A Comparative Study of DDoS Attack in Cloud Computing Environment
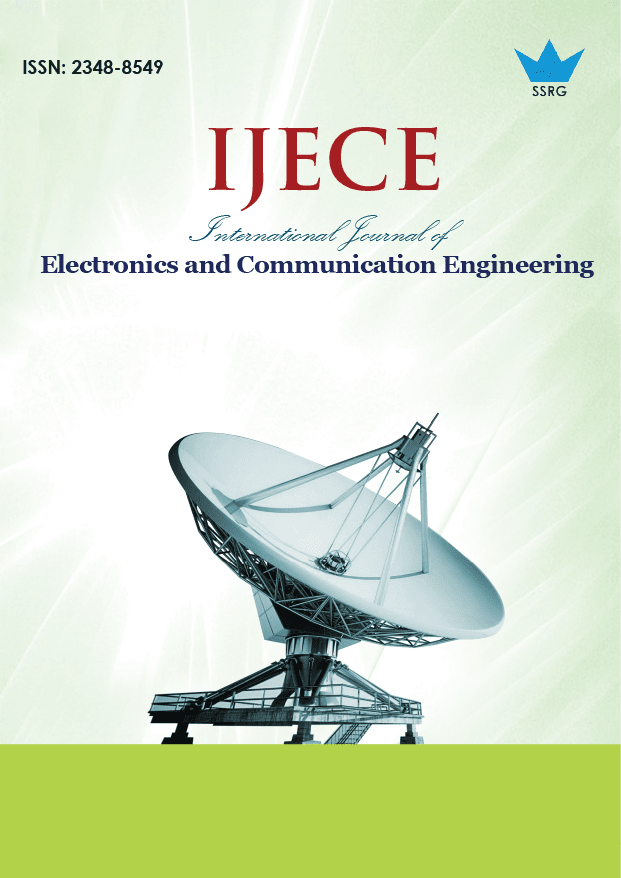
International Journal of Electronics and Communication Engineering |
© 2023 by SSRG - IJECE Journal |
Volume 10 Issue 7 |
Year of Publication : 2023 |
Authors : Animesh Kumar, Sandip Dutta, Prashant Pranav |
How to Cite?
Animesh Kumar, Sandip Dutta, Prashant Pranav, "A Comparative Study of DDoS Attack in Cloud Computing Environment," SSRG International Journal of Electronics and Communication Engineering, vol. 10, no. 7, pp. 87-96, 2023. Crossref, https://doi.org/10.14445/23488549/IJECE-V10I7P109
Abstract:
DDoS Attack refers to the flooding of the server through various mechanisms by the attackers to devoid the user of having access to the resources or to deplete the user's available resources. DDoS attack in the cloud has been one of the most frequent attacks in the service, eventually hampering the provider's and users' economic and resource availability. This paper categorized the DDoS research papers based on Network Management, Deep Learning Methods, Machine Learning, SoftwareDefined Networks, Resource Management, Load Distribution, Fuzzy Approach, etc. Accuracy, Precision, Recall, and F1 Score are compared with forty-one different proposed methods, and comparative graphs are also shown. SaDE-ELM performs best in all datasets, and SVM performs worst.
Keywords:
Cloud attack, Cloud computing, Deep learning, Machine learning, Security issues.
References:
[1] Anshuman Singh, and Brij B Gupta, “Distributed Denial-of-Service (DDoS) Attacks and Defense Mechanisms in Various Web-Enabled Computing Platforms: Issues, Challenges, and Future Research Directions,” International Journal on Semantic Web and Information Systems (IJSWIS), vol. 18, no. 1, pp. 1-43, 2022.
[CrossRef] [Google Scholar] [Publisher Link]
[2] Taher Labidi et al., “On the OLS Regression Algorithm and Pearson Correlation Algorithm for Improving the SLA Establishment Process in Cloud Computing,” Innovations in Systems and Software Engineering, vol. 18, pp. 215–229, 2022.
[CrossRef] [Google Scholar] [Publisher Link]
[3] Gaurav Somani et al., “Combating DDoS Attacks in the Cloud: Requirements, Trends, and Future Directions,” IEEE Cloud Computing, vol. 4, no. 1, pp. 22-32, 2017.
