A Hybrid Machine Learning Model with Combined Wrapper Feature Selection Techniques to Improve the Yield of Paddy
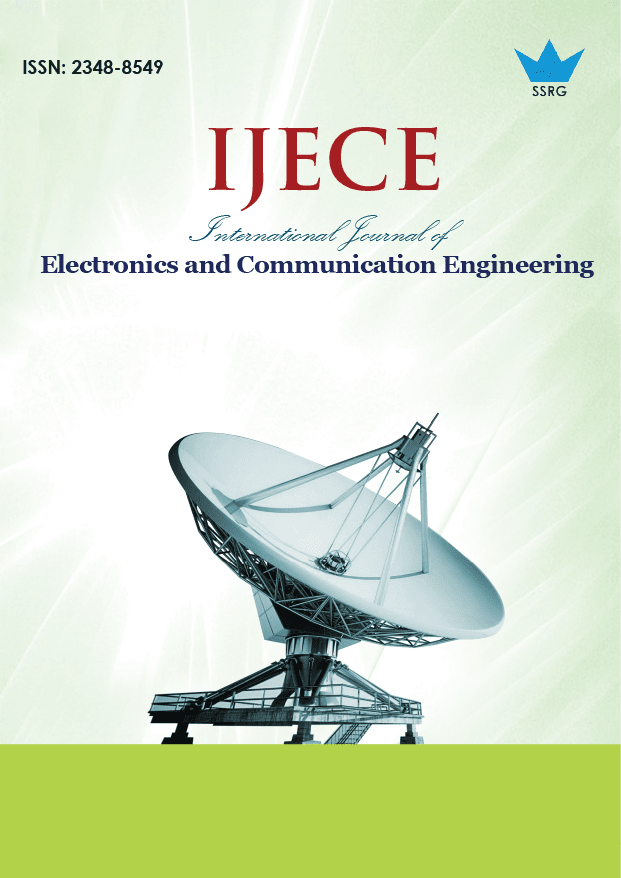
International Journal of Electronics and Communication Engineering |
© 2023 by SSRG - IJECE Journal |
Volume 10 Issue 12 |
Year of Publication : 2023 |
Authors : S. Muthukumaran, K. John Peter, E. Dilipkumar, S. Savithri, K. Senbagam |
How to Cite?
S. Muthukumaran, K. John Peter, E. Dilipkumar, S. Savithri, K. Senbagam, "A Hybrid Machine Learning Model with Combined Wrapper Feature Selection Techniques to Improve the Yield of Paddy," SSRG International Journal of Electronics and Communication Engineering, vol. 10, no. 12, pp. 45-61, 2023. Crossref, https://doi.org/10.14445/23488549/IJECE-V10I12P105
Abstract:
A third of the earth’s surface is taken up by agriculture, which is essential to the food production process. Paddy seeds are used to grow rice, which is a dependable food that is consumed by approximately half of all people worldwide. The alarming rate of population expansion makes it necessary for us to secure food security, and the nation should implement the measures required to increase the production of food grains. Since climatic, agronomic, irrigational, and cultivation techniques all affect paddy’s growth. The goal of the study is to increase the production of rice by using Machine Learning (ML) techniques to forecast the variables that affect paddy growth. More attributes will be employed to build the dataset as ML techniques are used in real-time, which will reduce model performance, raise computing costs, and make the dataset more susceptible to overfitting. This research developed a Hybrid Machine Learning Model with Combined Wrapper Feature Selection Techniques (HMLCWFS) for forecasting paddy production to get over these challenges. The suggested approach selects the most significant features from the Paddy Dataset (PD) using five Feature Selection (FS) approaches: Backward Elimination (BE), Stepwise Forward Selection (SFS), Feature Importance (FI), Exhaustive FS (EFS), and Gradient Boosting (GB) approaches. Using Poincare’s formula, the attributes chosen from each FS approach were concatenated, and the dataset was then recreated. The reconstructed dataset was used to deploy ML approaches like Decision Tree (DT) and Random Forest (RF), and the knowledge gleaned in the form of association rules was utilized to provide advice to paddy growers on how to increase productivity. The suggested model also takes into account the farmers’ preferred paddy farming techniques and makes recommendations regarding which paddy variety they should cultivate. This is accomplished by connecting the input parameters to the real-time PD trained by employing Support Vector Machine (SVM), K-Nearest Neighbor (KNN), and Naive Bayes (NB) methods. The classifier’s results were compared using performance metrics, and the findings demonstrate that the combined FS strategies employed in this research help to identify the elements contributing to the paddy crop’s improvement.
Keywords:
Feature Selection, Supervised Machine Learning, Paddy cultivation, SVM, Decision Tree, KNN.
References:
[1] Chandraprabha M., and Rajesh Kumar Dhanraj, “Ensemble Deep Learning Algorithm for Forecasting of Rice Crop Yield Based on Soil Nutrition Levels,” EAI Endorsed Transactions on Scalable Information Systems, vol. 10, no. 4, pp. 1-11, 2023.
