IoT-Driven Image Processing Framework for Accurate Skin Diseases Diagnosis and Classification
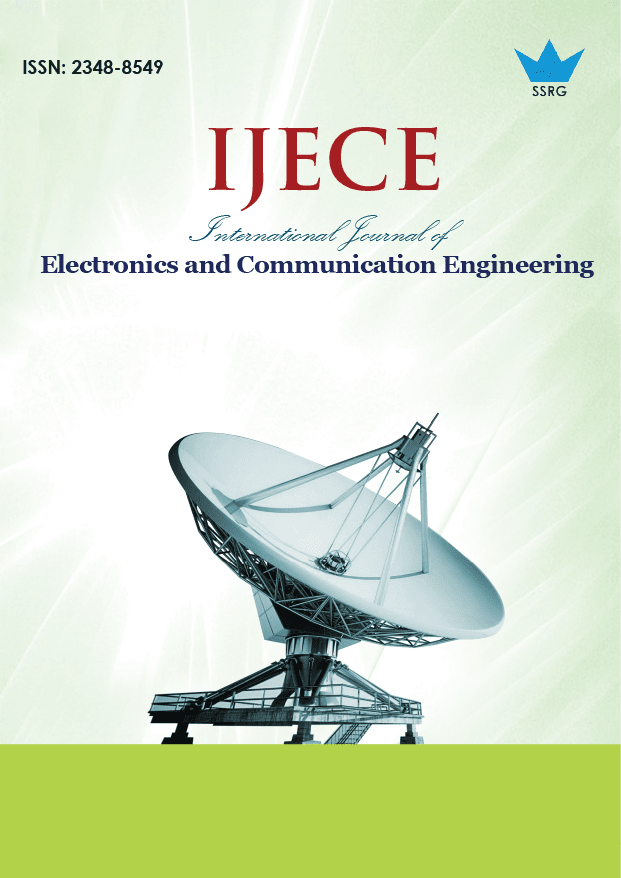
International Journal of Electronics and Communication Engineering |
© 2023 by SSRG - IJECE Journal |
Volume 10 Issue 7 |
Year of Publication : 2023 |
Authors : S. Umarani, Sunitha Y N, V Nuthan Prasad, KDV Prasad, Chanakya Kumar, Yogesh Mahajan |
How to Cite?
S. Umarani, Sunitha Y N, V Nuthan Prasad, KDV Prasad, Chanakya Kumar, Yogesh Mahajan, "IoT-Driven Image Processing Framework for Accurate Skin Diseases Diagnosis and Classification," SSRG International Journal of Electronics and Communication Engineering, vol. 10, no. 7, pp. 108-129, 2023. Crossref, https://doi.org/10.14445/23488549/IJECE-V10I7P111
Abstract:
Skin diseases affect millions worldwide, and accurate diagnosis and classification are critical for effective treatment. The Internet of Things (IoT) has emerged as a powerful technology that can be used to improve healthcare systems in recent years. This paper proposes an image processing framework based on IoT for accurate skin disease diagnosis and classification. To enable remote and real-time skin disease diagnosis, the proposed framework combines the capabilities of IoT devices, such as smartphones or wearable cameras, with advanced image processing techniques. The framework uses the cameras built into IoT devices to capture high-resolution images of the affected skin areas. After that, the images are securely transmitted to a central server or cloud-based platform for processing and analysis. The framework's image-processing component employs cutting-edge algorithms for image enhancement, feature extraction, and classification. Deep learning techniques, such as convolutional neural networks (CNNs), automatically extract relevant features from skin images. These characteristics are then used to classify skin diseases accurately. The framework incorporates robust encryption and authentication mechanisms during data transmission and storage to ensure the privacy and security of sensitive medical data. Patient consent and data anonymization techniques are also used to address privacy concerns. The proposed framework has several advantages over traditional methods for diagnosing skin diseases. It enables remote diagnosis by leveraging IoT devices, reducing the need for patients to travel to healthcare facilities. The real-time analysis allows for prompt intervention and treatment planning. Extensive experiments are carried out using a diverse dataset of skin disease images to assess the performance of the proposed framework. The findings show that the framework is highly accurate in diagnosing and classifying various skin diseases.
