq Al-Abri et al., “Review on Energy Application Using Blockchain Technology with an Introductions in the Pricing Infrastructure,” IEEE Access, vol. 10, pp. 80119-80137, 2022.
[CrossRef] [Google Scholar] [Publisher Link]
[8] Imane Worighi et al., “Integrating Renewable Energy in Smart Grid System: Architecture, Virtualization and Analysis,” Sustainable Energy, Grids and Networks, vol. 18, 2019.
[CrossRef] [Google Scholar] [Publisher Link]
[9] Hamed H.H. Aly, “A Proposed Intelligent Short-Term Load Forecasting Hybrid Models of ANN, WNN and KF based on Clustering Techniques for Smart Grid,” Electric Power Systems Research, vol. 182, 2020.
[CrossRef] [Google Scholar] [Publisher Link]
[10] K. Muralitharan, R. Sakthivel, and R. Vishnuvarthan, “Neural Network Based Optimization Approach for Energy Demand Prediction in Smart Grid,” Neurocomputing, vol. 273, pp. 199-208, 2018.
[CrossRef] [Google Scholar] [Publisher Link]
[11] Bilal Naji Alhasnawi et al., “A New Internet of Things Based Optimization Scheme of Residential Demand Side Management System,” IET Renewable Power Generation, vol. 16, no. 10, pp. 1992-2006, 2022.
[CrossRef] [Google Scholar] [Publisher Link]
[12] Ateeq Ur Rehman et al., “An Optimal Power Usage Scheduling in Smart Grid Integrated with Renewable Energy Sources for Energy Management,” IEEE Access, vol. 9, pp. 84619-84638, 2021.
[CrossRef] [Google Scholar] [Publisher Link]
[13] C. Swaminathan, and G.Nagarathinam, "A Perspective Observation of Power Generation using Wind Energy and its Benefits," SSRG International Journal of Industrial Engineering, vol. 3, no. 3, pp. 7-11, 2016.
[CrossRef] [Publisher Link]
[14] Sourabh Goyal et al., “Solar-Wind Hybrid Systems for Power Generation,” SSRG International Journal of Mechanical Engineering, vol. 6, no. 5, pp. 14-21, 2019.
[CrossRef] [Publisher Link]
[15] Ankita Borban, and Rakesh Singh Lodhi, "To Balance Power Demand and Power Availability through Renewable Energy in India," SSRG International Journal of Electrical and Electronics Engineering, vol. 2, no. 11, pp. 9-11, 2015.
[CrossRef] [Google Scholar] [Publisher Link]
[16] E. G. Swetala, P. Sujatha, and P. Bharath, "Automatic Load Frequency Control for Wind-Thermal Micro Grid Based on Deep Reinforcement Learning," SSRG International Journal of Electrical and Electronics Engineering, vol. 8, no. 8, pp. 1-8, 2021.
[CrossRef] [Publisher Link]
[17] V. Devaraj, and M. Kumaresan, "An Elite LOA-TFWO Approach for Load-Frequency Control of Islanded Micro-Grids Incorporating Renewable Sources," International Journal of Engineering Trends and Technology, vol. 70, no. 10, pp. 166- 187, 2022.
[CrossRef] [Google Scholar] [Publisher Link]