[CrossRef] [Google Scholar] [Publisher Link]
[4] Zhaomin Chen et al., “Power Spectrum Entropy-Based Detection and Mitigation of Low-Rate DoS Attacks,” Computer Networks, vol. 136, pp. 80-94, 2018.
[CrossRef] [Google Scholar] [Publisher Link]
[5] Sandeep Kautish, Reyana A, and Ankit Vidyarthi, “SDMTA: Attack Detection and Mitigation Mechanism for DDoS Vulnerabilities in Hybrid Cloud Environment,” IEEE Transactions on Industrial Informatics, vol. 18, no. 9, pp. 6455-6463, 2022.
[CrossRef] [Google Scholar] [Publisher Link]
[6] Muhammad Nadeem et al., “Intercept the Cloud Network from Brute Force and DDoS Attacks via Intrusion Detection and Prevention System,” IEEE Access, vol. 9, pp. 152300-152309, 2021.
[CrossRef] [Google Scholar] [Publisher Link]
[7] Divyasree I R, and Selvamani K, “DAD: Domain Adversarial Defense System against DDoS Attacks in Cloud,” IEEE Transactions on Network and Service Management, vol. 19, no. 1, pp. 554-568, 2022.
[CrossRef] [Google Scholar] [Publisher Link]
[8] Komal Purba, and Nitin Bhagat, “A Review on Load Balancing Algorithm in Cloud Computing,” SSRG International Journal of Computer Science and Engineering, vol. 1, no. 10, pp. 1-5, 2014.
[Google Scholar] [Publisher Link]
[9] Aanshi Bhardwaj, Veenu Mangat, and Renu Vig, “Hyperband Tuned Deep Neural Network with Well Posed Stacked Sparse Auto Encoder for Detection of DDoS Attacks in Cloud,” IEEE Access, vol. 8, pp. 181916-181929, 2020.
[CrossRef] [Google Scholar] [Publisher Link]
[10] Ilhan Firat Kilincer, Fatih Ertam, and Abdulkadir Sengur, “Machine Learning Methods for Cyber Security Intrusion Detection: Datasets and Comparative Study,” Computer Networks, vol. 188, p.107840, 2021.
[CrossRef] [Google Scholar] [Publisher Link]
[11] Gerry Saporito, A Deeper Dive into the NSL-KDD Data Set, 2020. [Online]. Available: https://towardsdatascience.com/a-deeper-dive-intothe-nsl-kdd-data-set-15c753364657
[12] Gopal Singh Kushwah, and Virender Ranga, “Detecting DDoS Attacks in Cloud Computing using Extreme Learning Machine and Adaptive Differential Evolution,” Wireless Personal Communications, vol. 124, pp. 2613-2636, 2022.
[CrossRef] [Google Scholar] [Publisher Link]
[13] Guang-Bin Huang, Qin-Yu Zhu, and Chee-Kheong Siew, “Extreme Learning Machine: A New Learning Scheme of Feedforward Neural Networks,” 2004 IEEE International Joint Conference on Neural Networks (IEEE Cat. No.04CH37541), Budapest, Hungary, pp. 985- 990, 2004.
[CrossRef] [Google Scholar] [Publisher Link]
[14] Shifei Ding et al., “Extreme Learning Machine: Algorithm, Theory and Applications,” Artificial Intelligence Review, vol. 44, pp. 103- 115, 2015.
[CrossRef] [Google Scholar] [Publisher Link]
[15] A. K. Qin, and P. N. Suganthan, “Self-Adaptive Differential Evolution Algorithm for Numerical Optimization,” 2005 IEEE Congress on Evolutionary Computation, Edinburgh, UK, pp. 1785-1791, 2005.
[CrossRef] [Google Scholar] [Publisher Link]
[16] Ahmed Abdullah Alqarni, “Majority Vote-Based Ensemble Approach for Distributed Denial of Service Attack Detection in Cloud Computing,” Journal of Cyber Security and Mobility, vol. 11, no. 2, pp. 265-278, 2022.
