A Review of Challenges and Solutions for Speech Quality Measurement in Low Bandwidth Sensor Networks
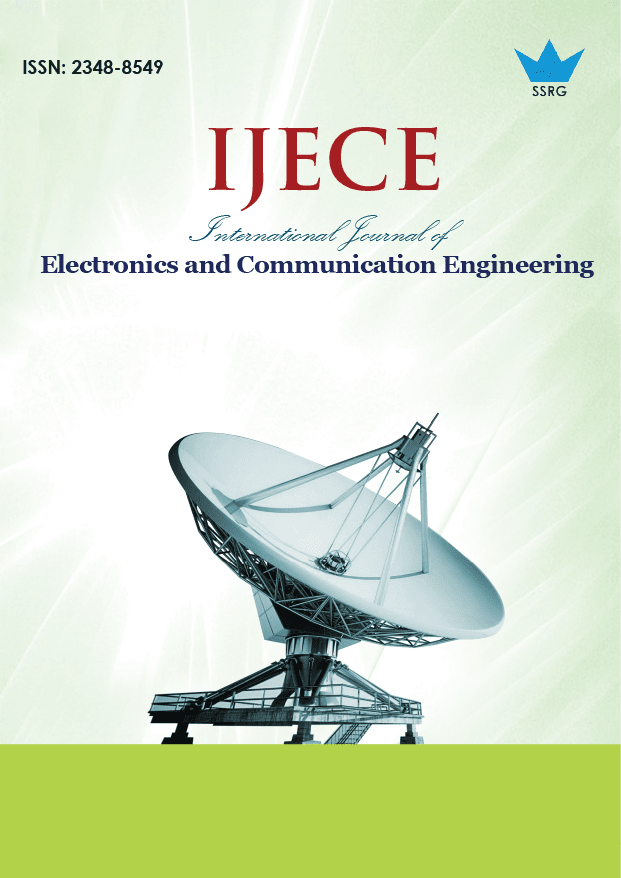
International Journal of Electronics and Communication Engineering |
© 2023 by SSRG - IJECE Journal |
Volume 10 Issue 5 |
Year of Publication : 2023 |
Authors : Vivekanand K Joshi, T. Kavitha |
How to Cite?
Vivekanand K Joshi, T. Kavitha, "A Review of Challenges and Solutions for Speech Quality Measurement in Low Bandwidth Sensor Networks," SSRG International Journal of Electronics and Communication Engineering, vol. 10, no. 5, pp. 149-159, 2023. Crossref, https://doi.org/10.14445/23488549/IJECE-V10I5P114
Abstract:
Radio communication has changed the face of communication. It is becoming superior to the landline telephone network. It is mainly famous for rigid voice communication with mobile and remote users using portable devices with good speech measurement quality. With the advent of technology, radio communication has shifted from analogue to digital domain. Low bandwidth Digital Radios are used by Professionals, Emergency service providers like Police and Firefighter to provide immediate, effective communication using portable devices at any remote place. These radios are operated in licensed frequency bands. They used analogue communication technology with sufficient bandwidth (25 KHZ). With this bandwidth, the speech quality in the communication was good. With the increasing demand for spectrum, frequency allocating authority decided to reduce bandwidth from 25 KHZ to 6.25 KHZ. Reducing bandwidth has affected many radios and sensor network parameters, ultimately lowering speech measurement quality. This paper analyses and summarizes those parameters and suggests possible methods for increasing speech measuring quality. It will help original equipment manufacturers, system planners, and engineers design the best possible combination of parameters for good speech quality in Digital Radio. The main affecting parameters are Technology which includes modulation and channel access techniques, Speech coding and quantization techniques, and planning and design of radio networks using Link Budget and Spoken language characteristics.
Keywords:
Bandwidth, Digital radio, Low bit rate codec, Speech quality, Sensor networks, Quantization.
References:
[1] T. APCO 25, “Project 25 FDMA – Common Air Interface New Technology Standards Project– Digital Radio Technical Standards”, TIA, 2003.
[Publisher Link]
[2] E. DMR1, “Electromagnetic compatibility and Radio spectrum Matters (ERM); Digital Mobile Radio (DMR) Systems” Part 1: DMR Air Interface (AI) protocol”, ETSI, 2006.