Keywords:
IoT (Internet of Things), Image processing, Skin diseases, Diagnosis, Authentication, Remote healthcare and Real-time analysis.
References:
[1] Marcos A M Almeida, and Iury A X Santos, "Classification Models for Skin Tumor Detection using Texture Analysis in Medical Images," Journal of Imaging, vol. 6, no. 6, pp. 51, 2020.
[CrossRef] [Google Scholar] [Publisher Link]
[2] Jainesh Rathod et al., "Diagnosis of Skin Diseases using Convolutional Neural Networks," Second International Conference on Electronics Communication and Aerospace Technology, pp. 1048-1051, 2018.
[CrossRef] [Google Scholar] [Publisher Link]
[3] Muhammad Ridaffa Purnomo, and Irma Palupi, "Classification of Skin Diseases to Detect their Causes using Convolutional Neural Networks," International Conference on Data Science and Its Applications, Bandung, Indonesia, pp. 187-193, 2021.
[CrossRef] [Google Scholar] [Publisher Link]
[4] Zhen Ma, and João Manuel R S Tavares, "A Novel Approach to Segment Skin Lesions in Dermoscopic Images Based on a Deformable Model," IEEE Journal of Biomedical and Health Informatics, vol. 20, no. 2, pp. 615-623, 2016.
[CrossRef] [Google Scholar] [Publisher Link]
[5] Zhe Wu et al., "Studies on Different CNN Algorithms for Face Skin Disease Classification Based on Clinical Images," IEEE Access, vol. 7, pp. 66505-66511, 2019.
[CrossRef] [Google Scholar] [Publisher Link]
[6] Piotr Dollár, and C. Lawrence Zitnick, "Fast Edge Detection using Structured Forests," IEEE Transactions on Pattern Analysis and Machine Intelligence, vol. 37, no. 8, pp. 1558-1570, 2015.
[CrossRef] [Google Scholar] [Publisher Link]
[7] Rajvi Parikh, and Hitesh Shah, "A Survey on Computer Vision Based Diagnosis for Skin Lesion Detection," International Journal of Engineering Science and Innovative Technology, vol. 2, no. 2, pp. 431-437, 2013.
[Google Scholar] [Publisher Link]
[8] Md. Anwar Hussen Wadud, M. F. Mridha, and Mohammad Motiur Rahman, "Word Embedding Methods for Word Representation in Deep Learning for Natural Language Processing," Iraqi Journal of Science, vol. 63, no. 3, pp. 1349-1361, 2022.
[CrossRef] [Google Scholar] [Publisher Link]
[9] Sheetalrani R Kawale et al., "An Intelligent System for Remote Monitoring of Patients Health and the Early Detection of Coronary Artery Disease," International Conference on Smart Generation Computing, Communication and Networking, Bangalore, India, pp. 1-6, 2022.
[CrossRef] [Google Scholar] [Publisher Link]
[10] K. Veera Swamy, and B. Divya, "Skin Disease Classification using Machine Learning Algorithms," 2nd International Conference on Communication, Computing and Industry 4.0, Bangalore, India, pp. 1-5, 2021.
[CrossRef] [Google Scholar] [Publisher Link]
[11] Jihan Alam, "An Efficient Approach for Skin Disease Detection using Deep Learning," IEEE Asia-Pacific Conference on Computer Science and Data Engineering, Brisbane, Australia, pp. 1-8, 2021.
[CrossRef] [Google Scholar] [Publisher Link]
[12] Igielska - Kalwat Joanna, and Kilian - Pięta Ewa, "The Use of Dalmatian Tansy and Auxiliary Substances as Natural Substitutes in the Treatment of Dermatosis," SSRG International Journal of Applied Chemistry, vol. 8, no. 3, pp. 1-9, 2021.
[CrossRef] [Google Scholar] [Publisher Link]
[13] Zhixiang Zhao et al., "A Novel Convolutional Neural Network for the Diagnosis and Classification of Rosacea: Usability Study," JMIR Medical Informatics, vol. 9, no. 3, 2021.
