Machine Learning based Predictive Assessments of Impacts of Influential Climatic Conditions for the Sustainable Productivity of Paddy Crops
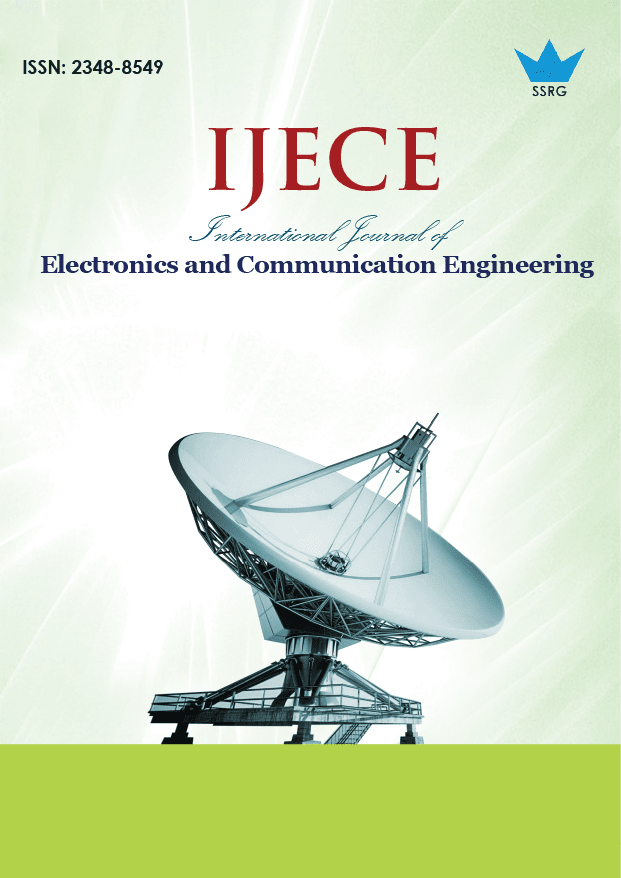
International Journal of Electronics and Communication Engineering |
© 2023 by SSRG - IJECE Journal |
Volume 10 Issue 5 |
Year of Publication : 2023 |
Authors : S. Thirumal, R. Latha |
How to Cite?
S. Thirumal, R. Latha, "Machine Learning based Predictive Assessments of Impacts of Influential Climatic Conditions for the Sustainable Productivity of Paddy Crops," SSRG International Journal of Electronics and Communication Engineering, vol. 10, no. 5, pp. 117-128, 2023. Crossref, https://doi.org/10.14445/23488549/IJECE-V10I5P111
Abstract:
The excessive inundation of paddy fields and the consumption of groundwater, fertilizers, and insecticides have resulted in substantial unintended consequences. The trajectory of the weather patterns and the correlation between weather and harvests will determine these effects' unique character and scale. Considering the essential environmental concerns affecting paddy ecosystems, this study aims to give a predictive evaluation and outline the ecological implications of rice production and the influence of a changing climate on paddy cultivation in the Region of Interest (RoI). In addition to these realities, it is essential to foresee the climate's effect on paddy fields by employing forward-thinking solutions for dealing with the ever-changing weather patterns that may directly impact rice yields. Philosophy-wise, Machine Learning (ML) differs from most traditional statistics since it emphasizes forecasting rather than description. In this paper, the case study centred on the findings of geographic climate models is conducted using multiplicative Long-Short Term Memory (mLSTM). mLSTM is employed to evaluate the consequences of climatic or environmental changes on rice production in RoI, showing the novelty of the work. To assess the effectiveness of the proposed methodology, the predicted outcomes are compared with the actual results. Sustainable rice production is assured through reasonable and actionable guidelines in the face of mounting environmental challenges.
Keywords:
Agriculture, Paddy crop, Predictive models, Machine learning, Crop productivity.
References:
[1] P. K. Aggarwal, and R. K. Mall, “Climate Change and Rice Yields in Diverse Agro-Environments of India. II. Effect of Uncertainties in Scenarios and Crop Models on Impact Assessment,” Climatic Change, vol. 52, no. 3, pp. 331-343, 2002.
[CrossRef] [Google Scholar] [Publisher Link]
[2] P. K. Aggarwal et al., “Simulating Genotypic Strategies for Increasing Rice Yield Potential In Irrigated, Tropical Environments,” Field Crops Research, vol. 51, no. 1–2, pp. 5–17, 1997.