[Publisher Link]
[3] E. DMR2, “Electromagnetic Compatibility and Radio Spectrum Matters (ERM); Digital Mobile Radio (DMR) Systems- Part 2: DMR Voice and Generic Services and Facilities”, ETSI, 2007.
[Publisher Link]
[4] E. TETRA, “Terrestrial Trunked Radio (TETRA); Voice Plus Data (V+D); Part 1: General Network Design”, ETSI, 2009.
[Publisher Link]
[5] E. DMR-3, “Electromagnetic Compatibility and Radio Spectrum Matters (ERM); Digital Mobile Radio (DMR) Systems; Part 3: DMR Data Protocol”, ETSI, 2013.
[Publisher Link]
[6] E. NXDN, “NXDNTM Digital Protocol Accepted by the International Telecommunications Union-Radio Communications Sector (ITU-R)” JVCKENWOOD, 2017. [Publisher Link]
[7] E. DPMR, “Digital Private Mobile Radio (dPMR) using FDMA with a Channel Spacing of 6,25 kHz”, ETSI, 2019.
[Publisher Link]
[8] A. S. Spanias, “Speech Coding: A Tutorial Review,” Proceedings of the IEEE, vol. 82, no. 10, pp. 1541–1582, 1994.
[CrossRef] [Google Scholar] [Publisher Link]
[9] Gaby Abou Haidar, Roger Achkar; and Hassan Dourgham, “A Comparative Simulation Study of the Real Effect Of PCM, DM and DPCM Systems on Audio and Image Modulation,” IEEE International Multi-disciplinary Conference on Engineering Technology (IMCET), pp. 144–149, 2016.
[CrossRef] [Google Scholar] [Publisher Link]
[10] Muhanned Al-Rawi, and Muaayed Al-Rawi, “Comparison Between 40kb/s ADPCM Algorithms,” The Scientific Bulletin of Electrical Engineering Faculty, vol. 18, no. 2, pp. 34–36, 2018.
[CrossRef] [Google Scholar] [Publisher Link]
[11] Rakshith Ravishankar, Yu Bai, and Yoonsuk Choi, “Design and Implementation of 32-Channel ADPCM CODEC,” In IEEE 9th Annual Computing and Communication Workshop and Conference (CCWC), pp. 634–641, 2019.
[CrossRef] [Google Scholar] [Publisher Link]
[12] Gaous Afrizal, and Hendrawan, “Impact of Random and Burst Packet Loss on Voice Codec G. 711, G. 722, G. 729, AMR-NB, AMR-WB,” In 4th International Conference on Wireless and Telematics (ICWT), pp. 1–4, 2018.
[CrossRef] [Google Scholar] [Publisher Link]
[13] Lee, Bong-Ki, and Chang, Joon-Hyuk, “Online Steepest Descent Optimization of Muting Technique Parameters in ITU-T G. 722 Frame Erasure Concealment,” Acta Acustica united with Acustica, vol. 105, no. 2, pp. 343–349, 2019.
[CrossRef] [Google Scholar] [Publisher Link]
[14] Sala Surekha, and Md Zia Ur Rahman, “Cognitive Energy-Aware Spectrum Sensing with Improved Throughput for Medical Sensor Networks,” IEEE Sensors Letters, vol. 6, no. 6, pp. 1-4, 2022.
[CrossRef] [Google Scholar] [Publisher Link]
[15] J. Allen, “Applications of the Short Time Fourier Transform to Speech Processing and Spectral Analysis,” In ICASSP’82. IEEE International Conference on Acoustics, Speech, and Signal Processing, vol. 7, pp. 1012–1015, 1982.
[CrossRef] [Google Scholar] [Publisher Link]
[16] Raja Abdelmalek, Zied Mnasri, and Faouzi Benzarti, “Determining the Optimal Conditions for Signal Reconstruction Based on STFT Magnitude,” International Journal of Speech Technology, vol. 21, no. 3, pp. 619–632, 2018.
[CrossRef] [Google Scholar] [Publisher Link]
[17] Mahdi Parchami et al., “Recent Developments in Speech Enhancement in the Short-Time Fourier Transform Domain,” IEEE Circuits and Systems Magazine, vol. 16, no. 3, pp. 45–77, 2016.
