Leveraging Semi-Supervised Graph Learning for Enhanced Diabetic Retinopathy Detection
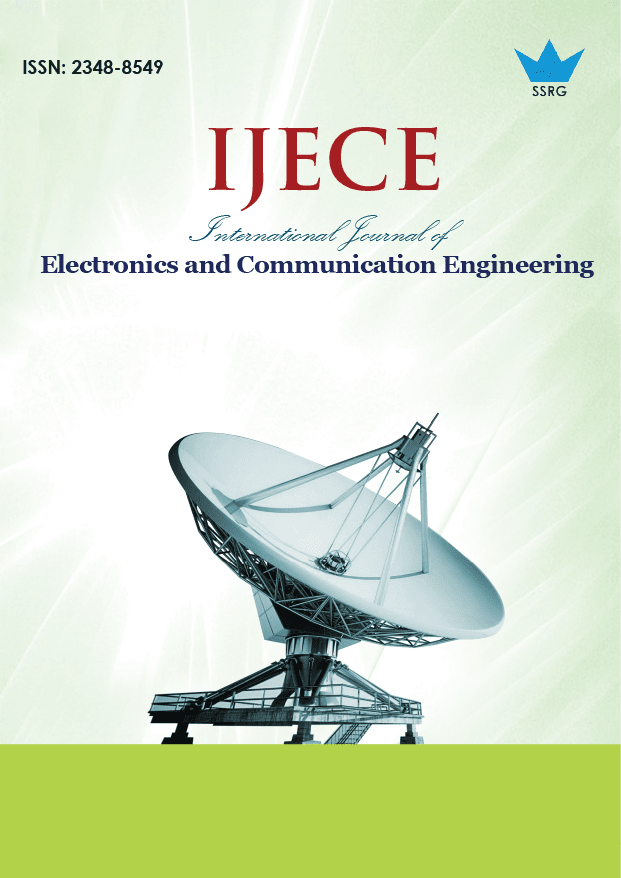
International Journal of Electronics and Communication Engineering |
© 2023 by SSRG - IJECE Journal |
Volume 10 Issue 8 |
Year of Publication : 2023 |
Authors : D. Dhinakaran, L. Srinivasan, D. Selvaraj, S. M. Udhaya Sankar |
How to Cite?
D. Dhinakaran, L. Srinivasan, D. Selvaraj, S. M. Udhaya Sankar, "Leveraging Semi-Supervised Graph Learning for Enhanced Diabetic Retinopathy Detection," SSRG International Journal of Electronics and Communication Engineering, vol. 10, no. 8, pp. 9-21, 2023. Crossref, https://doi.org/10.14445/23488549/IJECE-V10I8P102
Abstract:
Diabetic Retinopathy (DR) is a significant cause of blindness globally, highlighting the urgent need for early detection and effective treatment. Recent advancements in Machine Learning (ML) techniques have shown promise in DR detection, but the availability of labeled data often limits their performance. This research proposes a novel Semi-Supervised Graph Learning – SSGL algorithm tailored for DR detection, which capitalizes on the relationships between labelled and unlabeled data to enhance accuracy. The work begins by investigating data augmentation and preprocessing techniques to address the challenges of image quality and feature variations. Techniques such as image cropping, resizing, contrast adjustment, normalization, and data augmentation (rotation, flipping, Gaussian noise addition, and blurring) are explored to optimize feature extraction and improve the overall quality of retinal images.Moreover, apart from detection and diagnosis, this work delves into applying ML algorithms for predicting the risk of developing DR or the likelihood of disease progression. Personalized risk scores for individual patients are generated using comprehensive patient data encompassing demographic information, medical history, and retinal images. The proposed Semi-Supervised Graph learning algorithm is rigorously evaluated on two publicly available datasets and is benchmarked against existing methods. Results indicate significant improvements in classification accuracy, specificity, and sensitivity while demonstrating robustness against noise and outliers. Notably, the proposed algorithm addresses the challenge of imbalanced datasets, common in medical image analysis, further enhancing its practical applicability.
Keywords:
Diabetic retinopathy, Machine learning, Graph learning, Data augmentation, Normalization.
References:
[1] Thippa Reddy Gadekallu et al., “Early Detection of Diabetic Retinopathy using PCA-Firefly Based Deep Learning Model,” Electronics, vol. 9, no. 2, p. 274, 2020.
[CrossRef] [Google Scholar] [Publisher Link]
[2] Imran Qureshi, Jun Ma, and Qaisar Abbas, “Diabetic Retinopathy Detection and Stage Classification in Eye Fundus Images using Active Deep Learning,” Multimedia Tools and Applications, vol. 80, pp. 11691–11721, 2021.
[CrossRef] [Google Scholar] [Publisher Link]
[3] L. Srinivasan et al., “IoT-Based Solution for Paraplegic Sufferer to Send Signals to Physician via Internet,” SSRG International Journal of Electrical and Electronics Engineering, vol. 10, no. 1, pp. 41-52, 2023.