[CrossRef] [Google Scholar] [Publisher Link]
[3] T. Akhtar, N. G. Haider, and S. M. Khan, “A Comparative Study of the Application of Glowworm Swarm Optimization Algorithm with other Nature-Inspired Algorithms in the Network Load Balancing Problem,” Engineering, Technology and Applied Science Research (ETASR), vol. 12, no. 4, pp. 8777–8784, 2022.
[CrossRef] [Google Scholar] [Publisher Link]
[4] A. R. Radhi, and A. M. Burhan, “Non-Profit Organization, Project Selection Process, Using the Hygiene Method of Multi-Criteria Decision Making,” Engineering, Technology and Applied Science Research (ETASR), vol. 12, no. 5, pp. 9097–9101, 2022.
[CrossRef] [Google Scholar] [Publisher Link]
[5] U. Tomer, and P. Gandhi, “An Enhanced Software Framework for Improving QoS in IoT,” Engineering, Technology and Applied Science Research (ETASR), vol. 12, no. 5, pp. 9172–9177, 2022.
[CrossRef] [Google Scholar] [Publisher Link]
[6] Prabhat Barnwal, and Koji Kotani, “Climatic Impacts Across Agricultural Crop Yield Distributions: An Application of Quantile Regression on Rice Crops in Andhra Pradesh, India,” Ecological Economics, vol. 87, pp. 95–109, 2013.
[CrossRef] [Google Scholar] [Publisher Link]
[7] Nathaniel Beck, and Jonathan N. Katz, “What To Do (and Not to Do) with Time-Series Cross-Section Data,” American Political Science Review, vol. 89, no. 3, pp. 634–647, 1995.
[CrossRef] [Google Scholar] [Publisher Link]
[8] Banu Bekci, “A Case Study on the Interdependence Between the Coastal Ecosystem and Humankind,” Ocean & Coastal Management, vol. 210, pp. 105666, 2021.
[CrossRef] [Google Scholar] [Publisher Link]
[9] K.Soundarya, and T.Pavithra, “An Efficient Under Water Communication Using Hybrid Low Energy Adaptive Clustering Hierarchy Protocol,” SSRG International Journal of Electronics and Communication Engineering, vol. 6, no. 6, pp. 10-14, 2019.
[CrossRef] [Publisher Link]
[10] Jacob Paul P et al., “IoT and ML Based Monitoring of Urban Wastewater System,” SSRG International Journal of Electronics and Communication Engineering, vol. 7, no. 6, pp. 17-22, 2020.
[CrossRef] [Google Scholar] [Publisher Link]
[11] Ahmed Elbeltagi et al., “The Impact of Climate Changes on the Water Footprint of Wheat and Maize Production in the Nile Delta, Egypt,” Science of the Total Environment, vol. 743, pp.140770, 2020.
[CrossRef] [Google Scholar] [Publisher Link]
[12] Yumiao Wang et al., “Combining Multi-Source Data and Machine Learning Approaches to Predict Winter Wheat Yield in the Conterminous United States,” Remote Sensing, vol. 12, no. 8, pp.1232, 2020.
[CrossRef] [Google Scholar] [Publisher Link]
[13] Hugo Carrão et al., “An Optimized System for the Classification of Meteorological Drought Intensity with Applications in Drought Frequency Analysis,” Journal of Applied Meteorology and Climatology, vol. 53, no. 8, pp. 1943–1960, 2014.
[CrossRef] [Google Scholar] [Publisher Link]
[14] A.J. Challinor et al., “Adaptation of Crops to Climate Change Through Genotypic Responses to Mean and Extreme Temperatures,” Agriculture, Ecosystems & Environment, vol. 119, no. 1–2, pp. 190–204, 2007.
[CrossRef] [Google Scholar] [Publisher Link]
[15] Chi-Chung Chen, Bruce A. McCarl, and David E. Schimmelpfennig, “Yield Variability as Influenced by Climate: A Statistical Investigation,” Climatic Change, vol. 66, pp. 239–261, 2004.
[CrossRef] [Google Scholar] [Publisher Link]
[16] M. Rehan Dastagir, “Modeling Recent Climate Change Induced Extreme Events in Bangladesh: A Review,” Weather and Climate Extremes, vol. 7, pp. 49–60, 2015.