[CrossRef] [Google Scholar] [Publisher Link]
[18] Sneha Das, Tom Bäckström, and Guillaume Fuchs, “Fundamental Frequency Model for Postfiltering at Low Bitrates in a Transform-Domain Speech and Audio Codec,” Proceedings Interspeech, pp. 2837–2841, 2020.
[CrossRef] [Google Scholar] [Publisher Link]
[19] Schuh, Florian et al., “Efficient Multichannel Audio Transform Coding with Low Delay and Complexity,” Audio Engineering Society, 2016.
[Google Scholar] [Publisher Link]
[20] Sala Surekha, and Md Zia Ur Rahman, “Spectrum Sensing and Allocation Strategy for IoT Devices Using Continuous-Time Markov Chain-Based Game Theory Model,” In IEEE Sensors Letters, vol. 6, no. 4, pp. 1-4, 2022.
[CrossRef] [Google Scholar] [Publisher Link]
[21] Zoran Peric et al., “Simple Speech Transform Coding Scheme Using Forward Adaptive Quantization for Discrete Input Signal,” Information Technology and Control, vol. 48, no. 3, pp. 454–463, 2019.
[CrossRef] [Google Scholar] [Publisher Link]
[22] Johannes Ballé et al., “Nonlinear Transform Coding,” arXiv preprint arXiv:2007.03034, 2020.
[CrossRef] [Google Scholar] [Publisher Link]
[23] Hatem Elaydi, Mustafa I. Jaber, and Mohammed B. Tanboura, “Speech Compression Using Wavelets,” 2003.
[Google Scholar] [Publisher Link]
[24] R. Zelinski, and P. Noll, “Adaptive Transform Coding Of Speech Signals,” IEEE Transactions on Acoustics, Speech, and Signal Processing, vol. 25, no. 4, pp. 299–309, 1977.
[CrossRef] [Google Scholar] [Publisher Link]
[25] Jagadish S.Jakati et al., “Discrete Wavelet Transform with Thresholding: An Effective Speech De-Noising Algorithm,” SSRG International Journal of Electrical and Electronics Engineering, vol. 10, no. 1, pp. 138-147, 2023.
[CrossRef] [Publisher Link]
[26] J. I. Agbinya, “Discrete Wavelet Transform Techniques in Speech Processing,” In Proceedings of Digi-tal Processing Applications (TENCON’96), vol. 2, pp. 514–519, 1996.
[CrossRef] [Google Scholar] [Publisher Link]
[27] D. S. P. Chips, “New DVSI AMBE+TM Vocoder: Toll-Quality Speech at four kbps” [Online]. Available : https://www.dvsinc.com/products/a20x0.shtml
[28] Sala Surekha, and Md Zia Ur Rahman, “Blockchain Framework for Cognitive Sensor Network Using Non-Cooperative Game Theory,” IEEE Access, vol. 10, pp. 60114-60127, 2022.
[CrossRef] [Google Scholar] [Publisher Link]
[29] L. M. Supplee et al., “MELP: The New Federal Standard At 2400 Bps,” IEEE International Conference on Acoustics, Speech, and Signal Processing, vol. 2, pp. 1591–1594, 1997.
[CrossRef] [Google Scholar] [Publisher Link]
[30] Zeng Runhua, and Zhang Shuqun, “Improving Speech Emotion Recognition Method of Convolutional Neural Network,” International Journal of Recent Engineering Science, vol. 5, no. 3, pp. 1-7, 2018.
[CrossRef] [Google Scholar] [Publisher Link]
[31] M. W. Chamberlain, “A 600 Bps MELP Vocoder for Use on HF channels,” MILCOM Proceedings Communications for Network-Centric Operations: Creating the Information Force (Cat. No. 01CH37277), vol. 1, pp. 447–453, 2001.
[CrossRef] [Google Scholar] [Publisher Link]
[32] Tian Wang et al., “A 1200/2400 Bps Coding Suite Based on MELP,” Speech Coding, IEEE Workshop Proceedings, pp. 90–92, 2002.
[CrossRef] [Google Scholar] [Publisher Link]
[33] Nagesh Mantravadi et al., “Spectrum Sensing using Energy Measurement in Wireless Telemetry Networks using Logarithmic Adaptive Learning,” Acta IMEKO, vol. 11, no. 1, pp. 1-7, 2022.
