Signal Pre-Processing and Classification Algorithms for the Automatic Identification of Insomnia: A Short Review
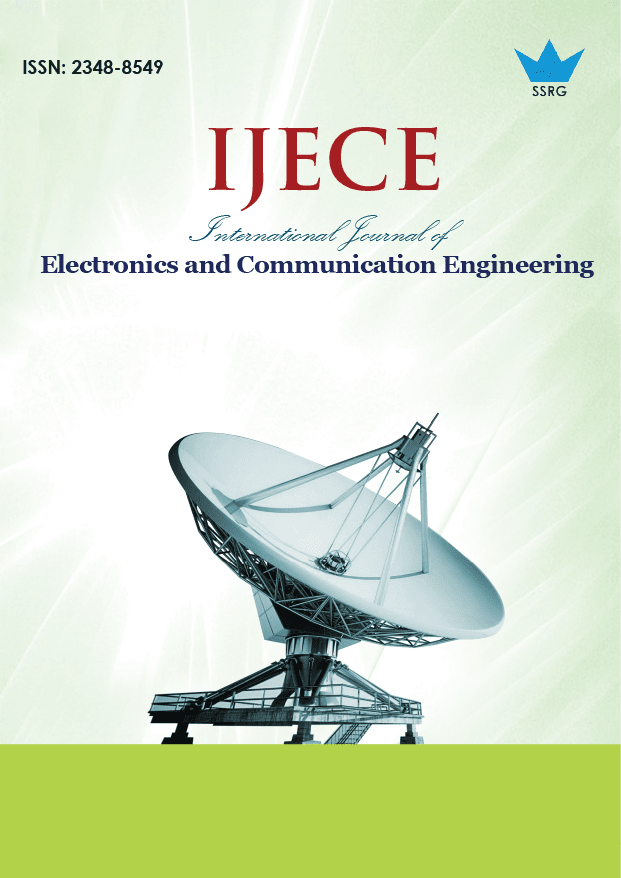
International Journal of Electronics and Communication Engineering |
© 2023 by SSRG - IJECE Journal |
Volume 10 Issue 12 |
Year of Publication : 2023 |
Authors : Steffi Philip Mulamoottil, Tandavan Vigneswaran |
How to Cite?
Steffi Philip Mulamoottil, Tandavan Vigneswaran, "Signal Pre-Processing and Classification Algorithms for the Automatic Identification of Insomnia: A Short Review," SSRG International Journal of Electronics and Communication Engineering, vol. 10, no. 12, pp. 62-72, 2023. Crossref, https://doi.org/10.14445/23488549/IJECE-V10I12P106
Abstract:
Insomnia is a sleep disorder when untreated, develops physiological, psychological, or psychiatric conditions like cardiovascular diseases, diabetes, stress, anxiety, memory loss, etc., for all age groups, mainly adults and older people. Identifying insomnia is cumbersome and demands human effort at the time of diagnosis. Enormous automated techniques were leveraged that can be viewed from the literature. The paper reviews various signal-processing methods that are helpful for artefact-free input and classification algorithms to identify the disorder automatically. This article looks into six signalprocessing methods, three feature extraction, and seven classification algorithms, focusing on their advantages, disadvantages, and limitations. The presented paper concentrates on delivering a detailed review of the methods used in the detection process and can help choose an appropriate model. The work can perform a guideline for identifying insomnia using physiological signals.
Keywords:
Sleep disorder, Machine Learning, EEG processing, Feature extraction, Deep Learning.
References:
[1] Christopher L. Drake, Timothy Roehrs, and Thomas Roth, “Insomnia Causes, Consequences, and Therapeutics: An Overview,” Depression and Anxiety, vol. 18, no. 4, pp. 163-176, 2003.
[CrossRef] [Google Scholar] [Publisher Link]
[2] Amal M. Osman et al., “Obstructive Sleep Apnea: Current Perspectives,” Nature and Science of Sleep, vol. 10, pp. 21-34, 2018.
[CrossRef] [Google Scholar] [Publisher Link]
[3] Shiyi Guo et al., “Restless Legs Syndrome: from Pathophysiology to Clinical Diagnosis and Management,” Frontiers in Aging Neuroscience, vol. 9, pp. 1-14, 2017.
[CrossRef] [Google Scholar] [Publisher Link]
[4] Franco Ferrillo et al., “A Model-Based Approach to Homeostatic and Ultradian Aspects of Nocturnal Sleep Structure in Narcolepsy,” Sleep, vol. 30, no. 2, pp. 157-165, 2007.
[CrossRef] [Google Scholar] [Publisher Link]
[5] Nathaniel F. Watson et al., “Recommended Amount of Sleep for a Healthy Adult: A Joint Consensus Statement of the American Academy of Sleep Medicine and Sleep Research Society,” Sleep, vol. 38, no. 6, pp. 843-844, 2015.
