Early-Stage Detection of Alzheimer’s Disease Using Modified Firefly Based Ensemble Classification Model
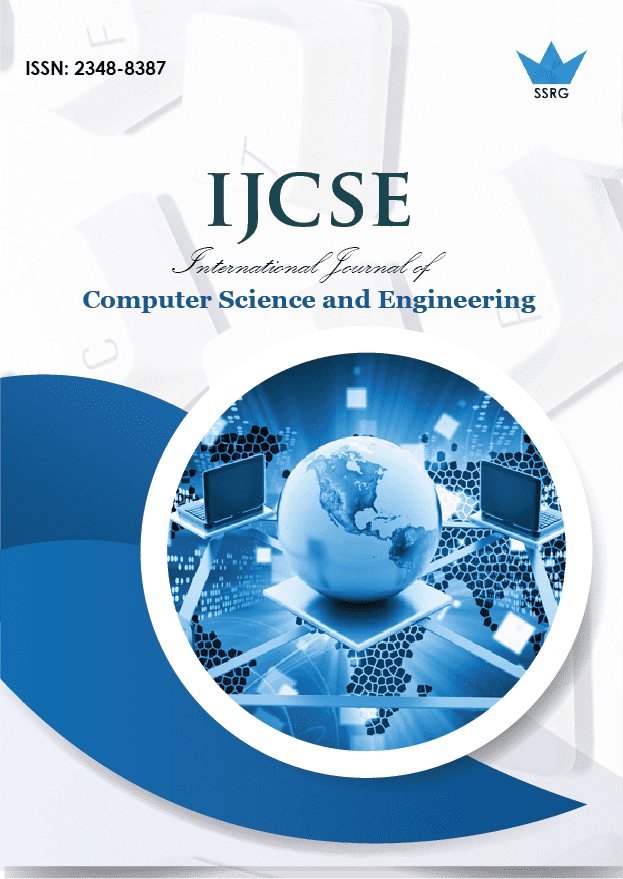
International Journal of Computer Science and Engineering |
© 2024 by SSRG - IJCSE Journal |
Volume 11 Issue 11 |
Year of Publication : 2024 |
Authors : Sri Lakshmi, Sreenu Babu |
How to Cite?
Sri Lakshmi, Sreenu Babu, "Early-Stage Detection of Alzheimer’s Disease Using Modified Firefly Based Ensemble Classification Model," SSRG International Journal of Computer Science and Engineering , vol. 11, no. 11, pp. 11-19, 2024. Crossref, https://doi.org/10.14445/23488387/IJCSE-V11I11P102
Abstract:
Alzheimer's disease (AD) is a progressive neurodegenerative disorder characterized by cognitive decline affecting millions of people globally. Early detection is crucial to managing the disease effectively, enabling timely intervention to slow its progression. Most conventional machine learning approaches have been explored to enhance early prediction; however, these techniques often suffer from issues like overfitting, complex feature selection, and the inability to handle large datasets efficiently. To address the challenges of early AD prediction in the proposed model, the Hybrid Firefly optimized feature selection along with One Class SVM was used to achieve a better quality of Biomarker data and further integrate it with a customized ensemble classification model to improve the prediction rate. The proposed model performs better than conventional models and is measured in terms of accuracy, recall, precision and F1 score.
Keywords:
Modified Firefly algorithm, One Class SVM, Ensemble Classification, XGboost, Feature Selection.
References:
[1] I. Murali Krishna et al., “Alzheimer's Disease Prediction Using an Enhanced Feature Selection of Multi Local Information Sharing Based on the ABC Algorithm,” Journal of Data Acquisition and Processing, vol. 39, no. 1, 2024.
[Google Scholar]
[2] Mehrdad Rostami et al., “Review of Swarm Intelligence-based Feature Selection Methods,” Engineering Applications of Artificial Intelligence, vol. 100, 2021.
[CrossRef] [Google Scholar] [Publisher Link]
[3] Afrah Salman Dawood, “A Comparative Study Using Deep Learning Models and Transfer Learning for Detection and Classification of Alzheimer’s Disease,” Iraqi Journal of Computers, Communications, Control & Systems Engineering (IJCCCE), vol. 24, no. 1, pp. 57 70, 2024.
[CrossRef] [Google Scholar] [Publisher Link]
[4] M. Sudharsan, and G. Thailambal, “Alzheimer’s Disease Prediction Using Machine Learning Techniques and Principal Component Analysis (PCA),” MaterialsToday Proceedings, vol. 81, no. 2, pp. 182-190, 2023.