[CrossRef] [Google Scholar] [Publisher Link]
[2] Md Didarul Islam et al., “Rapid Rice Yield Estimation Using Integrated Remote Sensing and Meteorological Data and Machine Learning,” Remote Sensing, vol. 15, no. 9, pp. 1-16, 2023.
[CrossRef] [Google Scholar] [Publisher Link]
[3] Baishun Liu et al., “Comparison of Yield Prediction Models and Estimation of the Relative Importance of Main Agronomic Traits Affecting Rice Yield Formation in Saline-Sodic Paddy Fields,” European Journal of Agronomy, vol. 148, 2023.
[CrossRef] [Google Scholar] [Publisher Link]
[4] A. Zhiwei Ye et al., “High-Dimensional Feature Selection Based on Improved Binary Ant Colony Optimization Combined with Hybrid Rice Optimization Algorithm,” International Journal of Intelligent Systems, vol. 2023, pp. 1-27, 2023.
[CrossRef] [Google Scholar] [Publisher Link]
[5] Yue Wang, and Yuechen Li, “Mapping the Ratoon Rice Suitability Region in China Using Random Forest and Recursive Feature Elimination Modeling,” Field Crops Research, vol. 301, 2023.
[CrossRef] [Google Scholar] [Publisher Link]
[6] V. Malathi et al., “Enhancing the Paddy Disease Classification by Using Cross-Validation Strategy for Artificial Neural Network over Baseline Classifiers,” Journal of Sensors, vol. 2023, pp. 1-13, 2023.
[CrossRef] [Google Scholar] [Publisher Link]
[7] Vinson Joshua, Selwin Mich Priyadharson, and Raju Kannadasan, “Exploration of Machine Learning Approaches for Paddy Yield Prediction in Eastern Part of Tamilnadu,” Agronomy, vol. 11, no. 10, pp. 1-19, 2021.
[CrossRef] [Google Scholar] [Publisher Link]
[8] A. Suruliandi, G. Mariammal, and S.P. Raja, “Crop Prediction Based on Soil and Environmental Characteristics Using Feature Selection Techniques,” Mathematical and Computer Modelling of Dynamical Systems, vol. 27, no. 1, pp. 117-140, 2021.
[CrossRef] [Google Scholar] [Publisher Link]
[9] Sangeetham Rohini, and S. Narayana Reddy, “Machine Learning Based Techniques for Paddy Yield Prediction for the State of Andhra Pradesh,” International Journal of Intelligent Systems and Applications in Engineering, vol. 1, no. 6S, pp. 753-764, 2023.
[Google Scholar] [Publisher Link]
[10] A.S.M. Mahmudul Hasan et al., “A Survey of Deep Learning Techniques for Weed Detection from Images,” Computers and Electronics in Agriculture, vol. 184, 2021.
[CrossRef] [Google Scholar] [Publisher Link]
[11] Alexandros Oikonomidis, Cagatay Catal, and Ayalew Kassahun, “Hybrid Deep Learning-Based Models for Crop Yield Prediction,” Applied Artificial Intelligence, vol. 36, no. 1, pp. 1933-1950, 2022.
[CrossRef] [Google Scholar] [Publisher Link]
[12] Bhuvaneswari Swaminathan, P. Saravanan, and V. Subramaniyaswamy, “Fertilizer Recommendation System for High Crop Yield Based on Prediction Model: A Comparative Analysis,” Advances in Data Science and Computing Technologies, pp. 1-8, 2022.
[CrossRef] [Google Scholar] [Publisher Link]
[13] P. Sathya, and P. Gnanasekaran, “Ensemble Feature Selection Framework for Paddy Yield Prediction in Cauvery Basin Using Machine Learning Classifiers,” Cogent Engineering, vol. 10, no. 2, pp. 1-18, 2023.
[CrossRef] [Google Scholar] [Publisher Link]
[14] Guojie Ruan et al., “Improving Wheat Yield Prediction Integrating Proximal Sensing and Weather Data with Machine Learning,” Computers and Electronics in Agriculture, vol. 195, 2022.
[CrossRef] [Google Scholar] [Publisher Link]
[15] Mohsen Yoosefzadeh-Najafabadi, Dan Tulpan, and Milad Eskandari, “Using Hybrid Artificial Intelligence and Evolutionary Optimization Algorithms for Estimating Soybean Yield and Fresh Biomass Using Hyperspectral Vegetation Indices,” Remote Sensing, vol. 13, no. 13, pp. 1-21, 2021.
[CrossRef] [Google Scholar] [Publisher Link]
[16] Anurag Satpathi et al., “Comparative Analysis of Statistical and Machine Learning Techniques for Rice Yield Forecasting for Chhattisgarh, India,” Sustainability, vol. 15, no. 3, pp. 1-18, 2023.
[CrossRef] [Google Scholar] [Publisher Link]
[17] Nilesh Kumar Singh, Shraddha Rawat, and Shweta Gautam, “Weather-Based Rice Crop Yield Forecasting Using Different Regression Techniques & Neural Network Approach for Prayagraj Region,” International Journal of Environment and Climate Change, vol. 13, no. 10, pp. 2425-2435, 2023.
[CrossRef] [Google Scholar] [Publisher Link]