[CrossRef] [Google Scholar] [Publisher Link]
[6] Goran Medic, Micheline Wille, and Michiel E.H. Hemels, “Short- and Long-Term Health Consequences of Sleep Disruption,” Nature and Science of Sleep, vol. 9, pp. 151-161, 2017.
[CrossRef] [Google Scholar] [Publisher Link]
[7] Dayanand Vishwanath Dhongade, and T.V.K.H. Rao, “Classification of Sleep Disorders Based on EEG Signals by Using Feature Extraction Techniques with KNN Classifier,” 2017 International Conference on Innovations in Green Energy and Healthcare Technologies (IGEHT), Coimbatore, India, pp. 1-5, 2017.
[CrossRef] [Google Scholar] [Publisher Link]
[8] Richard W. Homan, “The 10-20 Electrode System and Cerebral Location,” American Journal of EEG Technology, vol. 28, no. 4, pp. 269-279, 1988.
[CrossRef] [Google Scholar] [Publisher Link]
[9] Gonzalo M. Rojas et al., “Study of Resting-State Functional Connectivity Networks Using EEG Electrodes Position as Seed,” Frontiers in Neuroscience, vol. 12, pp. 1-12, 2018.
[CrossRef] [Google Scholar] [Publisher Link]
[10] P.Y. Ktonas, P.L. Osorio, and R.L. Everett, “Automated Detection of EEG Artifacts during Sleep: Preprocessing for All-Night Spectral Analysis,” Electroencephalography and Clinical Neurophysiology, vol. 46, no. 4, pp. 382-388, 1979.
[CrossRef] [Google Scholar] [Publisher Link]
[11] A. Berger, D.P.F. Möller, and M. Reuter, “Detection of Sleep with New Preprocessing Methods for EEG Analysing,” International Conference on Computational Intelligence: Computational Intelligence Theory and Applications, pp. 304-310, 1997.
[CrossRef] [Google Scholar] [Publisher Link]
[12] Nima Bigdely-Shamlo et al., “The PREP Pipeline: Standardized Preprocessing for Large-Scale EEG Analysis,” Frontiers in Neuroinformatics, vol. 9, pp. 1-20, 2015.
[CrossRef] [Google Scholar] [Publisher Link]
[13] Laurel J. Gabard-Durnam et al., “The Harvard Automated Processing Pipeline for Electroencephalography (HAPPE): Standardized Processing Software for Developmental and High-Artifact Data,” Frontiers in Neuroscience, vol. 12, pp. 1-24, 2018.
[CrossRef] [Google Scholar] [Publisher Link]
[14] Somayeh Raiesdana, “Automated Sleep Staging of OSAs Based on ICA Preprocessing and Consolidation of Temporal Correlations,” Australasian Physical & Engineering Sciences in Medicine, vol. 41, no. 1, pp. 161-176, 2018.
[CrossRef] [Google Scholar] [Publisher Link]
[15] Hui Huang et al., “EEG-Based Sleep Staging Analysis with Functional Connectivity,” Sensors, vol. 21, no. 6, pp. 1-15, 2021.
[CrossRef] [Google Scholar] [Publisher Link]
[16] Ian T. Jolliffe, and Jorge Cadima, “Principal Component Analysis: A Review and Recent Developments,” Philosophical Transactions of the Royal Society A: Mathematical, Physical and Engineering Sciences, vol. 374, no. 2065, pp. 1-16, 2016.
[CrossRef] [Google Scholar] [Publisher Link]
[17] Tom Howley et al., “The Effect of Principal Component Analysis on Machine Learning Accuracy with High Dimensional Spectral Data,” Knowledge-Based Systems, vol. 19, no. 5, pp. 363-370, 2006.
[CrossRef] [Google Scholar] [Publisher Link]
[18] Maham Saeidi et al., “Neural Decoding of EEG Signals with Machine Learning: A Systematic Review,” Brain Sciences, vol. 11, no. 11, pp. 1-44, 2021.
[CrossRef] [Google Scholar] [Publisher Link]
[19] Sagar Santaji, Snehal Santaji, and Veena Desai, “Automatic Sleep Stage Classification with Reduced Epoch of EEG,” Evolutionary Intelligence, vol. 15, pp. 2239-2246, 2022.
[CrossRef] [Google Scholar] [Publisher Link]
[20] Sagar Santaji, and Veena Desai, “Analysis of EEG Signal to Classify Sleep Stages Using Machine Learning,” Sleep and Vigilance, vol. 4, no. 2, pp. 145-152, 2020.
[CrossRef] [Google Scholar] [Publisher Link]
[21] Athar Shoka et al., “Literature Review on EEG Preprocessing, Feature Extraction, and Classifications Techniques,” Menoufia Journal of Electronic Engineering Research, vol. 28, pp. 292-299, 2019.
