Forecasting the Factors Responsible for Improving the Yield of Sugarcane Crop using Artificial Neural Network
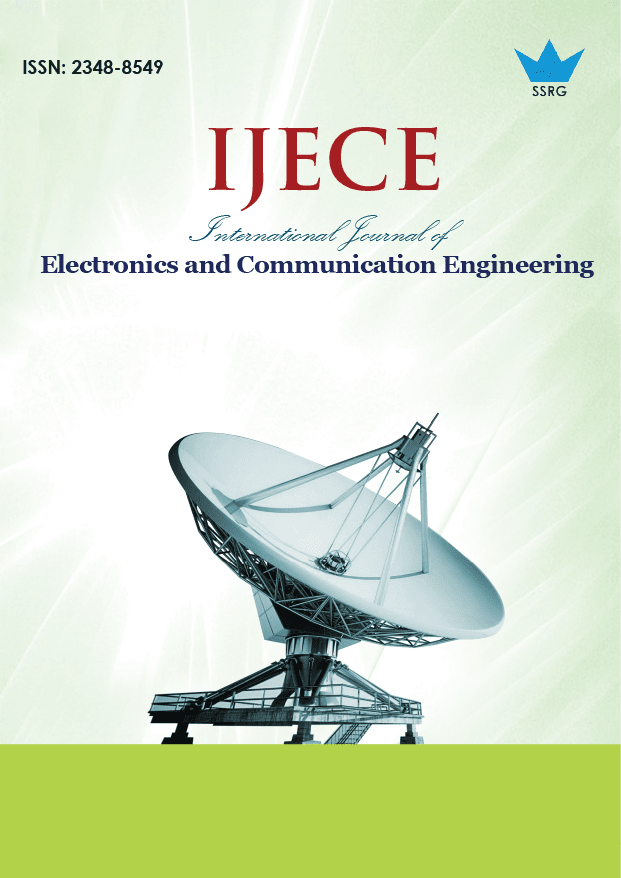
International Journal of Electronics and Communication Engineering |
© 2023 by SSRG - IJECE Journal |
Volume 10 Issue 7 |
Year of Publication : 2023 |
Authors : S. Muthukumaran, N. Gnanasankaran, Sandhya Soman, G. Rakesh |
How to Cite?
S. Muthukumaran, N. Gnanasankaran, Sandhya Soman, G. Rakesh, "Forecasting the Factors Responsible for Improving the Yield of Sugarcane Crop using Artificial Neural Network," SSRG International Journal of Electronics and Communication Engineering, vol. 10, no. 7, pp. 33-43, 2023. Crossref, https://doi.org/10.14445/23488549/IJECE-V10I7P104
Abstract:
Sugarcane is a cash crop cultivated in India's Tropical and Sub Tropical regions, contributing 5.7% of the Gross Domestic Product (GDP) to the Indian Economy. Sugarcane Farming gives employment opportunities to 60 million rural families. It is cultivated all over India to a latitude of 80◦ N to 300◦ N. Sugarcane contains sucrose in its stem juice and is the primary raw material for producing sugar. Sugarcane crop production depends on the season, biological, and economic cause. The growing demand for sugarcane worldwide incorporates the backbone of sugarcane agriculture. This paper proposed A Hybrid Machine Learning Model (HMLM) for forecasting the sugarcane yield, which helps both farmers and the sugar mills to assist in annual planning. The proposed model used Backward Feature Elimination to select the factors that dominate sugarcane production. K-Means Clustering (K-MC) is applied to the selected attributes, and the dataset is partitioned. An Artificial Neural Network (ANN) is created for each clustered dataset, and the parameters influencing sugarcane production are found. The performance of each Neural Network (NN) created was analysed by implementing performance measures, and the findings were compiled. The information gained from the suggested model aids farmers in making decisions to increase sugarcane crop productivity.
Keywords:
Artificial Neural Network, Backward feature elimination, K-means clustering, Machine learning, Sugarcane production.
References:
[1] Mohammad Hajeb et al., “Simultaneous Retrieval of Sugarcane Variables from Sentinel-2 Data using Bayesian Regularized Neural Network,” International Journal of Applied Earth Observation and Geoinformation, vol. 116, p. 103168, 2023.
[CrossRef] [Google Scholar] [Publisher Link]
[2] Athiraja Atheeswaran et al., “Expert System for Smart Farming for Diagnosing Sugarcane Diseases using Machine Learning,” Computers and Electrical Engineering, vol. 109, p. 108739, 2023.
[CrossRef] [Google Scholar] [Publisher Link]
[3] Anuj Rapaka, and A. Clara Kanmani, “An Optimized Hyper Parameter Tuned Convolution Neural Frame for Potato Leaves Disease Prediction,” SSRG International Journal of Electrical and Electronics Engineering, vol. 10, no. 2, pp. 180-195, 2023.
[CrossRef] [Publisher Link]
[4] S. K. Tyagi et al., “Technological Advancements in Jaggery-Making Processes and Emission Reduction Potential via Clean Combustion for Sustainable Jaggery Production: An Overview,” Journal of Environmental Management, vol. 301, p. 113792, 2022.
[CrossRef] [Google Scholar] [Publisher Link]
[5] Mahima Begum, Dhiman Dev Singha, and Bijnan Chandra Bordoloi, “Trend of Sugarcane and Jaggery Production in Assam and Associated Problems and Prospects,” SSRG International Journal of Agriculture & Environmental Science, vol. 3, no. 6, pp. 13-20, 2016.