[CrossRef] [Google Scholar] [Publisher Link]
[17] Jianhua Liu et al., “A Bayesian Q-Learning Game for Dependable Task Offloading Against DDoS Attacks in Sensor Edge Cloud,” IEEE Internet of Things Journal, vol. 8, no. 9, pp. 7546-7561, 2021.
[CrossRef] [Google Scholar] [Publisher Link]
[18] Zhi Li et al., “Exploring New Opportunities to Defeat Low-Rate DDoS Attack in Container-Based Cloud Environment,” IEEE Transactions on Parallel and Distributed Systems, vol. 31, no. 3, pp. 695-706, 2020.
[CrossRef] [Google Scholar] [Publisher Link]
[19] Marwane Zekri et al., “DDoS Attack Detection using Machine Learning Techniques in Cloud Computing Environments,” 2017 3rd International Conference of Cloud Computing Technologies and Applications (CloudTech), Rabat, Morocco, pp. 1-7, 2017.
[CrossRef] [Google Scholar] [Publisher Link]
[20] Aqeel Sahi et al., “An Efficient DDoS TCP Flood Attack Detection and Prevention System in a Cloud Environment,” IEEE Access, vol. 5, pp. 6036-6048, 2017.
[CrossRef] [Google Scholar] [Publisher Link]
[21] Hoang Lan Vu et al., “Analysis of Input Set Characteristics and Variances on K-Fold Cross-Validation for a Recurrent Neural Network Model on Waste Disposal Rate Estimation,” Journal of Environmental Management, vol. 311, p. 114869, 2022.
[CrossRef] [Google Scholar] [Publisher Link]
[22] Pillutla Harikrishna, and A. Amuthan, “Rival-Model Penalized Self-Organizing Map Enforced a DDoS Attack Prevention Mechanism for a Software-Defined Network-Based Cloud Computing Environment,” Journal of Parallel and Distributed Computing, vol. 154, pp. 142-152, 2021.
[CrossRef] [Google Scholar] [Publisher Link]
[23] N. Priyanka, and V. Vetriselvi, “Penetration Testing for Software Defined Networks against DOS Attack,” SSRG International Journal of Computer Science and Engineering, vol. 3, no. 8, pp. 10-13, 2016.
[CrossRef] [Google Scholar] [Publisher Link]
[24] Saptarshi Debroy et al., “Frequency-Minimal Utility-Maximal Moving Target Defense against DDoS in SDN-Based Systems,” IEEE Transactions on Network and Service Management, vol. 17, no. 2, pp. 890-903, 2020.
[CrossRef] [Google Scholar] [Publisher Link]
[25] Xenia Mountrouidou, and Vic Thomas, “CyberPaths: Cyber Security Labs for Liberal Arts Institutions using the NSF Global Environment for Network Innovations (GENI),” SIGCSE’19:Proceedings of the 50th ACM Technical Symposium on Computer Science Education, p. 1241, 2019.
[CrossRef] [Google Scholar] [Publisher Link]
[26] Trung V Phan, and Minho Park, “Efficient Distributed Denial-of-Service Attack Defense in SDN-Based Cloud,” IEEE Access, vol. 7, pp. 18701-18714, 2019.
[CrossRef] [Google Scholar] [Publisher Link]
[27] V. Gholami et al., “Comparison of Self-Organizing Map, Artificial Neural Network, and Co-Active Neuro-Fuzzy Inference System Methods in Simulating Groundwater Quality: Geospatial Artificial Intelligence,” Water Resources Management, vol. 36, pp. 451-469, 2022.
[CrossRef] [Google Scholar] [Publisher Link]
[28] Bin Yuan et al., “Minimizing Financial Cost of DDoS Attack Defense in Clouds with Fine-Grained Resource Management,” IEEE Transactions on Network Science and Engineering, vol. 7, no. 4, pp. 2541-2554, 2020.
[CrossRef] [Google Scholar] [Publisher Link]
[29] Gaurav Somani et al., “Scale Inside-Out: Rapid Mitigation of Cloud DDoS Attacks,” IEEE Transactions on Dependable and Secure Computing, vol. 15, no. 6, pp. 959-973, 2018.
