An Enhanced Framework for Categorization of Fruits Based on Ripeness using Ensemble PSO Model
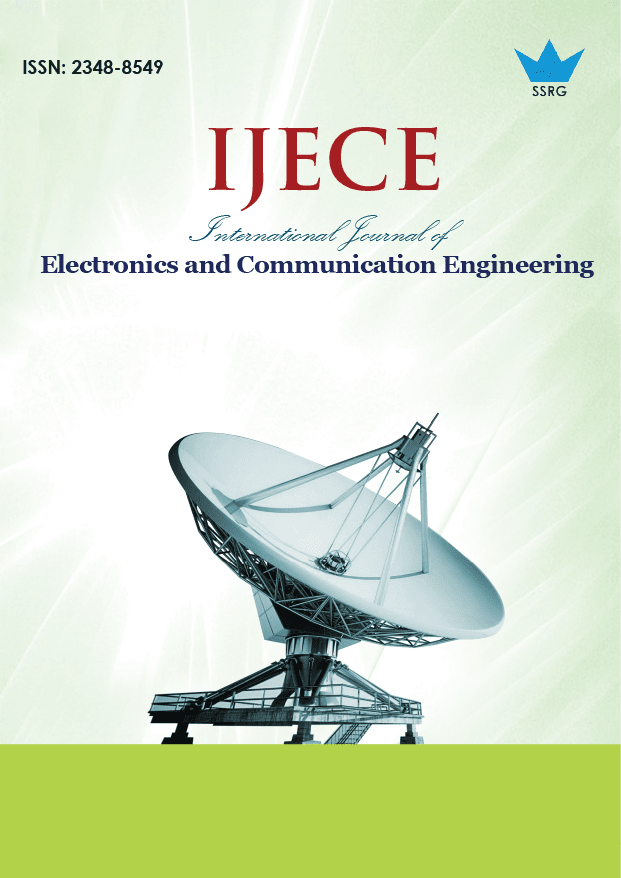
International Journal of Electronics and Communication Engineering |
© 2023 by SSRG - IJECE Journal |
Volume 10 Issue 5 |
Year of Publication : 2023 |
Authors : Muthulakshmi Arumugasamy, A. Antonidoss |
How to Cite?
Muthulakshmi Arumugasamy, A. Antonidoss, "An Enhanced Framework for Categorization of Fruits Based on Ripeness using Ensemble PSO Model," SSRG International Journal of Electronics and Communication Engineering, vol. 10, no. 5, pp. 76-84, 2023. Crossref, https://doi.org/10.14445/23488549/IJECE-V10I5P107
Abstract:
Ripeness-based categorization is a vital function after the harvest of fruits to balance the ripening treatment. Ripeness level is the significant factor determining the quality and lifetime of fruits. Various attributes are used to identify the ripeness level, of which the manual method of categorizing based on skin colour is predominantly used. This method is prone to error at many times. This paper implements an Ensemble Particle Swarm Optimization (EnPSO) algorithm to categorize the fruits based on the ripeness level. The comparison reveals that the proposed EnPSO algorithm outperforms the state-of-the-art algorithms used for the same problem. Categorization is done using the decision tree, which provides up to 98% classification accuracy.
Keywords:
Categorization, Ensemble particle swarm optimization, Quality and lifetime, Ripeness level
References:
[1] Alishba Adeelet al., “Entropy-Controlled Deep Features Selection Framework for Grape Leaf Diseases Recognition,” Expert Systems,
vol. 39, no. 7, p. 125729, 2022.
[CrossRef] [Google Scholar] [Publisher Link]
[2] Alishba Adeel et al., “Diagnosis and Recognition of Grape Leaf Diseases: An Automated System Based on a Novel Saliency Approach
and Canonical Correlation Analysis Based Multiple Features Fusion,” Sustainable Computing: Informatics and Systems, vol. 24, p.
100349, 2019.
[CrossRef] [Google Scholar] [Publisher Link]
[3] Khursheed Aurangzeb et al., “Advanced Machine Learning Algorithm Based System for Crops Leaf Diseases Recognition,” 6th
Conference on Data Science and Machine Learning Applications (CDMA), 2020.