[CrossRef] [Google Scholar] [Publisher Link]
[4] S. M. Udhaya Sankar et al., “Machine Learning System for Indolence Perception,” International Conference on Innovative Data Communication Technologies and Application (ICIDCA), pp. 55-60, 2023.
[CrossRef] [Google Scholar] [Publisher Link]
[5] Shilpa Joshi, and P. T. Karule, “Haemorrhages Detection using Geometrical Techniques,” Computer Methods in Biomechanics and Biomedical Engineering: Imaging & Visualization, vol. 8, no. 4, pp. 436–445, 2020.
[CrossRef] [Google Scholar] [Publisher Link]
[6] S. M. Udhaya Sankar et al., “Safe Routing Approach by Identifying and Subsequently Eliminating the Attacks in MANET,” International Journal of Engineering Trends and Technology, vol. 70, no. 11, pp. 219-231, 2022.
[CrossRef] [Google Scholar] [Publisher Link]
[7] Dhinakaran D et al., “Mining Privacy-Preserving Association Rules Based on Parallel Processing in Cloud Computing,” International Journal of Engineering Trends and Technology, vol. 70, no. 3, pp. 284-294, 2022.
[CrossRef] [Google Scholar] [Publisher Link]
[8] Muhammad Moazam Fraz et al., “An Ensemble Classification-Based Approach Applied to Retinal Blood Vessel Segmentation,” IEEE Transactions on Biomedical Engineering, vol. 59, no. 9, pp. 2538–2548, 2012.
[CrossRef] [Google Scholar] [Publisher Link]
[9] F. Orujov et al., “Fuzzy Based Image Edge Detection Algorithm for Blood Vessel Detection in Retinal Images,” Applied Soft Computing, vol. 94, p. 106452, 2020.
[CrossRef] [Google Scholar] [Publisher Link]
[10] S. Fowjiya, M. Karnan, and R. Sivakumar, “An Automatic Detection and Assessment of Diabetic Macular Edema along with Fovea Detection from Color Retinal Images,” International Journal of Computer & Organization Trends (IJCOT), vol. 3, no. 1, pp. 49-53, 2013.
[Google Scholar] [Publisher Link]
[11] Shailesh Kumar et al., “An Automated Early Diabetic Retinopathy Detection through Improved Blood Vessel and Optic Disc Segmentation,” Optics & Laser Technology, vol. 121, p. 105815, 2020.
[CrossRef] [Google Scholar] [Publisher Link]
[12] Chloe Bedard et al., “Systematic Review: Can Non-Mydriatic Cameras Accurately Detect Diabetic Retinopathy?,” Diabetes Research and Clinical Practice, vol. 129, pp. 154-159, 2017.
[CrossRef] [Google Scholar] [Publisher Link]
[13] Frank D. Verbraak et al., “Diagnostic Accuracy of a Device for the Automated Detection of Diabetic Retinopathy in a Primary Care Setting,” Diabetes Care, vol. 42, no. 4, pp. 651-656, 2019.
[CrossRef] [Google Scholar] [Publisher Link]
[14] Shakti Chourasiya, and Suvrat Jain, “A Study Review on Supervised Machine Learning Algorithms,” SSRG International Journal of Computer Science and Engineering, vol. 6, no. 8, pp. 16-20, 2019.
[CrossRef] [Google Scholar] [Publisher Link]
[15] Mohsen Hajabdollahi et al., “Hierarchical Pruning for Simplification of Convolutional Neural Networks in Diabetic Retinopathy Classification,” 41st Annual International Conference of the IEEE Engineering in Medicine and Biology Society (EMBC), pp. 970-973, 2019.
[CrossRef] [Google Scholar] [Publisher Link]
[16] Lifeng Qiao, Ying Zhu, and Hui Zhou, “Diabetic Retinopathy Detection using Prognosis of Microaneurysm and Early Diagnosis System for Non-Proliferative Diabetic Retinopathy Based on Deep Learning Algorithms,” IEEE Access, vol. 8, pp. 104292-104302, 2020.
[CrossRef] [Google Scholar] [Publisher Link]
[17] Hongyang Jiang et al., “An Interpretable Ensemble Deep Learning Model for Diabetic Retinopathy Disease Classification,” 41st Annual International Conference of the IEEE Engineering in Medicine and Biology Society (EMBC), pp. 2045-2048, 2019.
[CrossRef] [Google Scholar] [Publisher Link]
[18] Hongyang Jiang et al., “A Multi-Label Deep Learning Model with Interpretable Grad-CAM for Diabetic Retinopathy Classification,” 42nd Annual International Conference of the IEEE Engineering in Medicine & Biology Society (EMBC), pp. 1560-1563, 2020.