[CrossRef] [Google Scholar] [Publisher Link]
[30] Omar Abdel Wahab et al., “Optimal Load Distribution for the Detection of VM-Based DDoS Attacks in the Cloud,” IEEE Transactions on Services Computing, vol. 13, no. 1, pp. 114-129, 2020.
[CrossRef] [Google Scholar] [Publisher Link]
[31] Mohamed Hadi Habaebi et al., “Extending CloudSim to Simulate Sensor Networks,” Simulation, vol. 99, no. 1, pp. 3-22, 2022.
[CrossRef] [Google Scholar] [Publisher Link]
[32] Fei Zhang et al., “A Survey on Virtual Machine Migration: Challenges, Techniques, and Open Issues,” IEEE Communications Surveys & Tutorials, vol. 20, no. 2, pp. 1206-1243, 2018.
[CrossRef] [Google Scholar] [Publisher Link]
[33] Mahesh M Baradkar, and Bandu B Meshram, “A Survey on Cloud Security: Infrastructure as a Service,” SSRG International Journal of Computer Science and Engineering, vol. 6, no. 6, pp. 17-21, 2019.
[CrossRef] [Google Scholar] [Publisher Link]
[34] N. Sendhil Kumar, and G. Jyotheeswar Chowdary, “IAAS Based Cloud Security and a Deep View Trust Model,” SSRG International Journal of Computer Science and Engineering, vol. 3, no. 4, pp. 28-33, 2016.
[Publisher Link]
[35] Naela Rizvi, and Dharavath Ramesh, “FBQ-LA: Fuzzy Based Q-Learning Approach for Elastic Workloads in Cloud Environment,” Journal of Intelligent & Fuzzy Systems, vol. 36, no. 3, pp. 2715-2728, 2019.
[CrossRef] [Google Scholar] [Publisher Link]
[36] P. J. Beslin Pajila, E. Golden Julie, and Y. Harold Robinson, “FBDR-Fuzzy Based DDoS Attack Detection and Recovery Mechanism for Wireless Sensor Networks,” Wireless Personal Communications, vol. 122, pp. 3053-3083, 2022.
[CrossRef] [Google Scholar] [Publisher Link]
[37] Miguel Vivas-Cortez et al., “On some Generalized Raina-Type Fractional-Order Integral Operators and Related Chebyshev Inequalities,” AIMS Mathematics, vol. 7, no. 6, pp. 10256-10275, 2022.
[CrossRef] [Google Scholar] [Publisher Link]
[38] Yajie Li et al., “DDoS Attack Mitigation Based on Traffic Scheduling in Edge Computing- Enabled TWDM-PON,” IEEE Access, vol. 9, pp. 166566-166578, 2021.
[CrossRef] [Google Scholar] [Publisher Link]
[39] Nagarathna Ravi, and S. Mercy Shalinie, “Learning-Driven Detection and Mitigation of DDoS Attacks in IoT via SDN-Cloud Architecture,” IEEE Internet of Things Journal, vol. 7, no. 4, pp. 3559-3570, 2020.
[CrossRef] [Google Scholar] [Publisher Link]
[40] I. Lakshmi, “Security Analysis in Internet of Things using DDoS Mechanisms,” SSRG International Journal of Mobile Computing and Application, vol. 6, no. 1, pp. 19-24, 2019.
[CrossRef] [Publisher Link]
[41] Adil Bin Bhutto et al., “Reinforced Transformer Learning for VSI-DDoS Detection in Edge Clouds,” IEEE Access, vol. 10, pp. 94677- 94690, 2022.
[CrossRef] [Google Scholar] [Publisher Link]
[42] S. Velliangiri, and Hari Mohan Pandey, “Fuzzy-Taylor-Elephant Herd Optimization Inspired Deep Belief Network for DDoS Attack Detection and Comparison with State-of-the-Art Algorithms,” Future Generation Computer Systems, vol. 110, pp. 80-90, 2020.
[CrossRef] [Google Scholar] [Publisher Link]
[43] Damai Jessica Prathyusha, and Govinda Kannayaram, “A Cognitive Mechanism for Mitigating DDoS Attacks using the Artificial Immune System in a Cloud Environment,” Evolutionary Intelligence, vol. 14, pp. 607-618, 2021.
[CrossRef] [Google Scholar] [Publisher Link]