[CrossRef] [Google Scholar] [Publisher Link]
[34] S Sooraj, Ancy S Anselam, and Sakuntala S Pillai, “Performance Analysis of CELP Codec for Gaussian and Fixed Codebooks,” In International Conference on Communication Systems and Networks (ComNet), pp. 211–215, 2016.
[CrossRef] [Google Scholar] [Publisher Link]
[35] Chintalpudi S.L. Prasanna, and Md Zia Ur Rahman, “Noise Cancellation in Brain Waves Using a New Diffusion Normalized Least Power-Based Algorithm for Brain-Computer Interface Applications,” Measurement: Sensors, vol. 14, pp. 100038, 2021.
[CrossRef] [Google Scholar] [Publisher Link]
[36] Yu-Ting Lo et al., “A Pruned-CELP Speech Codec Using Denoising Autoencoder with Spectral Compensation for Quality and Intelligibility Enhancement,” In IEEE International Conference on Artificial Intelligence Circuits and Systems (AICAS), pp. 150–151, 2019.
[CrossRef] [Google Scholar] [Publisher Link]
[37] Md. Nizamuddin Salman, Polipalli Trinatha Rao, and Md. Zia Ur Rahman, “Novel Logarithmic Reference Free Adaptive Signal Enhancers for ECG Analysis of Wireless Health Care Monitoring Systems,” IEEE Access, vol. 6, pp. 46382-46395, 2018.
[CrossRef] [Google Scholar] [Publisher Link]
[38] Nikunj Tahilramani, and Ninad Bhatt, “Proposed Modifications in ITU-T G. 729 8 Kbps CS-Acelp Speech Codec and Its Overall Performance Analysis,” International Journal of Speech Technology, vol. 20, no. 3, pp. 615–628, 2017.
[CrossRef] [Google Scholar] [Publisher Link]
[39] Jyoshna, Girika, and Md Zia Ur Rahman, “An Intelligent Reference-Free Adaptive Learning Algorithm for Speech Enhancement,” Journal of Intelligent & Fuzzy Systems, vol. 42, no. 3, pp. 1895-1906, 2022.
[CrossRef] [Google Scholar] [Publisher Link]
[40] A. Ubale, and A. Gersho, “A Multiband CELP Wideband Speech Coder,” IEEE International Conference on Acoustics, Speech, and Signal Processing, vol. 2, pp. 1367–1370, 1997.
[CrossRef] [Google Scholar] [Publisher Link]
[41] G. Kim, “Wideband Speech Coding using CELP Algorithm,” PhD Thesis, Instytut Telekomunikacji, 2019.
[42] I. Soumya et al., “Efficient Block Processing of Long duration Biotelemetric Brain Data for Health Care Monitoring,” Review of Scientific Instruments, Vol. 86, pp.035003, 2015.
[CrossRef] [Google Scholar] [Publisher Link]
[43] J. P. Campbell, V. C. Welch, and T. E. Tremain, “An Expandable Error-Protected 4800 Bps CELP Coder (US Federal Standard 4800 Bps Voice Coder),” In International Conference on Acoustics, Speech, and Signal Processing, pp. 735–738, 1989.
[CrossRef] [Google Scholar] [Publisher Link]
[44] Gundlapalli Venkata Sai Karthik et al., “Efficient Signal Conditioning Techniques for Brain Activity in Remote Health Monitoring Network,” IEEE Sensors Journal, vol. 13, no. 9, pp. 3276-3283, 2013.
[CrossRef] [Google Scholar] [Publisher Link]
[45] S. Joshi, H. Purohit, and R. Choudhary, “A Simulation-Based Comparison on Code Excited Linear Prediction (CELP) Coder at Different Bit Rates,” In Proceedings of International Conference on Recent Advancement on Computer and Communication, 2018, pp. 297–304.
[CrossRef] [Google Scholar] [Publisher Link]
[46] Md. Zia Ur Rahman et al., “A Collateral Sensor Data Sharing Framework for Decentralized Healthcare Systems,” In IEEE Sensors Journal, vol. 21, no. 24, pp. 27848-27857, 2021.
[CrossRef] [Google Scholar] [Publisher Link]
[47] Hocine Chaouch, and Fatiha Merazka, “Multiple Description Coding and Forward Error Correction Concealment Methods for ACELP Coders in Packet Networks,” 6th International Conference on Image and Signal Processing and their Applications (ISPA), pp. 1–6, 2019.