[CrossRef] [Google Scholar] [Publisher Link]
[4] ArchanaChaudharyet al., “A Particle Swarm Optimization Based Ensemble for Vegetable Crop Disease Recognition,” Computers and
Electronics in Agriculture, vol. 178, p. 105747, 2020.
[CrossRef] [Google Scholar] [Publisher Link]
[5] Senthilarasi Marimuthu, and S. Mohamed Mansoor Roomi, “Particle Swarm Optimized Fuzzy Model for the Classification of Banana
Ripeness,” IEEE Sensors Journal, vol. 17, no.15, pp. 4903-4915, 2017.
[CrossRef] [Google Scholar] [Publisher Link]
[6] Chaitali Gaikwad, “View on Normal and Affected Fruit Classification,” International Journal on Recent and Innovation Trends in
Computing and Communication, vol. 3, no. 5, pp. 3425-3428, 2015.
[Google Scholar] [Publisher Link]
[7] Devi N et al., “Categorizing Diseases from Leaf Images Using a Hybrid Learning Model,” Symmetry, vol. 13, no. 11, p. 2073, 2021.
[CrossRef] [Google Scholar] [Publisher Link]
[8] D. Jude Hemanth, Omer Deperlioglu, and Utku Kose, “An Enhanced Diabetic Retinopathy Detection and Classification Approach
Using Deep Convolutional Neural Network,” Neural Computing and Applications, vol. 32, no. 3, pp. 707-721, 2020.
[CrossRef] [Google Scholar] [Publisher Link]
[9] Wei Wang, and Jianxun Gang, “Application of Convolutional Neural Network in Natural Language Processing,” International
Conference on Information Systems and Computer Aided Education (ICISCAE), 2018.
[CrossRef] [Google Scholar] [Publisher Link]
[10] Stephen D. Liang, “Optimization for Deep Convolutional Neural Networks: How Slim Can It Go?,” IEEE Transactions on Emerging
Topics in Computational Intelligencem, vol. 4, no. 2, pp. 171-179, 2020.
[CrossRef] [Google Scholar] [Publisher Link]
[11] Anuradha Gawande, and S.S. Dhande, “Implementation of Fruits Grading and Sorting System by Using Image Processing and Data
Classifier,” SSRG International Journal of Computer Science and Engineering, vol. 2, no. 6, pp. 22-27, 2015.
[CrossRef] [Google Scholar] [Publisher Link]
[12] John D. Kelleher, Deep Learning, MIT Press, 2019.
[Google Scholar] [Publisher Link]
[13] Yanan Sun et al., “Evolving Deep Convolutional Neural Networks for Image Classification,” IEEE Transactions on Evolutionary
Computation, vol. 24, no. 2, pp. 394-407, 2019.
[CrossRef] [Google Scholar] [Publisher Link]
[14] Yanan Sun, Gary G. Yen, and Zhang Yi, “Evolving Unsupervised Deep Neural Networks for Learning Meaningful Representations,”
IEEE Transactions on Evolutionary Computation, vol. 23, no. 1, pp. 89-103, 2018.
[CrossRef] [Google Scholar] [Publisher Link]
[15] M. Rajeshwari, and K. Rathika, “Palm Print Recognition Using Texture and Shape Features,” SSRG International Journal of Computer
Science and Engineering, vol. 9, no. 2, pp. 1-5, 2022.
[CrossRef] [Publisher Link]
[16] Gargi Sharma, and Gourav Shrivastava, “Crop Disease Prediction Using Deep Learning Techniques - A Review,” SSRG
InternationalJournal of Computer Science and Engineering, vol 9, no. 4, pp.23-28, 2022.
[CrossRef] [Publisher Link]
[17] A. Bindhu, and K.K. Thanammal, “Analytical Study on Digital Image Processing Applications,” SSRG International Journal of
Computer Science and Engineering, vol. 7, no. 6, pp. 4-7, 2020.
