Hyperspectral Image Classification using Deep Learning Techniques: A Review
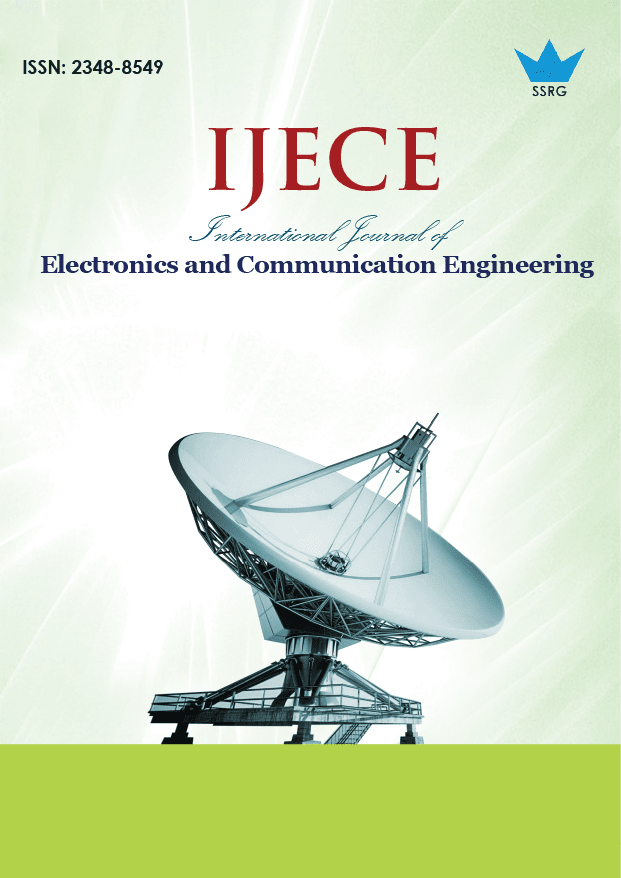
International Journal of Electronics and Communication Engineering |
© 2022 by SSRG - IJECE Journal |
Volume 9 Issue 6 |
Year of Publication : 2022 |
Authors : Syeda Sara Samreen, Hakeem Aejaz Aslam |
How to Cite?
Syeda Sara Samreen, Hakeem Aejaz Aslam, "Hyperspectral Image Classification using Deep Learning Techniques: A Review," SSRG International Journal of Electronics and Communication Engineering, vol. 9, no. 6, pp. 1-4, 2022. Crossref, https://doi.org/10.14445/23488549/IJECE-V9I6P101
Abstract:
Hyperspectral image classification is a salient topic of research in the domain of remote sensing. The major problems faced during hyperspectral image classification are the curse of dimensionality and the availability of limited samples during training. It was originally developed for mining and geology purposes to identify hidden minerals. Hyperspectral Imaging has various applications in geosciences, agriculture, astrology, and surveillance. The advancement in computing technology has led to the development of significant deep-learning techniques that play an important role in successfully classifying remotely sensed data. This paper reviews the various deep-learning methods used for hyperspectral image classification. Then the research gaps and methodology for every paper have been highlighted. This paper aims to benefit and support other researchers in further research in this field.
Keywords:
Convolutional Neural Network, Deep Belief Network, Generative Adversarial Network, Recurrent Neural Network, Remote Sensing.
References:
[1] Radhesyam Vaddi, and Prabukumar Manoharan, “Hyperspectral Image Classification Using CNN with Spectral and Spatial Feature Integration,” Infrared Physics & Technology, vol. 107, pp. 103-296, 2020. Crossref, https://doi.org/10.1016/j.infrared.2020.103296
[2] Ying Li, Haokui Zhang, and Qiang Shen, “Spectral–Spatial Classification of Hyperspectral Imagery with 3D Convolutional Neural Network,” IEEE Transactions on Geoscience and Remote Sensing, vol. 9, no. 1, pp. 1-21, 2017. Crossref, https://doi.org/10.3390/rs9010067
[3] Lichao Mou et al., “Deep Recurrent Neural Networks for Hyperspectral Image Classification,” IEEE Transactions on Geoscience and Remote Sensing, vol. 55, no. 7, pp. 3639-3655, 2017. Crossref, https://doi.org/10.1109/TGRS.2016.2636241
[4] Hao Wu, and Saurabh Prasad “Convolutional Recurrent Neural Networks for Hyperspectral Data Classification,” IEEE Transactions on Remote Sensing, vol. 9, no. 3, pp. 298, 2017.
[5] Ping Zhong et al., “Learning to Diversify Deep Belief Networks for Hyperspectral Image Classification,” IEEE Transactions on Geoscience and Remote Sensing, vol. 55, no. 6, pp. 3516-3530, 2017. Crossref, https://doi.org/10.3390/rs9030298
[6] Jiaojiao Li et al., “Hyperspectral Classification Based on Texture Feature Enhancement and Deep Belief Networks,” Remote Sensing, vol. 10, no. 3, pp. 396, 2018. Crossref, https://doi.org/10.3390/rs10030396
[7] Kun Tan et al., “A Parallel Gaussian–Bernoulli Restricted Boltzmann Machine for Mining Area Classification with Hyperspectral Imagery,” IEEE Journal of Selected Topics in Applied Earth Observations and Remote Sensing, vol. 12, no. 2, pp. 627-636, 2019. Crossref, https://doi.org/10.1109/JSTARS.2019.2892975
[8] Jiang Zhu et al., “Auto-Encoder Based for High Spectral Dimensional Data Classification and Visualization,” IEEE Second International Conference on Data Science in Cyberspace (DSC), pp. 350-354, 2017. Crossref, https://doi.org/10.1109/DSC.2017.32
[9] Aidin Hassanzadeh, Arto Kaarna, and Tuomo Kauranne, “Unsupervised Multi-Manifold Classification of Hyperspectral Remote Sensing Images with Contractive Autoencoder,” Scandinavian Conference on Image Analysis, pp. 169-180, 2017. Crossref, http://dx.doi.org/10.1007/978-3-319-59129-2_15
[10] Radford A, Metz L, and Chintala S, “Unsupervised Representation Learning with Deep Convolutional Generative Adversarial Networks,” ArXiv, 2015. Crossref, https://doi.org/10.48550/arXiv.1511.06434
[11] Ying Zhan et al., “Semi-Supervised Hyperspectral Image Classification Based on Generative Adversarial Networks,” IEEE Geoscience and Remote Sensing Letters, vol. 15, no. 2, pp. 212-216, 2017. Crossref, https://doi.org/10.1109/LGRS.2017.2780890