[CrossRef] [Google Scholar] [Publisher Link]
[48] Bhawana Kumari et al., “An Efficient Algebraic Codebook Structure for CS-ACELP Based Speech Codecs,” 8th International Conference on Computing, Communication and Networking Technologies (ICCCNT), pp. 1–6, 2017.
[CrossRef] [Google Scholar] [Publisher Link]
[49] Heba Ahmed Elsayed et al., “CS-ACELP Speech Coding Simulink Modeling, Verification, and Optimized DSP Implementation on DSK 6713,” International Conference on Promising Electronic Technologies (ICPET), pp. 80–85, 2019.
[CrossRef] [Google Scholar] [Publisher Link]
[50] N. Kim, “Wideband LD-CELP Coder,” PhD Thesis, Instytut Telekomunikacji, 2019.
[51] Sala, Surekha, Md Zia Ur Rahman, and Navarun Gupta, “A Low Complex Spectrum Sensing Technique for Medical Telemetry System,” Journal of Scientific & Industrial Research, vol. 80, no. 5, pp. 449-456, 2021.
[CrossRef] [Google Scholar] [Publisher Link]
[52] T. Nomura et al., “A Bitrate and Bandwidth Scalable Celp Coder,” In Proceedings of the 1998 IEEE International Conference on Acoustics, Speech and Signal Processing, ICASSP’98 (Cat. No. 98CH36181), vol. 1, pp. 341–344, 1998.
[CrossRef] [Google Scholar] [Publisher Link]
[53] I. A. Gerson, and M. A. Jasiuk, “Vector Sum Excited Linear Prediction (VSELP) Speech Coding at 8 Kbps,” In International Conference on Acoustics, Speech, and Signal Processing, pp. 461–464, 1990.
[CrossRef] [Google Scholar] [Publisher Link]
[54] Kandarp K. Patel, and Mark L. Fowler, “Vector Quantizer Design for Speech Signal Compression,” In 7th International Conference on Cloud Computing, Data Science & Engineering-Confluence, pp. 744–749, 2017.
[CrossRef] [Google Scholar] [Publisher Link]
[55] Karush Suri, “Sub - Band Coding and Speech Quality Testing,” SSRG International Journal of Electronics and Communication Engineering, vol. 3, no. 1, pp. 10-13, 2016.
[CrossRef] [Publisher Link]
[56] M. Shafi V. Makwana, A. B. Nandurbarkar, and K. R. Parmar, “Speech Compression Using Tree-Structured Vector Quantization,” 2nd International Conference on Devices, Circuits and Systems (ICDCS), pp. 1–4, 2014.
[CrossRef] [Google Scholar] [Publisher Link]
[57] Kai Xiang, and Ruimin Hu, “Low Bitrates Audio Coding Using Lattice Vector Quantization Based on Scalable and High Order Codebook Extension Scheme,” International Conference on Wireless Communications & Signal Processing (WCSP), pp. 1–4, 2010.
[CrossRef] [Google Scholar] [Publisher Link]
[58] J. Foster, R. Gray, and M. Dunham, “Finite-State Vector Quantization for Waveform Coding,” IEEE Transactions on Information Theory, vol. 31, no. 3, pp. 348–359, 1985.
[CrossRef] [Google Scholar] [Publisher Link]
[59] N. M. Nasrabadi, and Y. Feng, “A Dynamic Finite-State Vector Quantization Scheme,” In International Conference on Acoustics, Speech, and Signal Processing, vol. 4, pp. 2261–2264, 1990.
[CrossRef] [Google Scholar] [Publisher Link]
[60] F.G.B. De Natale, S. Fioravanti, and D. D. Giusto, “DCRVQ: A New Strategy for Efficient Entropy Coding of Vector-Quantized Images,” IEEE Transactions on Communications, vol. 44, no. 6, pp. 696–706, 1996.
[CrossRef] [Google Scholar] [Publisher Link]
[61] Guobin Shen, and M. L. Liou, “An Efficient Codebook Post-Processing Technique and A Window-Based Fast-Search Algorithm for Image Vector Quantization,” IEEE Transactions on Circuits and Systems for Video Technology, vol. 10, no. 6, pp. 990–997, 2000.
[CrossRef] [Google Scholar] [Publisher Link]
[62] V. Krishnan, “A framework for low bitrate speech coding in noisy environment,” PhD Thesis, Georgia Institute of Technology, 2005. [Online]. Available : https://repository.gatech.edu/home.
