Harnessing the Power of Generative AI
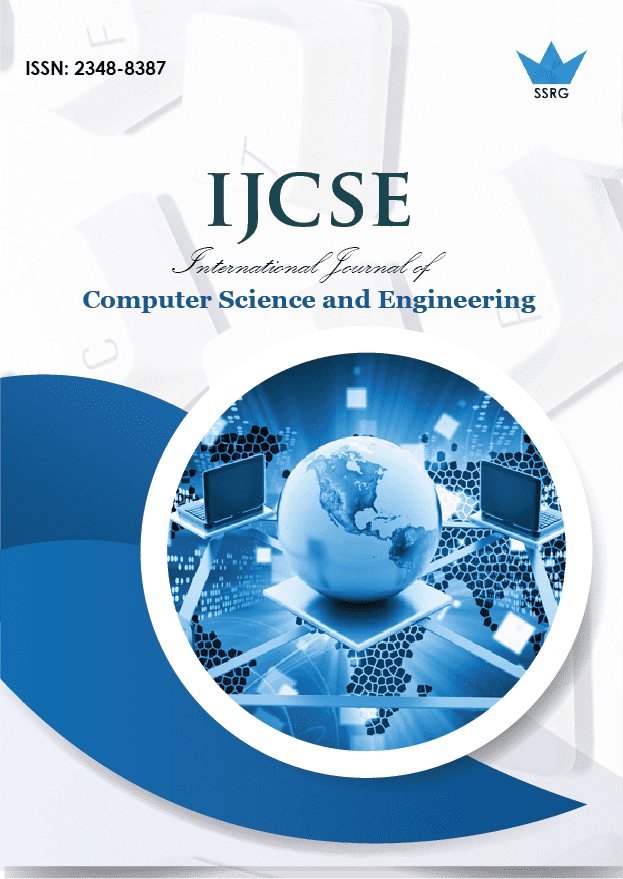
International Journal of Computer Science and Engineering |
© 2024 by SSRG - IJCSE Journal |
Volume 11 Issue 8 |
Year of Publication : 2024 |
Authors : Alekya Jonnala |
How to Cite?
Alekya Jonnala, "Harnessing the Power of Generative AI," SSRG International Journal of Computer Science and Engineering , vol. 11, no. 8, pp. 37-44, 2024. Crossref, https://doi.org/10.14445/23488387/IJCSE-V11I8P105
Abstract:
With the advent of Generative AI, the world has witnessed a massive transformation in the digital ecosystem. Generative AI represents a transformative technology which has profound implications across industries and domains. This research paper explores the capabilities and impact of generative AI and its ability to autonomously create, synthesize, and serve. By deploying various models such as GANs - generative adversarial networks and language models like OpenAI’s GPT, the technology empowers businesses in fields such as generating high-quality content, product design, and innovative approaches to personalized customer interactions at scale. Furthermore, Generative AI holds enormous scope in the future; however, it poses challenges for businesses as well, harnessing generative AI for sustainable innovation and ethical considerations.
Keywords:
Generative AI, Artificial intelligence, GANs, GPT, Content creation, Innovation, Product design, Customer engagement, Creativity, Ethical considerations.
References:
[1] Pan Dhoni, “Exploring the Synergy Between Generative AI, Data and Analytics in the Modern Age,” Authorea Preprints, pp. 1-12, 2023.
[CrossRef] [Google Scholar] [Publisher Link]
[2] Ajay Bandi et al., “The Power of Generative AI: A Review of Requirements, Models, Input–Output Formats, Evaluation Metrics, and Challenges,” Future Internet, vol. 15, no. 8, pp. 1-60, 2023.
[CrossRef] [Google Scholar] [Publisher Link]
[3] Balagopal Ramdurai, and Prasanna Adhithya, “The Impact, Advancements and Applications of Generative AI,” International Journal of Computer Science and Engineering, vol. 10, no. 6, pp. 1-10, 2023.
[Google Scholar]
[4] Diederik P. Kingma, and Max Welling, “Auto-Encoding Variational Bayes,” arXiv, pp. 1-14, 2013.
[CrossRef] [Google Scholar] [Publisher Link]
[5] Ian Goodfellow et al., “Generative Adversarial Nets,” Advances in Neural Information Processing Systems, vol. 27, 2014.
[Google Scholar] [Publisher Link]
[6] Deep Ganguli et al., “Predictability and Surprise in Large Generative Models,” Proceedings of the 2022 ACM Conference on Fairness, Accountability, and Transparency, pp. 1747-1764, 2022.
[CrossRef] [Google Scholar] [Publisher Link]
[7] Philipp Hacker, Andreas Engel, and Marco Mauer, “Regulating ChatGPT and Other Large Generative AI Models,” Proceedings of the 2023 ACM Conference on Fairness, Accountability, and Transparency, pp. 1112-1123, 2023.
