Performance Enhancement of Image Stitching Process Under Bound Energy aided feature matching and Varying Illumination Environments
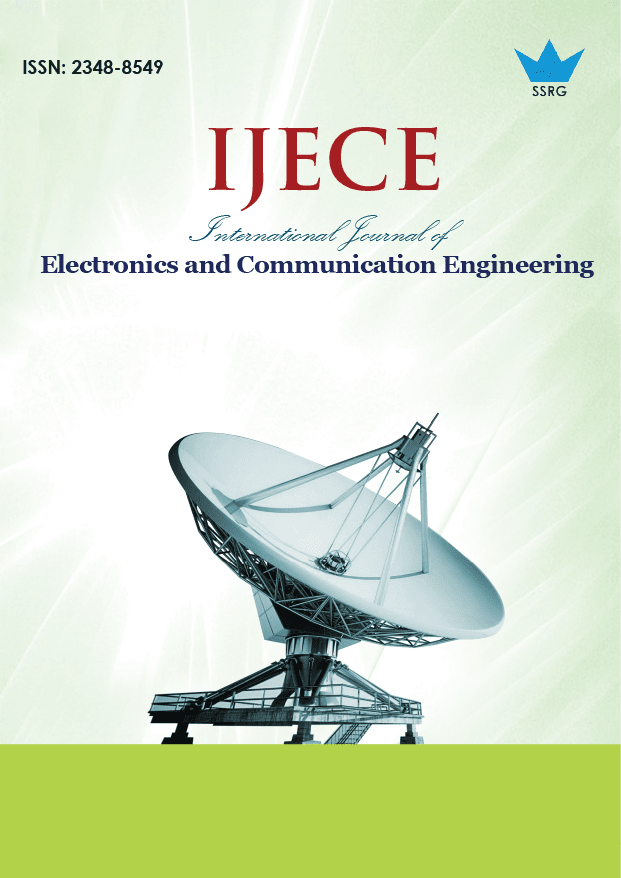
International Journal of Electronics and Communication Engineering |
© 2019 by SSRG - IJECE Journal |
Volume 6 Issue 9 |
Year of Publication : 2019 |
Authors : Venkat P. Patil, Dr. C. Ram Singla |
How to Cite?
Venkat P. Patil, Dr. C. Ram Singla, "Performance Enhancement of Image Stitching Process Under Bound Energy aided feature matching and Varying Illumination Environments," SSRG International Journal of Electronics and Communication Engineering, vol. 6, no. 9, pp. 1-6, 2019. Crossref, https://doi.org/10.14445/23488549/IJECE-V6I9P101
Abstract:
Stitched images is a method that mixes several images or images of the intersecting perspective field to create a high-resolution panoramic image. In the field of medical imagery, satellite data, computer vision, military automatic target identifiers, we can see the importance of image mosaicing. The fields of computer vision, photography and computer graphics are currently being researched on image stitching and video stitching. Registration of images includes five major steps: detection of image features and its description; matching features; outsider rejection; deriving transformation function; and reproduction of images. Stitching images of similar images is a difficult job when images are captured in variable light conditions. In this paper, we have examined image panorama development which is based on seamless image stitching to overcome the above mentioned problems by adopting the de-hazing technique on the acquired wide view scenes. This is achieved further before identifying the image functionalities and bound energy-energized features that match the image's invariant scale attributes (SIFT). Compared to the current image stitching techniques the experimentation of the suggested model uses squared distance to match the features. The suggested seamless stitchable technique is assessed on the basis of metrics VSGV and HSGV called as “Vertical Square Gradient Value” and “Horizontal Square Gradient Value” and respectively. Analysis of the aforementioned stitching algorithm aims at reducing the amount of computation time and inconsistencies in the stitched result obtained.
Keywords:
Bound Energy, ASIFT, FAST, FREAK, Panorama.
References:
[1] Alahi, A., Ortiz, R., Vandergheynst, P., 2012. “FREAK: Fast retina keypoint,” IEEE Conference on Computer Vision and Pattern Recognition, Providence, RI, pp. 510–517.
[2] Brown, M., Lowe, D.G., 2007. “Automatic panoramic image stitching using invariant features.” Int. J. Comput. Vis. 74 (1), 59–73.