[CrossRef] [Google Scholar] [Publisher Link]
[22] J.S. Sørensen et al., “A Comparison of IIR and Wavelet Filtering for Noise Reduction of the ECG,” 2010 Computing in Cardiology, Belfast, UK, pp. 489-492, 2010.
[Google Scholar] [Publisher Link]
[23] Bingtao Zhang et al., “EEG-Based Automatic Sleep Staging Using Ontology and Weighting Feature Analysis,” Computational and Mathematical Methods in Medicine, vol. 2018, pp. 1-16, 2018.
[CrossRef] [Google Scholar] [Publisher Link]
[24] Manish Sharma, Virendra Patel, and U. Rajendra Acharya, “Automated Identification of Insomnia Using Optimal Bi-Orthogonal Wavelet Transform Technique with Single-Channel EEG Signals,” Knowledge-Based Systems, vol. 224, 2021.
[CrossRef] [Google Scholar] [Publisher Link]
[25] Bufang Yang, and Hongxing Liu, “Automatic Identification of Insomnia Based on Single-Channel EEG Labelled with Sleep Stage Annotations,” IEEE Access, vol. 8, pp. 104281-104291, 2020.
[CrossRef] [Google Scholar] [Publisher Link]
[26] Mostafa Shahin et al., “Deep Learning and Insomnia: Assisting Clinicians with their Diagnosis,” IEEE Journal of Biomedical and Health Informatics, vol. 21, no. 6, pp. 1546-1553, 2017.
[CrossRef] [Google Scholar] [Publisher Link]
[27] Baha Şen et al., “A Comparative Study on Classification of Sleep Stage Based on EEG Signals Using Feature Selection and Classification Algorithms,” Journal of Medical Systems, vol. 38, pp. 1-21, 2014.
[CrossRef] [Google Scholar] [Publisher Link]
[28] Igor Stancin, Mario Cifrek, and Alan Jovic, “A Review of EEG Signal Features and their Application in Driver Drowsiness Detection Systems,” Sensors, vol. 21, no. 11, pp. 1-29, 2021.
[CrossRef] [Google Scholar] [Publisher Link]
[29] Cabir Vural, and Murat Yildiz, “Determination of Sleep Stage Separation Ability of Features Extracted from EEG Signals Using Principle Component Analysis,” Journal of Medical Systems, vol. 34, pp. 83-89, 2010.
[CrossRef] [Google Scholar] [Publisher Link]
[30] Shreya Bhat et al., “Automated Diagnosis of Autism: In Search of a Mathematical Marker,” Reviews in the Neurosciences, vol. 25, no. 6, pp. 851-861, 2014.
[CrossRef] [Google Scholar] [Publisher Link]
[31] Hong-Bo Xie et al., “Complexity Analysis of the Biomedical Signal Using Fuzzy Entropy Measurement,” Applied Soft Computing, vol. 11, no. 2, pp. 2871-2879, 2011.
[CrossRef] [Google Scholar] [Publisher Link]
[32] U. Rajendra Acharya et al., “Nonlinear Dynamics Measures for Automated EEG-Based Sleep Stage Detection,” European Neurology, vol. 74, no. 5-6, pp. 268-287, 2016.
[CrossRef] [Google Scholar] [Publisher Link]
[33] You Meme Wu et al., “EEG Power during Waking and NREM Sleep in Primary Insomnia,” Journal of Clinical Sleep Medicine, vol. 9, no. 10, pp. 1031-1037, 2013.
[CrossRef] [Google Scholar] [Publisher Link]
[34] Daniel Forget, Charles M. Morin, and Clyne H. Bastien, “The Role of the Spontaneous and Evoked K-Complex in Good-Sleeper Controls and in Individuals with Insomnia,” Sleep, vol. 34, no. 9, pp. 1251-1260, 2011.
[CrossRef] [Google Scholar] [Publisher Link]
[35] Nico Surantha, Tri Fennia Lesmana, and Sani Muhamad Isa, “Sleep Stage Classification Using Extreme Learning Machine and Particle Swarm Optimization for Healthcare Big Data,” Journal of Big Data, vol. 8, pp. 1-17, 2021.
[CrossRef] [Google Scholar] [Publisher Link]
[36] Bin Ma et al., “Development of a Support Vector Machine Learning and Smart Phone Internet of Things-Based Architecture for Real-Time Sleep Apnea Diagnosis,” BMC Medical Informatics and Decision Making, vol. 20, no. 14, pp. 1-13, 2020.
[CrossRef] [Google Scholar] [Publisher Link]
[37] Jair Cervantes et al., “A Comprehensive Survey on Support Vector Machine Classification: Applications, Challenges and Trends,” Neurocomputing, vol. 408, pp. 189-215, 2020.