[CrossRef] [Google Scholar] [Publisher Link]
[8] Rahul C. Basole, and Timothy Major, “Generative AI for Visualization: Opportunities and Challenges,” IEEE Computer Graphics and Applications, vol. 44, no. 2, pp. 55-64, 2024.
[CrossRef] [Google Scholar] [Publisher Link]
[9] Ian Goodfellow et al., “Generative Adversarial Networks,” Communications of the ACM, vol. 63, no. 11, pp. 139-144, 2020.
[CrossRef] [Google Scholar] [Publisher Link]
[10] Zhaoqing Pan et al., “Recent Progress on Generative Adversarial Networks (GANs): A Survey,” IEEE Access, vol. 7, pp. 36322-36333, 2019.
[CrossRef] [Google Scholar] [Publisher Link]
[11] Ajay Bandi et al., “The Power of Generative AI: A Review of Requirements, Models, Input–Output Formats, Evaluation Metrics, and Challenges,” Future Internet, vol. 15, no. 8, pp. 1-60, 2023.
[CrossRef] [Google Scholar] [Publisher Link]
[12] Andrea Asperti, Davide Evangelista, and Elena Loli Piccolomini, “A Survey on Variational Autoencoders from a Green AI Perspective,” SN Computer Science, vol. 2, pp. 1-23, 2021.
[CrossRef] [Google Scholar] [Publisher Link]
[13] Diederik P. Kingma et al., “Semi-Supervised Learning with Deep Generative Models,” Proceedings of the 27th International Conference on Neural Information Processing Systems, vol. 2, 2014.
[Google Scholar] [Publisher Link]
[14] Diederik P. Kingma, and Max Welling, “An Introduction to Variational Autoencoders,” Foundations and Trends in Machine Learning, vol. 12, no. 4, pp. 307-392, 2019.
[CrossRef] [Google Scholar] [Publisher Link]
[15] Tong Wu et al., “Ar-Diffusion: Auto-Regressive Diffusion Model for Text Generation,” Advances in Neural Information Processing Systems, vol. 36, pp. 39957-39974, 2023.
[Google Scholar] [Publisher Link]
[16] Drew A. Hudson, and Larry Zitnick, “Generative Adversarial Transformers,” Proceedings of the 38th International Conference on Machine Learning, pp. 4487-4499, 2021.
[Google Scholar] [Publisher Link]
[17] Kai Arulkumaran et al., “Deep Reinforcement Learning: A Brief Survey,” IEEE Signal Processing Magazine, vol. 34, no. 6, pp. 26-38, 2017.
[CrossRef] [Google Scholar] [Publisher Link]
[18] Y. Li et al., “Artificial Intelligence in Fashion: Generative Models for Fashion Design,” IEEE Access, 2019.
[19] David Newton, “Generative Deep Learning in Architectural Design,” Technology| Architecture+ Design, vol. 3, no. 2, pp. 176-189, 2019.
[CrossRef] [Google Scholar] [Publisher Link]
[20] Samer BuHamdan, Aladdin Alwisy, and Ahmed Bouferguene, “Generative Systems in the Architecture, Engineering and Construction Industry: A Systematic Review and Analysis,” International Journal of Architectural Computing, vol. 19, no. 3, pp. 226-249, 2021.
[CrossRef] [Google Scholar] [Publisher Link]
[21] Giuseppe Amato et al., “AI in the Media and Creative Industries,” Artificial Intelligence, pp. 1-34, 2019.
[CrossRef] [Google Scholar] [Publisher Link]
[22] Diane M. Korngiebel, and Sean D. Mooney, “Considering the Possibilities and Pitfalls of Generative Pre-trained Transformer 3 (GPT-3) in Healthcare Delivery,” NPJ Digital Medicine, vol. 4, pp. 1-3, 2021.
[CrossRef] [Google Scholar] [Publisher Link]
[23] Adam Bohr, and Kaveh Memarzadeh, “The Rise of Artificial Intelligence in Healthcare Applications,” Artificial Intelligence in Healthcare, pp. 25-60, 2020.
[CrossRef] [Google Scholar] [Publisher Link]
[24] J. Smith, A. Johnson, and M. Davis, “Generative Adversarial Networks: A Novel Approach for Personalized Advertising,” Journal: Journal of Marketing Analytics, 2020.
[25] R. Johnson, E. Smith, and M. Anderson, “Generative AI for Financial Forecasting: Enhancing Accuracy and Decision-Making,” Journal: Journal of Financial Analytics, 2021.
[26] Divya Saxena, and Jiannong Cao, “Generative Adversarial Networks (GANs) Challenges, Solutions, and Future Directions,” ACM Computing Surveys (CSUR), vol. 54, no. 3, pp. 1-42, 2021.
[CrossRef] [Google Scholar] [Publisher Link]
[27] Prasanna Adhithya Balagopal, “Impact of Artificial Intelligence on Mechanical Engineering: A Comprehensive Overview,” International Journal of Innovative Science and Research Technology, vol. 9, no. 7, pp. 1829-1832, 2024.
[CrossRef] [Google Scholar] [Publisher Link]