[3] Ganesa Moorthy, N., Nandhini Devi, S., 2014. “Outlier detection through unsupervised approach with bound energy.” Int. J. Emerg. Technol. Comp. Sci. Electron. 7 (1), 154–158.
[4] Hua, Zhen, Li, Yewei, Li, Jinjiang, 2010.,” Image stitch algorithm based on SIFT and MVSC”, In: 2010 Seventh In proceedings of IEEE International Conference on Fuzzy Systems and Knowledge Discovery (FSKD), vol. 6, pp. 2628–2632.
[5] Huang, C.M., Lin, S.W., Chen, J.H., 2015.” Efficient image stitching of continuous image sequence with image and seam selections.” IEEE Sens. J. 15 (10), 5910– 5918.
[6] Lei, Z., Liu, X., Zhao, L., Chen, L., Li, Q., Yuan, T., Lu, W., 2016. “A novel 3D stitching method for WLI based large range surface topography measurement. “ Optics Commun. 359, 435–447.
[7] Li, Y., Wang, Y., Huang, W., Zhang, Z., 2008. “Automatic image stitching using sift”. In: proceedings of IEEE International Conference on Audio, Language and Image Processing, pp. 568–571.
[8] Li, W., Jin, C.B., Liu, M., Kim, H., Cui, X., 2018.” Local similarity refinement of shape preserved warping for parallax-tolerant image stitching”. IET Image Process. 12 (5), 661–668.
[9] Lowe, David G., 2004. “Distinctive Image features from Scale Invariant Keypoints.” J. Comp. Vision 60 (2), 91–110.
[10] Lu, Y., Hua, Z., Gao, K., Xu, T., 2018. “Multiperspective image stitching and regularization via hybrid structure warping”. Comput. Sci. Eng. 20 (2), 10–23.
[11] Peleg, S., 1981.” Elimination of seams from photomosaics.” Comput. Graph. Image Process. 16 (1), 90–94.
[12] Rosten, E., Porter, R., Drummond, T., 2010. “Faster and better: a machine learning approach to corner detection.” IEEE Trans. Pattern Anal. Mach. Intell. 32 (1), 105– 119.
[13] Shu, Wu., Wang, Shengrui, 2013. “Information-Theoretic outlier detection for largescale categorical data”. IEEE Trans. Knowl. Data Eng. 25 (3), 589–602.
[14] Song, T., Jeon, C., Ko, H., 2015. “Image stitching using chaos-inspired dissimilarity measure”. Electron. Lett. 51 (3), 232–234.
[15] Yang, Z.L., Guo, B.L., 2008. “Image mosaic based on SIFT.” In: Proceedings of IEEE International Conference on Intelligent Information Hiding and Multimedia Signal Processing, pp. 1422–1425.
[16] Yang, X.H., Wang, M., 2013. “Seamless image stitching method based on ASIFT”. Comput. Eng. 39 (2), 241–244.
[17] Zhi, Q., Cooperstock, J.R., 2012. “Toward dynamic image mosaic generation with robustness to parallax”. IEEE Trans. Image Process. 21 (1), 366–378.
[18] Ma. Xiaomin Liu Ding and Zhang Jian "A fast affine invariant features for image stitching under large viewpoint changes". Neurocomputing vol. 3 no. 151 2015 pp. 1430-1438.
[19] Boykov, Y., Kolmogorov, V., 2004. “An experimental comparison of mincut/max-flow algorithms for energy minimization” in vision. IEEE Trans. Pattern Anal. Mach. Intell. 26 (9), 1124–1137. [20] V. P Patil, UB Gohatre,”Performance comparison of image stitching methods under different illumination conditions “ ,Int J Eng Technol Sci Res 4 (9), 249-59
[21] Liu, R., Li, Z., Jia, J., 2008.” Image partial blur detection and classification”. In: Proc. IEEE Conf. Comput. Vis. Pattern Recognit. (CVPR), pp. 1–8.
[22] Summa, B., Tierny, J., Pascucci, V., 2012. “Panorama weaving: fast and flexible seam processing”. ACM Trans. Graph. (TOG) 31 (4), 83. [23] Guoshen Yu, and Jean-Michel Morel, “ASIFT: An Algorithm for Fully Affine Invariant Comparison”, Image Processing On Line, 1 (2011), pp. 11–38.
[24] http://web.cecs.pdx.edu/