[CrossRef] [Google Scholar] [Publisher Link]
[18] K. Archana, and K.G.Saranya, “Crop Yield Prediction, Forecasting and Fertilizer Recommendation Using Voting Based Ensemble
Classifier,” SSRG International Journal of Computer Science and Engineering, vol. 7, no. 5, pp. 1-4, 2020.
[CrossRef] [Google Scholar] [Publisher Link]
[19] Mansi Shinde et al., “Crop Recommendation and Fertilizer Purchase System,” IJCSIT International Journal of Computer Science and
Information Technologies, vol. 7, no. 2, pp. 665-667, 2016.
[Google Scholar] [Publisher Link]
[20] V. Sellam, and E. Poovammal, “Prediction of Crop Yield using Regression Analysis,” IJST Indian Journal of Science and Technology,
vol. 9, no. 38, pp. 1-5, 2016.
[CrossRef] [Google Scholar] [Publisher Link]
[21] U.K. Diwan et al., “Yield Prediction of Wheat at Pre-Harvest Stage Using Regression-Based Statistical Model for 8 District of Chhattisgarh, India,” IJCMAS International Journal of Current Microbiology and Applied Sciences, vol. 7, no. 1, pp. 2180-2183, 2018.
[CrossRef] [Google Scholar] [Publisher Link]
[22] Rushika Ghadge et al., “Prediction of Crop Yield using Machine Learning,” IRJET International Research Journal of Engineering and Technology, vol. 5, no. 2, pp. 2237-2239, 2018.
[Google Scholar] [Publisher Link]
[23] P.Priya, U.Muthaiah, and M.Balamurugan, “Predicting Yield of the Crop Using Machine Learning Algorithm,” IJESRT International Journal of Engineering Sciences & Research Technology, vol. 7, no. 4, 2018.
[Google Scholar] [Publisher Link]
[24] Vaneesbeer Singh, Vinod Sharma, and AbidSarwar, “Analysis of Soil and Prediction of Crop Yield (Rice) Using Machine Learning Approach,” International Journal of Advanced Research in Computer Science, Vol. 8, no. 5, pp. 1254-1259, 2017.
[Google Scholar] [Publisher Link]
[25] Vrushal Milan Dolas, and Uday Joshi, “A Novel Approach for Classification of Soil and Crop Prediction,” International Journal of Computer Science and Mobile Computing, vol. 7, no. 3, pp. 20-24, 2018.
[Google Scholar] [Publisher Link]
[26] R. Sujatha, and P. Isakki, “A Study on Crop Yield Forecasting Using Classification Techniques,” International Conference on Computing Technologies and Intelligent Data Engineering (ICCTIDE'16), 2016.
[CrossRef] [Google Scholar] [Publisher Link]
[27] Supriya D M, “Analysis of Soil Behavior and Prediction of Crop Yield using Data Mining Approach,” International Journal of Innovative Research in Computer and Communication Engineering, vol. 5, no. 5, pp. 9648-9653, 2017.
[Google Scholar] [Publisher Link]
[28] Akinbohun Folake, Akinbohun Ambrose, and Oyinloye Oghenerukevwe E., “Stacked Ensemble Model for Hepatitis in Healthcare System,” International Journal of Computer and Organization Trends, vol. 9, no. 4, pp. 25-29, 2019.
[CrossRef] [Publisher Link]
[29] Sumitra Nuanmeesri, and Lap Poomhiran, “Improved Classification of Intact Ripe Mango Sweetness using Fusion Deep Learning and Enhanced Near-Infrared Spectroscopy,” International Journal of Engineering Trends and Technology, vol. 70, no. 7, pp. 60-67, 2022.
[CrossRef] [Publisher Link]
[30] Jiawei Ma et al., “Design of an Artificial Assisted Fruit Picking Device,” International Journal of Computer and Organization Trends, vol. 10, no. 2, pp. 1-3, 2020. [CrossRef] [Publisher Link]
[31] TariebiKarikarisei, and Egbe Ebiyeritei Wisdom, “The Effect of Some Storage Systems on the Shelf Life of Avocado Pear (Persea Americana M.),” International Journal of Recent Engineering Science, vol. 10, no. 2, pp. 22-28, 2023.
[CrossRef] [Publisher Link]