Harnessing EEG Data to Explore Stress Reduction through Hypnosis
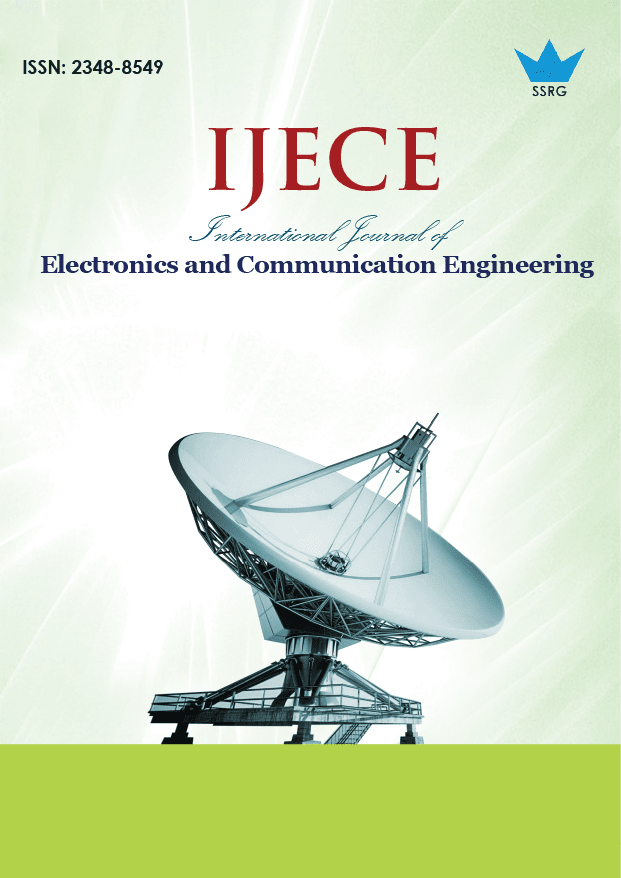
International Journal of Electronics and Communication Engineering |
© 2023 by SSRG - IJECE Journal |
Volume 10 Issue 12 |
Year of Publication : 2023 |
Authors : Swati Kamthekar, Brijesh Iyer, Prachi Deshpande, Manjiri Gokhale |
How to Cite?
Swati Kamthekar, Brijesh Iyer, Prachi Deshpande, Manjiri Gokhale, "Harnessing EEG Data to Explore Stress Reduction through Hypnosis," SSRG International Journal of Electronics and Communication Engineering, vol. 10, no. 12, pp. 83-92, 2023. Crossref, https://doi.org/10.14445/23488549/IJECE-V10I12P108
Abstract:
The rising prevalence of stress in today’s fast-paced world has incited individuals to seek complementary and alternative methods, such as yoga, meditation, and hypnosis, to find solace and peacefulness. Allopathic medicines are increasingly being shunned due to their adverse side effects. Hypnotic susceptibility assessment and stress reduction analysis are critical areas of research. To date, the hypnotic susceptibility was measured with a scale. That scale score was used for the supervised classification of the hypnotic susceptibility of the subject using EEG-like inputs. Scale scores are subjective and, hence, may be prone to errors. This paper reports a novel approach that utilizes only EEG-based evaluation of hypnotic susceptibility. The subjects classified with medium and high susceptibility were considered for stress reduction and EEG analysis using hypnosis. For hypnotic susceptibility analysis, Redefined Composite Multiscale Dispersion Entropy (RCMDE) and Multivariate Dispersion Entropy (MvDE), along with an unsupervised K-means classifier, were used. For stress analysis, alpha and beta asymmetry are used as features. The results obtained from this approach deliver valuable insights into hypnotic susceptibility and stress reduction, contributing to the advancement of EEG-based assessment methods.
Keywords:
Alpha beta asymmetry, Complementary medicine, Entropy, EEG signal, Multivariate, Stress reduction.
References:
[1] Jyoti Sekhar Banerjee, Mufti Mahmud, and David Brown, “Heart Rate Variability-Based Mental Stress Detection: An Explainable Machine Learning Approach,” SN Computer Science, vol. 4, 2023.
