Quantitative And Qualitative Analysis For Lung Nodule Segmentation
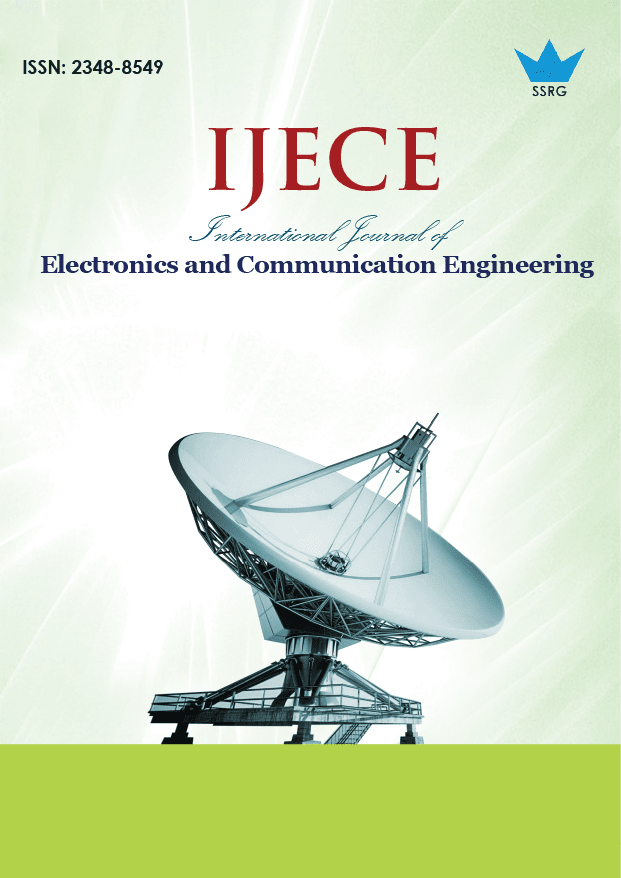
International Journal of Electronics and Communication Engineering |
© 2019 by SSRG - IJECE Journal |
Volume 6 Issue 5 |
Year of Publication : 2019 |
Authors : Rabiya Banu .A , Kannan.R |
How to Cite?
Rabiya Banu .A , Kannan.R, "Quantitative And Qualitative Analysis For Lung Nodule Segmentation," SSRG International Journal of Electronics and Communication Engineering, vol. 6, no. 5, pp. 16-21, 2019. Crossref, https://doi.org/10.14445/23488549/IJECE-V6I5P104
Abstract:
Prevention of lung cancer is the toughest problem due to cancer cells’ structure, where most of the cells are combined. The image processing techniques are mostly used for the prediction of lung cancer and also for early detection and treatment to prevent lung cancer. Various features are extracted from the 2D images; therefore, pattern recognition based approaches are useful for predicting lung cancer. 2D Image processing techniques are used in most medical areas for image improvement and analysis in earlier detection and treatment stages. The time factor is very important to discover the abnormality points in target images, especially in various cancer nodules such as lung cancer, breast cancer. Image quality assessment and efficiency are the main factors of this research; image quality assessment, as well as improvement, are depending on the enhancement stage where low pre-processing techniques are used based on the filter. Following the Output segmentation principles, an enhanced region of the object of interest used as a basic foundation of feature extraction is obtained. Relying on general features, a normality comparison is made. A comprehensive and comparative review for lung cancer prediction by a previous researcher using image processing techniques is presented. The objection for the prediction of lung cancer by a previous researcher using image processing techniques is also presented
Keywords:
Image processing, Segmentation, lung cancer, feature extraction.
References:
[1] S.Ganesh Prabhu, K. Vinotha, M. Shanthala, S. Subhashini, S. Vishnu, “IOT Based Home Automation and Security System”, SSRG International Journal of Electronics and Communication Engineering (SSRG-IJECE), vol. 4, no. 3, pp. 19-22, 2017.