[CrossRef] [Google Scholar] [Publisher Link]
[14] N. Vikranth Kumar et al., “Classification of Skin Diseases using Image Processing and SVM,” International Conference on Vision towards Emerging Trends in Communication and Networking, pp. 1-5, 2019.
[CrossRef] [Google Scholar] [Publisher Link]
[15] M Ramesha et al., "An Integrated IoT Technology for Health and Traffic Monitoring System with Smart Ambulance," IEEE North Karnataka Subsection Flagship International Conference, Vijaypur, India, pp. 1-6, 2022.
[CrossRef] [Google Scholar] [Publisher Link]
[16] K. Neetu Chikyal, and Veera Swamy, "Performance Assessment of Various Thyroid Image Segmentation Techniques with Consistency Verification," Journal of Advance Research in Dynamical & Control Systems, vol. 11, no. 2, pp. 1299-1309, 2019.
[Publisher Link]
[17] Muhammad Attique Khan et al., "Multi-Class Skin Lesion Detection and Classification via Teledermatology," IEEE Journal of Biomedical and Health Informatics, vol. 25, no. 12, pp. 4267-4275, 2021.
[CrossRef] [Google Scholar] [Publisher Link]
[18] V. Dankan Gowda et al., "Implementation of a Machine Learning-Based Model for Cardiovascular Disease Post Exposure prophylaxis," International Conference for Advancement in Technology, Goa, India, pp. 1-5, 2023.
[CrossRef] [Google Scholar] [Publisher Link]
[19] Archana Ajith et al., "Digital Dermatology: Skin Disease Detection Model using Image Processing," International Conference on Intelligent Computing and Control Systems, pp. 168-173, 2017.
[CrossRef] [Google Scholar] [Publisher Link]
[20] Md. Anwar Hussen Wadud, Mohammed Alatiyyah, and M. F. Mridha, "Non-Autoregressive End-to-End Neural Modeling for Automatic Pronunciation Error Detection," Applied Sciences, vol. 13, no. 1, p. 109, 2022.
[CrossRef] [Google Scholar] [Publisher Link]
[21] K. Manivannan, and S. Sathiamoorthy, "Bird Mating Optimizer with Deep Learning-based Tuberculosis Detection using Chest Radiographs," International Journal of Engineering Trends and Technology, vol. 71, no. 2, pp. 341-348, 2023.
[CrossRef] [Publisher Link]
[22] Vinayshekhar Bannihatti Kumar, Sujay S Kumar, and Varun Saboo, "Dermatological Disease Detection using Image Processing and Machine Learning," Third International Conference on Artificial Intelligence and Pattern Recognition, pp. 1-6, 2016.
[CrossRef] [Google Scholar] [Publisher Link]
[23] Avinash Sharma et al., "Extraction of Fetal ECG using ANFIS and the Undecimated-Wavelet Transform," IEEE 3rd Global Conference for Advancement in Technology, pp. 1-5, 2022.
[CrossRef] [Google Scholar] [Publisher Link]
[24] K. C. Shahana sherin, and R. Shayini, "Classification of Skin Lesions in Digital Images for the Diagnosis of Skin Cancer," International Conference on Smart Electronics and Communication, Trichy, India, pp. 162-166, 2020.
[CrossRef] [Google Scholar] [Publisher Link]
[25] Avinash Sharma et al., “Computer Vision Based Healthcare System for Identification of Diabetes & Its Types using AI,” Measurement: Sensors, vol. 27, p. 10075, 2023.
[CrossRef] [Google Scholar] [Publisher Link]
[26] Adheena Santy, and Robin Joseph, "Segmentation Methods for Computer Aided Melanoma Detection," Global Conference on Communication Technologies, pp. 490-493, 2015.
[CrossRef] [Google Scholar] [Publisher Link]
[27] C. Saraswathi, and B. Pushpa, "Computer Imaging of Alopecia Areata and Scalp Detection: A Survey," International Journal of Engineering Trends and Technology, vol. 70, no. 8, pp. 347-358, 2022.
[CrossRef] [Publisher Link]
[28] Ranjeet Suryawanshi et al., “Enhanced Diagnostic Methods for Identifying Anomalies in Imaging of Skin Lesions,” International Journal of Electrical and Electronics Research, vol. 10, no. 4, pp. 1077-1085, 2022.