[CrossRef] [Google Scholar] [Publisher Link]
[17] Kim Han-ryong et al., “Effects of Elevated CO2 Concentration and High Temperature on Growth and Yield on Rice. II. The Effect on Yield and Its Components of Akihikari Rice,” Journal of the Crop Science Society of Japan, vol. 65, no. 4, pp. 644–651, 1996.
[CrossRef] [Google Scholar] [Publisher Link]
[18] P. Droogers et al., “Adaptation Strategies to Climate Change to Sustain Food Security,” Climate Change in Contrasting River Basins: Adaptation Strategies for Water, Food and Environment, pp. 49–73, 2004.
[CrossRef] [Google Scholar] [Publisher Link]
[19] L. S. Hingane, K. Rupa Kumar, and Bh. V. Ramana Murty, “Long-Term Trends of Surface Air Temperature in India,” Journal of Climatology, vol. 5, no. 5, pp. 521–528, 1985.
[CrossRef] [Google Scholar] [Publisher Link]
[20] Murat Isik, and Stephen Devadoss, “An Analysis of the Impact of Climate Change on Crop Yields and Yield Variability,” Applied Economics, vol. 38, no. 7, pp. 835–844, 2007.
[CrossRef] [Google Scholar] [Publisher Link]
[21] T.G.F. Kittel, “The Vulnerability of Biodiversity to Rapid Climate Change,” Climate Vulnerability, vol. 4, pp. 185–201, 2013.
[CrossRef] [Google Scholar] [Publisher Link]
[22] Ben Krause et al., “Dynamic Evaluation of Neural Sequence Models,” Proceedings of the 35th International Conference on Machine Learning, pp. 2766-2775, 2018.
[CrossRef] [Publisher Link]
[23] K.S.Kavi Kumar, and Jyoti Parikh, “Indian Agriculture and Climate Sensitivity,” Global Environmental Change, vol. 11, no. 2, pp. 147–154, 2001.
[CrossRef] [Google Scholar] [Publisher Link]
[24] P. Krishnan et al., “Impact of Elevated Co2 and Temperature on Rice Yield and Methods of Adaptation as Evaluated by Crop Simulation Studies,” Agriculture, Ecosystems & Environment, vol. 122, no. 2, pp. 233–242, 2007.
[CrossRef] [Google Scholar] [Publisher Link]
[25] Yianna Lambrou, and Sibyl Nelson, “Gender Issues in Climate Change Adaptation: Farmers’ Food Security in Andhra Pradesh,” Research, Action and Policy: Addressing the Gendered Impacts of Climate Change, pp. 189–206, 2012.
[CrossRef] [Google Scholar] [Publisher Link]
[26] Franck Lecocq, and Zmarak Shalizi, “How Might Climate Change Affect Economic Growth in Developing Countries ? A Review of the Growth Literature with A Climate Lens,” Policy Research Working Papers, 2007.
[CrossRef] [Google Scholar] [Publisher Link]
[27] S. Thirumal, and R. Latha, “Teaching and Learning Based Optimization with Deep Learning Model for Rice Crop Yield Prediction,” SSRG International Journal of Electrical and Electronics Engineering, vol. 10, no. 4, pp. 105-114, 2023.
[CrossRef] [Publisher Link]
[28] Anuj Rapaka, and A. Clara Kanmani, “An Optimized Hyper Parameter Tuned Convolution Neural Frame for Potato Leaves Disease Prediction,” SSRG International Journal of Electrical and Electronics Engineering, vol. 10, no. 2, pp. 180-195, 2023.
[CrossRef] [Publisher Link]
[29] Vasanthakumary Selvanayagam, “A Statistical Analysis of Rainfall Variability and Paddy Production Trend in Batticaloa District, Sri Lanka,” SSRG International Journal of Agriculture & Environmental Science, vol. 5, no. 6, pp. 31-38, 2018.
[CrossRef] [Google Scholar] [Publisher Link]
[30] Atreya Paul, Amartya Pani, and Arup Bhandary, “Analysis the Pattern and Role of Crop Concentration and Diversification in Different Blocks of South 24 Pargana District, West Bengal,” SSRG International Journal of Agriculture & Environmental Science, vol. 7, no. 5, pp. 57-71, 2020.
[CrossRef] [Publisher Link]