[63] Cheu Meh Chu, and David V. Anderson, “Likelihood Codebook Reordering Vector Quantization,” IEEE International Conference on Acoustics, Speech and Signal Processing, pp. 5114–5117, 2013.
[CrossRef] [Google Scholar] [Publisher Link]
[64] P. R. Kanawade, and S. S. Gundal, “Tree-Structured Vector Quantization Based Technique for Speech Compression,” International Conference on Data Management, Analytics and Innovation (ICDMAI), pp. 274–279, 2017.
[CrossRef] [Google Scholar] [Publisher Link]
[65] H.-R. Vocoder, “DVSI Vocoder Independent Evaluation Results”. [Online]. Available:
https://www.dvsinc.com/papers/eval_results.html.
[66] B. Durai Babu et al., “Transformer Health Monitoring System using GSM Technology,” International Journal of Electronics and Communication Engineering, vol. 9, no.11, pp.11-16, 2022.
[CrossRef][Publisher Link]
[67] S Sakthi Vel, D. Muhammad Noorul Mubarak, and S Aji, “A study on Vowel Duration in Tamil: Instrumental Approach,” IEEE International Conference on Computational Intelligence and Computing Research (ICCIC), pp. 1–4, 2015.
[CrossRef] [Google Scholar] [Publisher Link]
[68] Kimiko Tsukada, “An Acoustic Comparison of Vowel Length Contrasts in Arabic, Japanese and Thai: Durational and Spectral Data,” International Journal on Asian Language Processing, vol. 19, no. 4, pp. 127–138, 2009.
[CrossRef] [Publisher Link]
[69] Pardeep Singh, and Kamlesh Dutta, “Formant Analysis of Punjabi Non-Nasalized Vowel Phonemes,” International Conference on Computational Intelligence and Communication Networks, pp. 375–380, 2011.
[CrossRef] [Google Scholar] [Publisher Link]
[70] S. Suma Christal Mary et al., “Data Security in Wireless Sensor Networks using an Efficient Cryptographic Technique to Protect Against Intrusion,” International Journal of Electronics and Communication Engineering, vol. 10, no.4, pp.41-50, 2023.
[CrossRef] [Publisher Link]
[71] Zhou Xuewen et al., “Acoustic Feature and Variance of Uigur Vowels,” International Conference on Speech Database and Assessments (Oriental COCOSDA), pp. 180–184, 2011.
[CrossRef] [Google Scholar] [Publisher Link]
[72] J. R. Sawusch, “Effects of Duration and Formant Movement on Vowel Perception,” In Proceeding of Fourth International Conference on Spoken Language Processing. ICSLP’96, vol. 4, pp. 2482–2485, 1996.
[CrossRef] [Google Scholar] [Publisher Link]
[73] András Kalapos et al., “Vision-Based Reinforcement Learning for Lane-Tracking Control,” ACTA IMEKO, vol. 10, no.3, pp. 1-8, 2021.
[CrossRef] [Google Scholar] [Publisher Link]
[74] Eulalia Balestrieri et al., “A Review of Accurate Phase Measurement Methods and Instruments for Sinewave Signals,” ACTA IMEKO, vol. 9, no.2, pp. 1-7, 2021.
[CrossRef] [Google Scholar] [Publisher Link]
[75] Lorenzo Ciani et al., “A Hybrid Tree Sensor Network for A Condition Monitoring System to Optimise Maintenance Policy,” ACTA IMEKO, vol. 9, no.1, pp. 3-9, 2020.
[CrossRef] [Google Scholar] [Publisher Link]
[76] S. Surekha, and Md Zia Ur Rahman, “Spectrum Sensing for Wireless Medical Telemetry Systems Using a Bias Compensated Normalized Adaptive Algorithm,” International Journal of Microwave and Optical Technology, vol. 16, no. 2, pp. 124-133, 2021.
[Google Scholar] [Publisher Link]
[77] K. Murali, and S. SivaPerumal, “Sector Multi-Beam Space Optimal Bit Error Rate Enhancement in Wireless 5G Using Power Domain NOMA,” Soft Computing, vol. 27, pp. 537-545, 2023.
[CrossRef] [Google Scholar] [Publisher Link]