[CrossRef] [Google Scholar] [Publisher Link]
[29] Habeba Mahmoud, Mohamed Abdel-Nasser, and Osama A Omer, "Computer Aided Diagnosis System for Skin Lesions Detection using Texture Analysis Methods," International Conference on Innovative Trends in Computer Engineering, pp. 140-144, 2018.
[CrossRef] [Google Scholar] [Publisher Link]
[30] P. Ajay et al., "Intelligent Breast Abnormality Framework for Detection and Evaluation of Breast Abnormal Parameters," International Conference on Edge Computing and Applications, pp. 1503-1508, 2022.
[CrossRef] [Google Scholar] [Publisher Link]
[31] Parameshwar R. Hegde, Manjunath M. Shenoy, and B.H. Shekar, "Comparison of Machine Learning Algorithms for Skin Disease Classification Using Color and Texture Features," International Conference on Advances in Computing Communications and Informatics, pp. 1825-1828, 2018.
[CrossRef] [Google Scholar] [Publisher Link]
[32] M Ramesha et al., "Implementation of IoT Based Wireless Electronic Stethoscope," Third International Conference on Multimedia Processing, Communication & Information Technology, pp. 103-106, 2020.
[CrossRef] [Google Scholar] [Publisher Link]
[33] M. Chandrahasa, Varun Vadigeri, and Dixit Salecha, "Detection of Skin Cancer using Image Processing Techniques," International Journal of Modern Trends in Engineering and Research, vol. 3, no. 5, pp. 111-114, 2016.
[Google Scholar]
[34] Aya Abu Ali, and Hasan Al-Marzouqi, "Melanoma Detection using Regular Convolutional Neural Networks," International Conference on Electrical and Computing Technologies and Applications, pp. 1-5, 2017.
[CrossRef] [Google Scholar] [Publisher Link]
[35] M Koteswara Rao, K. Veera Swamy, and K Anitha Sheela, "Advanced Machine Learning Discriminant Analysis Models for Face Retrieval System," 2nd International Conference on I-SMAC (IoT in Social Mobile Analytics and Cloud) (I-SMAC), pp. 609-613, 2018.
[CrossRef] [Google Scholar] [Publisher Link]
[36] Seema Kolkur, and D.R. Kalbande, "Survey of Texture based Feature Extraction for Skin Disease Detection," International Conference on ICT in Business Industry & Government, pp. 1-6, 2016.
[CrossRef] [Google Scholar] [Publisher Link]
[37] Sakil Ahammed et al., "An IoT-based Real-Time Remote Health Monitoring System," International Journal of Recent Engineering Science, vol. 8, no. 3, pp. 23-29, 2021.
[Google Scholar] [Publisher Link]
[38] Lincy Vincent, and J Roopa Jayasingh, "Comparison of Psoriasis Disease De-Tection and Classification through Various Image Processing Techniques-A Review," 6th International Conference on Devices Circuits and Systems, pp. 122-124, 2022.
[CrossRef] [Google Scholar] [Publisher Link]
[39] Alaa Haddad, and Shihab A. Hameed, "Image Analysis Model for Skin Disease Detection: Framework," 7th International Conference on Computer and Communication Engineering, pp. 1-4, 2018.
[CrossRef] [Google Scholar] [Publisher Link]
[40] R. Kavitha et al., "Cardiovascular Disease Prediction using LSTM Algorithm Based on Cytokines," 4th International Conference for Emerging Technology, Belgaum, India, pp. 1-5, 2023.
[CrossRef] [Google Scholar] [Publisher Link]
[41] Yujun Yang, Jianping Li, and Yimei Yang, "The Research of the Fast SVM Classifier Method," 12th International Computer Conference on Wavelet Active Media Technology and Information Processing, pp. 121-124, 2015.
[CrossRef] [Google Scholar] [Publisher Link]
[42] Shamim Ahmed et al., "Human Skin Diseases Detection and Classification using CNN," International Conference on Electrical, Computer and Communication Engineering, Chittagong, Bangladesh, pp. 1-6, 2023.
[CrossRef] [Google Scholar] [Publisher Link]