[CrossRef] [Google Scholar] [Publisher Link]
[19] Alan Lands et al., “Implementation of Deep Learning Based Algorithms for Diabetic Retinopathy Classification from Fundus Images,” 4 th International Conference on Trends in Electronics and Informatics (ICOEI)(48184), pp. 1028-1032, 2020.
[CrossRef] [Google Scholar] [Publisher Link]
[20] Doaa K. Elswah, Ahmed A. Elnakib, and Hossam El-din Moustafa, “Automated Diabetic Retinopathy Grading using Resnet,” 37th National Radio Science Conference (NRSC), pp. 248-254, 2020.
[CrossRef] [Google Scholar] [Publisher Link]
[21] Sheeba Lal et al., “Adversarial Attack and Defence through Adversarial Training and Feature Fusion for Diabetic Retinopathy Recognition,” Sensors, vol. 21, no. 11, pp. 1-21, 2021.
[CrossRef] [Google Scholar] [Publisher Link]
[22] Sarmad Maqsood, Robertas Damaševičius, and Rytis Maskeliūnas, “Hemorrhage Detection Based on 3D CNN Deep Learning Framework and Feature Fusion for Evaluating Retinal Abnormality in Diabetic Patients,” Sensors, vol. 21, no. 11, pp. 1-21, 2021.
[CrossRef] [Google Scholar] [Publisher Link]
[23] A. Boned-Murillo et al., “Structural and Functional Findings in Patients with Moderate Diabetic Retinopathy,” Graefe's Archive for Clinical and Experimental Ophthalmology, vol. 259, pp. 3625-3635, 2021.
[CrossRef] [Google Scholar] [Publisher Link]
[24] H. B. Kapti, A. K. Sahin, and O. Ozdemir, “Evaluation of Retinal and Choroidal Thicknesses in Patients with Diabetes Mellitus without Diabetic Retinopathy: A Comparative Study,” Nigerian Journal of Clinical Practice, vol. 25, no. 9, pp. 1495-1500, 2022.
[CrossRef] [Google Scholar] [Publisher Link]
[25] D. Selvaraj et al., “Outsourced Analysis of Encrypted Graphs in the Cloud with Privacy Protection,” SSRG International Journal of Electrical and Electronics Engineering, vol. 10, no. 1, pp. 53-62, 2023.
[CrossRef] [Google Scholar] [Publisher Link]
[26] Dhinakaran D et al., “IOT-Based Whip-Smart Trash Bin using LoRa WAN,” Expert Clouds and Applications, vol. 673, 2023.
[CrossRef] [Google Scholar] [Publisher Link]
[27] D. Dhinakaran, “Dam Management and Disaster Monitoring System using IoT,” International Conference on Sustainable Computing and Data Communication Systems (ICSCDS), pp. 1197-1201, 2023.
[CrossRef] [Google Scholar] [Publisher Link]
[28] S. M. Udhaya Sankar et al., “A Way for Smart Home Technology for Disabled and Elderly People,” International Conference on Innovative Data Communication Technologies and Application (ICIDCA), pp. 369-373, 2023.
[CrossRef] [Google Scholar] [Publisher Link]
[29] G. K. Monica et al., “MEMS Based Sensor Robot for Immobilized Persons,” International Conference on Innovative Data Communication Technologies and Application (ICIDCA), pp. 924-929, 2023.
[CrossRef] [Google Scholar] [Publisher Link]
[30] K. Sudharson et al., “Efficient Soil Condition Monitoring with IoT Enabled Intelligent Farming Solution,” IEEE International Students’ Conference on Electrical, Electronics and Computer Science (SCEECS), pp. 1-6, 2023.
[CrossRef] [Google Scholar] [Publisher Link]
[31] K. Shankar et al., “Automated Detection and Classification of Fundus Diabetic Retinopathy Images using Synergic Deep Learning Model,” Pattern Recognition Letters, vol. 133, pp. 210–216, 2020.
[CrossRef] [Google Scholar] [Publisher Link]
[32] B. Venkaiahppalaswamy, PVGD Prasad Reddy, and Suresh Batha, “An Effective Diagnosis of Diabetic Retinopathy Based on 3d Hybrid Squeezenet Architecture,” International Journal of Engineering Trends and Technology, vol. 70, no. 12, pp. 147-159, 2022.
[CrossRef] [Publisher Link]
[33] Michael David Abràmoff et al., “Improved Automated Detection of Diabetic Retinopathy on a Publicly Available Dataset through Integration of Deep Learning,” Investigative Ophthalmology & Visual Science, vol. 57, no. 13, pp. 5200-5206, 2016.
[CrossRef] [Google Scholar] [Publisher Link]
[34] Varun Gulshan et al., “Development and Validation of a Deep Learning Algorithm for Detection of Diabetic Retinopathy in Retinal Fundus Photographs,” JAMA, vol. 316, no. 22, pp. 2402-2410, 2016.
[CrossRef] [Google Scholar] [Publisher Link]