Advanced Approaches to Brain Tumor Classification and Diagnosis
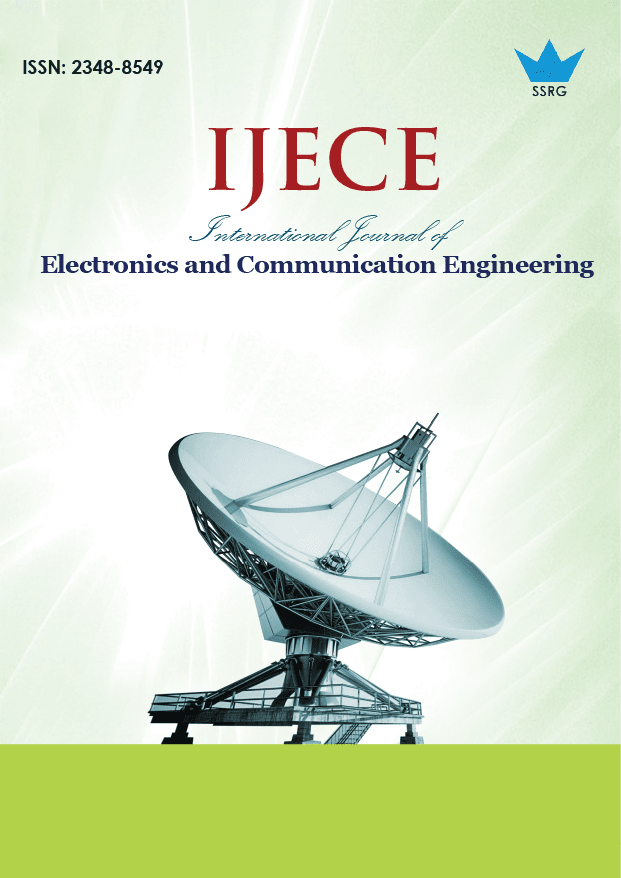
International Journal of Electronics and Communication Engineering |
© 2022 by SSRG - IJECE Journal |
Volume 9 Issue 1 |
Year of Publication : 2022 |
Authors : G Jeyalakshmi, K.A Shahul Hameed |
How to Cite?
G Jeyalakshmi, K.A Shahul Hameed, "Advanced Approaches to Brain Tumor Classification and Diagnosis," SSRG International Journal of Electronics and Communication Engineering, vol. 9, no. 1, pp. 6-9, 2022. Crossref, https://doi.org/10.14445/23488549/IJECE-V9I1P102
Abstract:
In hospitals, the data about the presence andposition of brain tumours (BT) is important to support clinicians in analysis and treatment. Automatic BT segmentation on the images attained by magnetic resonance imaging (MRI) is the best method to achieve this data. Recently, machine learning (ML) and deep learning (DL) algorithms have been introduced to accurately process MRI images to classify brain tumour stages. This article reviews various ML and DL algorithms proposed in brain tumour segmentation and classification algorithms.
Keywords:
A brain tumour, Deep learning and Machine learning.
References:
[1] Tongxue Zhou et al., “Latent Correlation Representation Learning for Brain Tumor Segmentation with Missing MRI Modalities,” IEEE Transactions on Image Processing, vol. 30, pp. 4263–4274, 2021. Crossref, https://doi.org/10.1109/tip.2021.3070752
[2] Biting Yu et al., “SA-LUT-Nets: Learning Sample-Adaptive Intensity Lookup Tables for Brain Tumor Segmentation,” IEEE Transactions onMedical Imaging, vol. 40, no. 5, pp. 1417–1427, 2021. Crossref, https://doi.org/10.1109/tmi.2021.3056678
[3] Liang Chen et al., “Drinet for Medical Image Segmentation,” IEEE Transactions on Medical Imaging, vol. 37, no. 11, pp. 1–1, 2018. Crossref, https://doi.org/10.1109/tmi.2018.2835303
[4] Zhenyu Tang et al., “Multi-Atlas Segmentation of MR Tumor Brain Images Using Low-Rank Based Image Recovery,” IEEE Transactions on Medical Imaging, vol. 37, no. 10, pp. 1– 1, 2018. Crossref, https://doi.org/10.1109/TMI.2018.2824243
[5] Chao Ma et al., “Concatenated and Connected Random Forests with Multiscale Patch Driven Active Contour Model for Automated Brain Tumor Segmentation of MR Images,” IEEETransactions on Medical Imaging, vol. 37, no. 8, pp. 1943–1954, 2018. Crossref, https://doi.org/10.1109/tmi.2018.2805821
[6] Mohammad Shahjahan Majib et al., “VGG-Scnet: A VGG Net-Based Deep Learning Framework for Brain Tumor Detection on MRI Images,” IEEE Access, vol. 9, pp. 116942–116952, 2021. Crossref, https://doi.org/10.1109/access.2021.3105874
[7] Amran Hossain et al., “Yolov3 Deep Neural Network Model To Detect Brain Tumor in Portable Electromagnetic Imaging System,” IEEE Access, vol. 9, pp. 82647– 82660, 2021. Crossref, https://doi.org/10.1109/access.2021.3086624 G Jeyalakshmi & K.A Shahul Hameed / IJECE, 9(1), 6-9, 2022 9
[8] Abbas Khan, Hyongsuk Kim, and Leon Chua “PMED-Net: Pyramid Based Multi-Scale Encoder-Decoder Network for Medical Image Segmentation,” IEEE Access, vol. 9, pp. 55988–55998, 2021. Crossref, https://doi.org/10.1109/ACCESS.2021.3071754
[9] Khurram Ejaz, “Hybrid Segmentation Method with Confidence Region Detection for Tumor Identification,” IEEE Access, pp. 1– 1, 2020. Crossref, https://doi.org/10.1109/ACCESS.2020.3016627
[10] Juhun Lee, and Robert M Nishikawa, "Cross-Organ, Cross-Modality Transfer Learning: Feasibility Study for Segmentation and Classification,” IEEE Access, vol. 8, pp. 210194–210205, 2020. Crossref, https://doi.org/10.1109/access.2020.3038909
[11] Afnan M. Alhassan, and Wan Mohd Nazmee Wan Zainon, “BAT Algorithm with Fuzzy C-Ordered Means (BAFCOM) Clustering Segmentation and Enhanced Capsule Networks (ECN) for Brain Cancer MRI Images Classification,” IEEE Access, vol. 8, pp. 201741–201751, 2020. Crossref, https://doi.org/10.1109/access.2020.3035803
[12] Nagwa M. Aboelenein et al., “HTTU-Net: Hybrid Two Track U-Net for Automatic Brain Tumor Segmentation,” IEEE Access, vol. 8, pp. 1–1, 2020. Crossref, https://doi.org/10.1109/ACCESS.2020.2998601
[13] Jianxin Zhang et al., “Attention Gate Resu-Net for Automatic MRI Brain Tumor Segmentation,” IEEE Access, vol. 8, pp. 58533– 58545, 2020. Crossref, https://doi.org/10.1109/access.2020.2983075
[14] Abdu Gumaei et al., “A Hybrid Feature Extraction Method with Regularized Extreme Learning Machine for Brain Tumor Classification,” IEEE Access, vol. 7, pp. 1–1, 2019. Crossref, https://doi.org/10.1109/access.2019.2904145