Medical Image Segmentation Approaches: A Survey
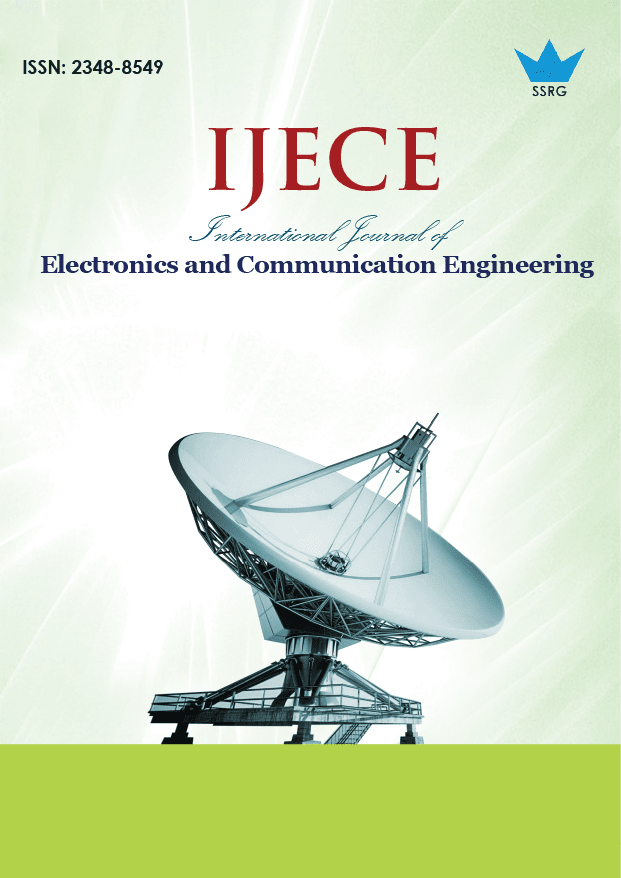
International Journal of Electronics and Communication Engineering |
© 2020 by SSRG - IJECE Journal |
Volume 7 Issue 7 |
Year of Publication : 2020 |
Authors : Nageswari P, Rajan S, Manivel K |
How to Cite?
Nageswari P, Rajan S, Manivel K, "Medical Image Segmentation Approaches: A Survey," SSRG International Journal of Electronics and Communication Engineering, vol. 7, no. 7, pp. 1-3, 2020. Crossref, https://doi.org/10.14445/23488549/IJECE-V7I7P101
Abstract:
Segmentation is an important preprocessing step in medical image analysis. Its main aim is to partition the image areas into several regions concerning similar distinctiveness such as texture, color, and intensity. It has been widely useful in many purposes such as recognition of tumors and coronary borders, planning for surgery, measuring the tumor volume, blood cell classification, and extraction of heart image from cardiac cine angiograms. In recent years, for the medical image, many approaches have been proposed for the segmentation process. Thresholding, region-based, edge-based, and clustering-based methods are the important segmentation process techniques for medical image analysis. This paper studies the different types of segmentation approaches that have been used in medical imaging frameworks.
Keywords:
Segmentation, Medical image, Speckle noise, Fuzzy Clustering Technique.
References:
[1] Sahoo, P.K., Soltani, S., Wong, A.K., 1988. “A survey of thresholding techniques Computer Vision”, Graphics and Image Processing, 41, 233–260.
[2] R. S. A. V. K. V. Shrimali, “Current trends in segmentation of medical ultrasound B-mode images: A review,” IETE Tech. Rev., Vol. 1, no. 8_17, pp. 26, 2009.
[3] Adams, R., Bischof, L., 1994. Seeded region growing. IEEE Transactions on Pattern Analysis and Machine Intelligence (IEEE TPAMI) 16, 641–647.
[4] Pan, Z., Lu, J., 2007. A Bayes-based region-growing algorithm for medical image segmentation”, computing in science and Engineering 9, 32–38.
[5] Nithila EE, Kumar SS (2016) Segmentation of lung nodule in CT data using active contour model and fuzzy C-mean clustering. Alex Eng J 55:2583–2588
[6] Simhachalam B, Ganesan G (2016) Performance comparison of fuzzy and non-fuzzy classification methods. Egypt Inform J 2016(17):183–188.
[7] Yin S, Qian Y, Gong M (2017) “Unsupervised hierarchical image segmentation through fuzzy entropy maximization”. Pattern Recogn 68:245–269.
[8] Ji J, Wang K-L (2014) “A robust nonlocal fuzzy clustering algorithm with between-cluster separation measure for SAR image segmentation”. IEEE J Sel Top Appl Earth Obs Remote Sens 7(12):4929–4936.
[9] Balla-Arabe S, Gao X, Wang B (2013) “A fast and robust level set method for image segmentation using fuzzy clustering and lattice Boltzmann method”. IEEE Trans Cybern 43(3):910–920.
[10] Barkana, B. D., Saricicek, I., & Yildirim, B. (2017). Performance analysis of descriptive statistical features in retinal vessel segmentation via fuzzy logic, ANN, SVM, and classifier fusion. Knowledge-Based Systems, 118, 165-176.
[11] Ananthi VP, Balasubramaniam P, Kalaiselvi T (2016) “A new fuzzy clustering algorithm for the segmentation of brain tumor”. Soft Comput 20:4859–4879
[12] N.Pradeepa, A.Renuga Devi, Saumiya Jose Thomas, “Survey of MRI Brain Image Segmentation Methods”, SSRG International Journal of Electronics and Communication Engineering, Vol 2 Issue 2 2015.
[13] N.Pradeepa , A.Renuga Devi and Saumiya Jose Thomas,(2015). Survey of MRI Brain Image Segmentation Methods. SSRG International Journal of Electronics and Communication Engineering 2.2, 21-23.
[14] Nookala Venu , B. Anuradha "Two Different Multi-Kernels for Fuzzy C-means Algorithm for Medical Image Segmentation", International Journal of Engineering Trends and Technology (IJETT), V20(2),77-82 Feb 2015. ISSN:2231-5381.
[15] K.Bhargavi, Dr. T. Sreenivasulu Reddy "Segmentation and Classification for Brain MRI Image Based on Modified FCM with Zernike Moment Classifier", International Journal of Engineering Trends and Technology (IJETT), V44(2),66-71 February 2017. ISSN:2231-5381.