[CrossRef] [Google Scholar] [Publisher Link]
[6] A. Narayanamoorthy, The Irrigation Future of India: Overview and Synthesis, Global Issues in Water Policy, vol. 29, pp. 1-23, 2022.
[CrossRef] [Google Scholar] [Publisher Link]
[7] Shyamal S. Virnodkar et al., “CaneSat Dataset to Leverage Convolutional Neural Networks for Sugarcane Classification from Sentinel2,” Journal of King Saud University-Computer and Information Sciences, vol. 34, no. 6, pp. 3343-3355, 2020.
[CrossRef] [Google Scholar] [Publisher Link]
[8] Marife Kung Villareal, and Alejandro Fernandez Tongco, “Sugarcane Classification using Spectral Signature and Object-Based Image Analysis (OBIA) in LiDAR Data Sets,” SSRG International Journal of Agriculture & Environmental Science, vol. 6, no. 4, pp. 9-16, 2019.
[CrossRef] [Google Scholar] [Publisher Link]
[9] Ali Kaab et al., “Combined Life Cycle Assessment and Artificial Intelligence for Prediction of Output Energy and Environmental Impacts of Sugarcane Production,” Science of the Total Environment, vol. 664, pp. 1005-1019, 2019.
[CrossRef] [Google Scholar] [Publisher Link]
[10] Ana Cláudia dos Santos Luciano et al., “Empirical Model for Forecasting Sugarcane Yield on a Local Scale in Brazil using Landsat Imagery and Random Forest Algorithm,” Computers and Electronics in Agriculture, vol. 184, p. 106063, 2021.
[CrossRef] [Google Scholar] [Publisher Link]
[11] Ate Poortinga et al., “Mapping Sugarcane in Thailand using Transfer Learning, a Lightweight Convolutional Neural Network, NICFI High Resolution Satellite Imagery and Google Earth Engine,” ISPRS Open Journal of Photogrammetry and Remote Sensing, vol. 1, p. 100003, 2021.
[CrossRef] [Google Scholar] [Publisher Link]
[12] Lidan Meng et al., “Understanding the Pathways for Irreversible Aggregate Clusters Formation in Concentrated Sugarcane Juice Derived from the Membrane Clarification Process,” LWT, vol. 151, p.112204, 2021.
[CrossRef] [Google Scholar] [Publisher Link]
[13] Pengwen Wang, Behzad Aalipur Hafshejani, and Daluyo Wang, “An Improved Multilayer Perceptron Approach for Detecting Sugarcane Yield Production in IoT-Based Smart Agriculture,” Microprocessors and Microsystems, vol. 82, p. 103822, 2021.
[CrossRef] [Google Scholar] [Publisher Link]
[14] Anteneh Agezew Melash et al., “Indigenous Agricultural Knowledge: A Neglected Human Based Resource for Sustainable Crop Protection and Production,” Heliyon, vol. 9, no. 1, pp. 1-9, 2023.
[CrossRef] [Google Scholar] [Publisher Link]
[15] S. Divya Meena et al., "Crop Yield Improvement with Weeds, Pest and Disease Detection,” Procedia Computer Science, vol. 218, pp. 2369- 2382, 2023.
[CrossRef] [Google Scholar] [Publisher Link]
[16] S. Thirumal, and R. Latha, “Teaching and Learning based Optimization with Deep Learning Model for Rice Crop Yield Prediction,” SSRG International Journal of Electrical and Electronics Engineering, vol. 10, no. 4, pp. 105-114, 2023.
[CrossRef] [Publisher Link]
[17] D. Sheema et al., “The Detection and Identification of Pest-FAW Infestation in Maize Crops using Iot-Based Deep-Learning Algorithm,” SSRG International Journal of Electrical and Electronics Engineering, vol. 9, no. 12, pp. 180-188, 2022.
[CrossRef] [Publisher Link]
[18] Yongqiang Wang et al., “Multiobjective Optimization of Regional Irrigation and Nitrogen Schedules using the CERES-Maize Model with Crop Parameters Determined from the Remotely Sensed Leaf Area Index,” Agricultural Water Management, vol. 286, p. 108386, 2023.
[CrossRef] [Google Scholar] [Publisher Link]
[19] K. Archana, and K. G. Saranya, “Crop Yield Prediction, Forecasting and Fertilizer Recommendation using Voting Based Ensemble Classifier,” SSRG International Journal of Computer Science and Engineering, vol. 7, no. 5, pp. 1-4, 2020.
[CrossRef] [Google Scholar] [Publisher Link]
[20] Jasmin Praful Bharadiya, Nikolaos Tzenios Tzenios, and Manjunath Reddy, “Forecasting of Crop Yield using Remote Sensing Data, Agrarian Factors and Machine Learning Approaches,” Journal of Engineering Research and Reports, vol. 24, no. 12, pp. 29-44, 2023.
[CrossRef] [Google Scholar] [Publisher Link]
[21] Ryoya Tanabe, Tsutomu Matsui, and Takashi S T Tanaka, “Winter Wheat Yield Prediction using Convolutional Neural Networks and UAV-Based Multispectral Imagery,” Field Crops Research, vol. 291, p. 108786, 2023.
[CrossRef] [Google Scholar] [Publisher Link]