[CrossRef] [Google Scholar] [Publisher Link]
[2] M. Ben Ayed, “Balanced Communication-Avoiding Support Vector Machine when Detecting Epilepsy Based on EEG Signals,” Engineering, Technology and Applied Science Research, vol. 10, no. 6, pp. 6462-6468, 2020.
[CrossRef] [Google Scholar] [Publisher Link]
[3] Tee Yi Wen, and Siti Armiza Mohd Aris, “Electroencephalogram (EEG) Stress Analysis on Alpha/Beta Ratio and Theta/Beta Ratio,” Indonesian Journal of Electrical Engineering and Computer Science, vol. 17, no. 1, pp. 175-182, 2020.
[CrossRef] [Google Scholar] [Publisher Link]
[4] Ala Hag et al., “EEG Mental Stress Assessment Using Hybrid Multi-Domain Feature Sets of Functional Connectivity Network and Time-Frequency Features,” Sensors, vol. 21, no. 18, pp. 1-23, 2021.
[CrossRef] [Google Scholar] [Publisher Link]
[5] Helané Wahbeh et al., “Complementary and Alternative Medicine for Posttraumatic Stress Disorder Symptoms: A Systematic Review,” Journal of Evidence-Based Integrative Medicine, vol. 19, no. 3, pp. 161-175, 2014.
[CrossRef] [Google Scholar] [Publisher Link]
[6] Pallav Sengupta, “Health Impacts of Yoga and Pranayama: A State-of-the-Art Review,” International Journal of Preventive Medicines, vol. 3, no. 7, pp. 444-458, 2012.
[Google Scholar] [Publisher Link]
[7] Theodore X. Barber, and Wilfried de Moor, “A Theory of Hypnotic Induction Procedures,” American Journal of Clinical Hypnosis, vol. 15, no. 2, pp. 112-135, 1972.
[CrossRef] [Google Scholar] [Publisher Link]
[8] Loren Toussaint et al., “Effectiveness of Progressive Muscle Relaxation, Deep Breathing, and Guided Imagery in Promoting Psychological and Physiological States of Relaxation,” Evidence-Based Complementary and Alternative Medicine, vol. 2021, pp. 1-8, 2021.
[CrossRef] [Google Scholar] [Publisher Link]
[9] N. Zech et al., “Efficacy, Acceptability and Safety of Guided Imagery/Hypnosis in Fibromyalgia - A Systematic Review and Meta-Analysis of Randomized Controlled Trials,” European Journal of Pain, vol. 21 no. 2, pp. 217-227, 2016.
[CrossRef] [Google Scholar] [Publisher Link]
[10] Maria Hadjibalassi et al., “The Effect of Guided Imagery on Physiological and Psychological Outcomes of Adult ICU Patients: A Systematic Literature Review and Methodological Implications,” Australian Critical Care, vol. 31, no. 2, pp. 73-86, 2018.
[CrossRef] [Google Scholar] [Publisher Link]
[11] P. Lush et al., “The Sussex-Waterloo Scale of Hypnotizability (SWASH): Measuring Capacity for Altering Conscious Experience,” Neuroscience of Consciousness, vol. 2021, no. 1, pp. 1-7, 2021.
[CrossRef] [Google Scholar] [Publisher Link]
[12] John C. Ruch, Arlene H. Morgan, and Ernest R. Hilgard, “Measuring Hypnotic Responsiveness: A Comparison of the Barber Suggestibility Scale and the Stanford Hypnotic Susceptibility Scale, Form A,” International Journal of Clinical and Experimental Hypnosis, vol. 22, no. 4, pp. 365-376, 1974.
[CrossRef] [Google Scholar] [Publisher Link]
[13] Golnaz Baghdadi, and Ali Motie Nasrabadi, “Comparison of Different EEG Features in the Estimation of Hypnosis Susceptibility Level,” Computers in Biology and Medicines, vol. 42, no. 5, pp. 590-597, 2012.
[CrossRef] [Google Scholar] [Publisher Link]
[14] N.V. Kimmatkar, and B. Vijaya Babu, “Human Emotion Detection with Electroencephalography Signals and Accuracy Analysis Using Feature Fusion Techniques and a Multimodal Approach for Multiclass Classification,” Engineering, Technology & Applied Science Research, vol. 12, no. 4, pp. 9012-9017, 2022.