[2] Ganesh Prabhu.S, Sangeetha.S, Shanmathi.S, Sharmila.M, “Automatic Toll E-Ticketing for Transportation Systems” SSRG International Journal of Electronics and Communication Engineering (SSRG-IJECE), vol. 4, no. 3, pp. 05-08, 2017.
[3] Ganesh Prabhu.S, R.R.Thirrunavukkarasu, S.Logesh Kumar, Karthik.S “Big Data Prologue Linking Cloud Computing to Ascertain the Smart Polling” International Journal of Current Engineering and Scientific Research ISSN (Print): 2393-8374, (Online): 2394-0697, Volume-4, Issue-9, 2017.
[4] Ganesh Prabhu.S, R.R.Thirrunavukkarasu, S.Logesh Kumar, Karthik.S “Lowering Glucose Elevations using Smart Head-on conjunction through virtual Congruence of Cloud on Internet” International Journal of Engineering Research in Computer Science and Engineering (IJERCSE) Vol 4, Issue 10, October 2017.
[5] R. Anirudh, J. J. Thiagarajan, and T. Bremer. (2016, Mar.). Lung nodule detection using 3D convolutional neural networks trained on weakly labeled data. Presented at SPIE Med. Imaging
[6] A. Mansoor, U. Bagci, B. Foster, Z. Xu, G. Z. Papadakis, L. R. Folio, J. K. Udupa, and D. J. Mollura, “Segmentation and image analysis of abnormal lungs at CT: current approaches, challenges, and future trends,” RadioGraphics, vol. 35, no. 4, pp. 1056-1076, 2015.
[7] L. Hedlund, R. F. Anderson, P. Goulding, J. Beck, E. Effmann, and C. Putman, “Two methods for isolating the lung area of a CT scan for density information,” Radiology, vol. 144, no. 2, pp. 353-357, 1982.
[8] X. Ye, X. Lin, J. Dehmeshki, G. Slabaugh, and G. Beddoe, “Shape-based computer-aided detection of lung nodules in thoracic CT images,” IEEE Trans. Biomed. Eng., vol. 56, no. 7, pp. 1810–1820, 2009.
[9] G. Aresta, A. Cunha, and A. Campilho, “Detection of juxta-pleural lung nodules in computed tomography images,” in Computer-Aided Diagnosis on Medical Imaging, 2017, pp. 101343N.
[10] G. J. Kemerink, R. J. Lamers, B. J. Pellis, H. H. Kruize, and J. Van Engelshoven, “On segmentation of lung parenchyma in quantitative computed tomography of the lung,” Medical Physics, vol. 25, no. 12, pp. 2432-2439, 1998.
[11] H. A. Gietema, Y. Wang, D. Xu, R. J. van Klaveren, H. de Koning, E. Scholten, J. Verschakelen, G. Kohl, M. Oudkerk, and M. Prokop, “Pulmonary nodules detected at lung cancer screening: interobserver variability of semiautomated volume measurements,” Radiology, vol. 241, no. 1, pp. 251-257, 2006.
[12] S. G. Armato, and W. F. Sensakovic, “Automated lung segmentation for thoracic CT: Impact on computer-aided diagnosis1,” Academic Radiology, vol. 11, no. 9, pp. 1011-1021, 2004.
[13] S. Hu, E. A. Hoffman, and J. M. Reinhardt, “Automatic lung segmentation for accurate quantitation of volumetric X-ray CT images,” IEEE transactions on medical imaging, vol. 20, no. 6, pp. 490-498, 2001.
[14] L. Hedlund, R. F. Anderson, P. Goulding, J. Beck, E. Effmann, and C. Putman, “Two methods for isolating the lung area of a CT scan for density information,” Radiology, vol. 144, no. 2, pp. 353-357, 1982.
[15] Ebanesar.C, Hamsa Vagini R, Sangeetha Bregit.A, J Dinesh Peter, “Computer Aided System for Automated Heterogeneous Cancer Recognition using Google Cloud Platform” SSRG International Journal of Computer Science and Engineering 5.5 (2018): 11-16.