[CrossRef] [Google Scholar] [Publisher Link]
[38] Tarek Lajnef et al., “Learning Machines and Sleeping Brains: Automatic Sleep Stage Classification Using Decision-Tree Multi-Class Support Vector Machines,” Journal of Neuroscience Methods, vol. 250, pp. 94-105, 2015.
[CrossRef] [Google Scholar] [Publisher Link]
[39] Bahzad Taha Jijo, and Adnan Mohsin Abdulazeez, “Classification Based on Decision Tree Algorithm for Machine Learning,” Journal of Applied Science and Technology Trends, vol. 2, no. 1, pp. 20-28, 2021.
[CrossRef] [Google Scholar] [Publisher Link]
[40] Jinwen Liang et al., “Efficient and Secure Decision Tree Classification for Cloud-Assisted Online Diagnosis Services,” IEEE Transactions on Dependable and Secure Computing, vol. 18, no. 4, pp. 1632-1644, 2021.
[CrossRef] [Google Scholar] [Publisher Link]
[41] Alexander J. Boe et al., “Automating Sleep Stage Classification Using Wireless, Wearable Sensors,” NPJ Digital Medicine, vol. 2, pp. 1-9, 2019.
[CrossRef] [Google Scholar] [Publisher Link]
[42] Irena Koprinska, Gert Pfurtscheller, and Doris Flotzinger, “Sleep Classification in Infants by Decision Tree-Based Neural Networks,” Artificial Intelligence in Medicine, vol. 8, no. 4, pp. 387-401, 1996.
[CrossRef] [Google Scholar] [Publisher Link]
[43] Leo Breiman, “Random Forests,” Machine Learning, vol. 45, pp. 5-32, 2001.
[CrossRef] [Google Scholar] [Publisher Link]
[44] A.H.M.T.C. Bakmeedeniya, “Random Forest Approach for Sleep Stage Classification,” International Journal of Scientific and Research Publications, vol. 10, no. 5, pp. 768-772, 2020.
[CrossRef] [Google Scholar] [Publisher Link]
[45] Alessia Sarica, Antonio Cerasa, and Aldo Quattrone, “Random Forest Algorithm for the Classification of Neuroimaging Data in Alzheimer’s Disease: A Systematic Review,” Frontiers in Aging Neuroscience, vol. 9, pp. 1-12, 2017.
[CrossRef] [Google Scholar] [Publisher Link]
[46] Kalaivani Sundararajan et al., “Sleep Classification from Wrist-Worn Accelerometer Data Using Random Forests,” Scientific Reports, vol. 11, pp. 1-10, 2021.
[CrossRef] [Google Scholar] [Publisher Link]
[47] Thakerng Wongsirichot, and Anantaporn Hanskunatai, “A Classification of Sleep Disorders with Optimal Features Using Machine Learning Techniques,” Journal of Health Research, vol. 31, no. 3, pp. 209-217, 2017.
[CrossRef] [Google Scholar] [Publisher Link]
[48] Ozal Yildirim, Ulas Baran Baloglu, and U. Rajendra Acharya, “A Deep Learning Model for Automated Sleep Stages Classification Using PSG Signals,” International Journal of Environmental Research and Public Health, vol. 16, no. 4, pp. 1-21, 2019.
[CrossRef] [Google Scholar] [Publisher Link]
[49] Huy Phan et al., “Joint Classification and Prediction CNN Framework for Automatic Sleep Stage Classification,” IEEE Transactions on Biomedical Engineering, vol. 66, no. 5, pp. 1285-1296, 2019.
[CrossRef] [Google Scholar] [Publisher Link]
[50] Chengfan Li et al., “A Deep Learning Method Approach for Sleep Stage Classification with EEG Spectrogram,” International Journal of Environmental Research and Public Health, vol. 19, no. 10, pp. 1-17, 2022.
[CrossRef] [Google Scholar] [Publisher Link]
[51] Marina Ronzhina et al., “Sleep Scoring Using Artificial Neural Networks,” Sleep Medicine Reviews, vol. 16, no. 3, pp. 251-263, 2012.
[CrossRef] [Google Scholar] [Publisher Link]
[52] Enso Grossi, and Massimo Buscema, “Introduction to Artificial Neural Networks,” European Journal of Gastroenterology & Hepatology, vol. 19, no. 12, pp. 1046-1054, 2007.
[CrossRef] [Google Scholar] [Publisher Link]
[53] Claude Robert, Christian Guilpin, and Aymé Limoge, “Review of Neural Network Applications in Sleep Research,” Journal of Neuroscience Methods, vol. 79, no. 2, pp. 187-193, 1998.
[CrossRef] [Google Scholar] [Publisher Link]