[CrossRef] [Google Scholar] [Publisher Link]
[15] Elahe’ Yargholi, and Ali Motie Nasrabadi, “The Impacts of Hypnotic Susceptibility on Chaotic Dynamics of EEG Signals during Standard Tasks of Waterloo-Stanford Group Scale,” Journal of Medical Engineering & Technology, vol. 37, no. 4, pp. 273-281, 2013.
[CrossRef] [Google Scholar] [Publisher Link]
[16] Riccardo Chiarucci et al., “Cross-Evidence for Hypnotic Susceptibility through Nonlinear Measures on EEGs of Non-Hypnotized Subjects,” Scientific Reports, vol. 4, no. 1, 2014.
[CrossRef] [Google Scholar] [Publisher Link]
[17] Graham A. Jamieson, and Adrian P. Burgess, “Hypnotic Induction is Followed by State-Like Changes in the Organization of EEG Functional Connectivity in the Theta and Beta Frequency Bands in High-Hypnotically Susceptible Individuals,” Frontiers in Human Neuroscience, vol. 8, pp. 1-11, 2014.
[CrossRef] [Google Scholar] [Publisher Link]
[18] P.N. Smyrlis, D.C. Tsouros, and M.G. Tsipouras, “Constrained K-Means Classification,” Engineering, Technology & Applied Science Research, vol. 8, no. 4, pp. 3203-3208, 2018.
[CrossRef] [Google Scholar] [Publisher Link]
[19] Emad Alyan, Naufal M. Saad, and Nidal Kamel, “Effects of Workstation Type on Mental Stress: FNIRS Study,” Human Factors: The Journal of the Human Factors and Ergonomics Society, vol. 63, no. 7, pp. 1230-1255, 2021.
[CrossRef] [Google Scholar] [Publisher Link]
[20] Sanay Muhammad Umar Saeed et al., “EEG Based Classification of Long-Term Stress Using Psychological Labeling,” Sensors, vol. 20, no. 7, pp. 1-15, 2020.
[CrossRef] [Google Scholar] [Publisher Link]
[21] Emad Alyan et al., “Frontal Electroencephalogram Alpha Asymmetry during Mental Stress Related to Workplace Noise,” Sensors, vol. 21, no. 6, pp. 1-12, 2021.
[CrossRef] [Google Scholar] [Publisher Link]
[22] Jan Moritz Fischer et al., “Stress Reduction by Yoga Versus Mindfulness Training in Adults Suffering from Distress: A Three-Armed Randomized Controlled Trial Including Qualitative Interviews (RELAX Study),” Journal of Clinical Medicine, vol. 11, no. 19, pp. 1-23, 2022.
[CrossRef] [Google Scholar] [Publisher Link]
[23] Marco Capó, Aritz Pérez, and Jose A. Lozano, “An Efficient K-Means Clustering Algorithm for Tall Data,” Data Mining and Knowledge Discovery, vol. 34, no. 3, pp. 776-811, 2020.
[CrossRef] [Google Scholar] [Publisher Link]
[24] Mehlika Saraç, A Literature Review on Mindfulness at Work Places: Conceptualization, Measurement, and Outcomes, Handbook of Research on Positive Organizational Behavior for Improved Workplace Performance, IGI Global, pp. 55-71, 2020.
[CrossRef] [Google Scholar] [Publisher Link]
[25] Asma Baghdadi, Yassine Aribi, and Adel M. Alimi, “Efficient Human Stress Detection System Based on Frontal Alpha Asymmetry,” International Conference on Neural Information Processing: Neural Information Processing, pp. 858-867, 2017.
[CrossRef] [Google Scholar] [Publisher Link]
[26] Maryam Alimardani et al., “Classification of EEG Signals for a Hypnotrack BCI System,” 2018 IEEE/RSJ International Conference on Intelligent Robots and Systems (IROS), Madrid, Spain, pp. 240-245, 2018.
[CrossRef] [Google Scholar] [Publisher Link]
[27] H. Norhazman et al., “Behaviour of EEG Alpha Asymmetry when Stress is Induced and Binaural Beat is Applied,” 2012 International Symposium on Computer Applications and Industrial Electronics (ISCAIE), Kota Kinabalu, Malaysia, pp. 297-301, 2012.
[CrossRef] [Google Scholar] [Publisher Link]