[CrossRef] [Google Scholar] [Publisher Link]
[5] Petros Paplomatas et al., “An Ensemble Feature Selection Approach for Analysis and Modeling of Transcriptome Data in Alzheimer’s Disease,” Applied Science, vol. 13, no. 4, pp. 1-11, 2023.
[CrossRef] [Google Scholar] [Publisher Link]
[6] Qasem Al-Tashi et al., “Approaches to Multi-Objective Feature Selection: A Systematic Literature Review,” IEEE Access, vol. 8, pp. 125076-125096, 2020.
[CrossRef] [Google Scholar] [Publisher Link]
[7] Ashir Javeed et al., “Early Prediction of Dementia Using Feature Extraction Battery (FEB) and Optimized Support Vector Machine (SVM) for Classification,” Biomedicines, vol. 11, no. 2, pp. 1-13, 2023.
[CrossRef] [Google Scholar] [Publisher Link]
[8] Noushath Shaffi et al., “Bagging the Best: A Hybrid SVM-KNN Ensemble for Accurate and Early Detection of Alzheimer’s and Parkinson’s Diseases,” Brain Informatics:16th International Conference, Hoboken, NJ, USA, pp. 443-455, 2023.
[CrossRef] [Google Scholar] [Publisher Link]
[9] Ana Gabriela Sanchez-Reyna et al., “Feature Selection and Machine Learning Applied for Alzheimer’s Disease Classification,” VIII Latin American Conference on Biomedical Engineering and XLII National Conference on Biomedical Engineering, Cancún, México, pp. 121 128, 2020.
[CrossRef] [Google Scholar] [Publisher Link]
[10] Hala Alshamlan et al., “Identifying Effective Feature Selection Methods for Alzheimer’s Disease Biomarker Gene Detection Using Machine Learning,” Diagnostics, vol. 13, no. 10, pp. 1-14, 2023.
[CrossRef] [Google Scholar] [Publisher Link]
[11] Nicholas Pudjihartono et al., “A Review of Feature Selection Methods for Machine Learning-Based Disease Risk Prediction,” Frontiers in Bioinformatics, vol. 2, pp. 1-17, 2022.
[CrossRef] [Google Scholar] [Publisher Link]
[12] Ashir Javeed et al., “Predicting Dementia Risk Factors Based on Feature Selection and Neural Networks,” Computers, Materials & Continua, vol. 75, no. 2, pp. 2491-2508, 2022.
[CrossRef] [Google Scholar] [Publisher Link]
[13] Noushath Shaffi et al., “Performance Evaluation of Deep, Shallow and Ensemble Machine Learning Methods for the Automated Classification of Alzheimer’s Disease,” International Journal of Neural Systems, vol. 34, no. 7, pp. 1-17, 2024.
[CrossRef] [Google Scholar] [Publisher Link]
[14] Chitradevi Dhakhinamoorthy et al., “Hybrid Whale and Gray Wolf Deep Learning Optimization Algorithm for Prediction of Alzheimer’s Disease,” Mathematics, vol. 11, no. 5, pp. 1-17, 2023.
[CrossRef] [Google Scholar] [Publisher Link]
[15] Mohamadreza Khosravi, Hossein Parsaei, and Khosro Rezaee, “Novel Classification Scheme For Early Alzheimer’s Disease (AD) Severity Diagnosis Using Deep Features of the Hybrid Cascade Attention Architecture: Early Detection of AD on MRI Scans,” Tsinghua Science and Technology, pp. 1-20, 2024.
[CrossRef] [Google Scholar] [Publisher Link]
[16] Ranjan Kumar et al., “Ensemble Learning‑Based Early Detection of Influenza Disease,” Multimedia Tools and Applications, vol. 83, pp. 5723-5743, 2024.
[CrossRef] [Google Scholar] [Publisher Link]
[17] Sina Fathi et al., “A Deep Learning‑Based Ensemble Method for Early Diagnosis of Alzheimer’s Disease Using MRI Images,” Neuroinformatics, vol. 22, pp. 89-105, 2024.
[CrossRef] [Google Scholar] [Publisher Link]
[18] Aliaa El-Gawady, BenBella S. Tawfik, and Mohamed A. Makhlouf, “Hybrid Feature Selection Method for Predicting Alzheimer’s Disease Using Gene Expression Data,” Computers, Materials & ContinuaTechScience Press, vol. 74, no. 3, pp. 5559-5572, 2023.
[CrossRef] [Google Scholar] [Publisher Link]