Enhancing Parkinson’s Disease Prognosis with LSTM-Based Deep Learning for Precision Diagnosis and Symptom Trajectory Analysis
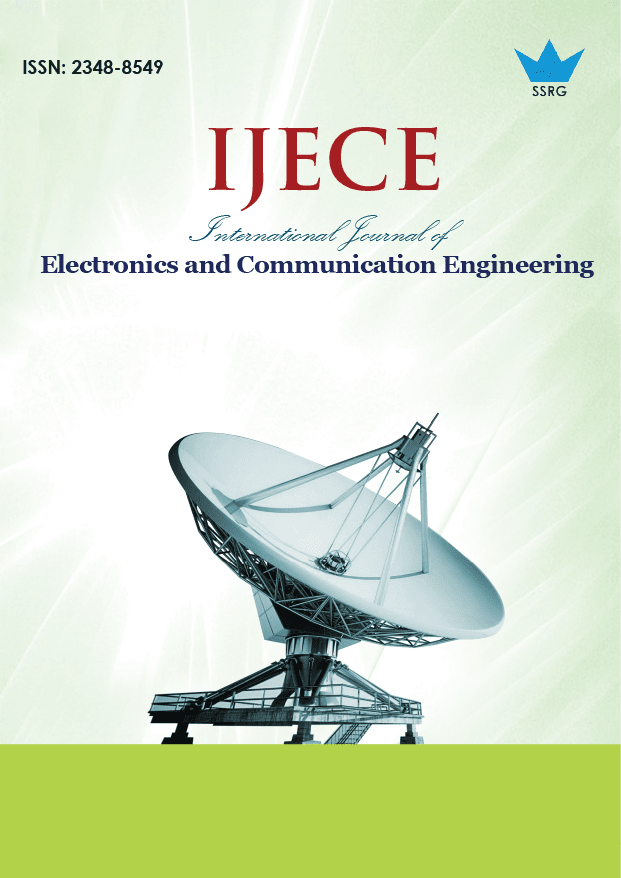
International Journal of Electronics and Communication Engineering |
© 2024 by SSRG - IJECE Journal |
Volume 11 Issue 1 |
Year of Publication : 2024 |
Authors : S.V.V.D. Venu Gopal, Sanam Siva Ramaraja, Kalli Srinivasa Nageswara Prasad, Viswaprasad Kasetti |
How to Cite?
S.V.V.D. Venu Gopal, Sanam Siva Ramaraja, Kalli Srinivasa Nageswara Prasad, Viswaprasad Kasetti, "Enhancing Parkinson’s Disease Prognosis with LSTM-Based Deep Learning for Precision Diagnosis and Symptom Trajectory Analysis," SSRG International Journal of Electronics and Communication Engineering, vol. 11, no. 1, pp. 53-66, 2024. Crossref, https://doi.org/10.14445/23488549/IJECE-V11I1P105
Abstract:
This study develops a novel prognostic model for Parkinson’s Disease (PD) based on an LSTM network. PD is one of the most common neurodegenerative disorders. To overcome these limitations of traditional PD analysis models, our approach dramatically increases accuracy (90.00%), precision (94.85%), and recall (85.98%). Using patient-specific data, including genetics and lifestyle information along with detailed symptomatology, the model creates an individualised analysis for each patient’s particular manifestation of PD with its ability to process time-series data and handle non-stationary processes, the robust LSTM network can produce a rich characterisation of how PD symptoms develop over time. The model’s effectiveness is further enhanced by its stringent performance indicators, including an F1 Score of 90.20% and an AUC-ROC of 93.79%, indicating greater precision in prediction, especially during the early stages before progressing toward full PD For healthcare diagnostics and PD management, this breakthrough promises to be a game- The study presents a new standard for disease management and patient care. It provides healthcare providers with an accountable, personalised, and flexible diagnostic tool for PD assessment.
Keywords:
Parkinson’s Disease prognosis, LSTM Deep Learning, Patient-centric data analysis, Symptom trajectory modeling, AI in neurological disorders, Precision healthcare diagnostics.
References:
[1] Eduardo Tolosa et al., “Challenges in the Diagnosis of Parkinson’s Disease,” The Lancet Neurology, vol. 20, no. 5, pp. 385-397, 2021.
[CrossRef] [Google Scholar] [Publisher Link]
[2] Anthony E. Lang et al., “A Critical Appraisal of the Premotor Symptoms of Parkinson’s Disease: Potential Usefulness in Early Diagnosis and Design of Neuroprotective Trials,” Movement Disorders, vol. 26, no. 5, pp. 775-783, 2011.
[CrossRef] [Google Scholar] [Publisher Link]
[3] Nafiseh Ghaffar Nia, Erkan Kaplanoglu, and Ahad Nasab, “Evaluation of Artificial Intelligence Techniques in Disease Diagnosis and Prediction,” Discover Artificial Intelligence, vol. 3, 2023.
[CrossRef] [Google Scholar] [Publisher Link]
[4] Balaji E. et al., “Automatic and Non-Invasive Parkinson’s Disease Diagnosis and Severity Rating Using LSTM Network,” Applied Soft Computing, vol. 108, 2021.
[CrossRef] [Google Scholar] [Publisher Link]
[5] Lydia Chougar et al., “The Role of Magnetic Resonance Imaging for the Diagnosis of Atypical Parkinsonism,” Frontiers in Neurology, vol. 11, pp. 1-17, 2020.
[CrossRef] [Google Scholar] [Publisher Link]
[6] Beatriz Garcia Santa Cruz, Andreas Husch, and Frank Hertel, “Machine Learning Models for Diagnosis and Prognosis of Parkinson’s Disease Using Brain Imaging: General Overview, Main Challenges, and Future Directions,” Frontiers in Aging Neuroscience, vol. 15, pp. 1-20, 2023.
[CrossRef] [Google Scholar] [Publisher Link]
[7] P. Pradeep, and Kamalakannan J., “Effective Predictor Model for Parkinson’s Disease Using Machine Learning,” International Journal of Computer Engineering in Research Trends, vol. 10, no. 4, pp. 204-209, 2023.
[Publisher Link]
[8] Robert Petersen et al., “Insights in Parkinson’s Disease and Aging-Related Movement Disorders: 2022,” Frontiers in Aging Neuroscience, vol. 103, 2023.
[Publisher Link]
[9] Gennaro Pagano, Flavia Niccolini, and Marios Politis, “Imaging in Parkinson’s Disease,” Clinical Medicine Journal, vol. 16, no. 4, pp. 371-375, 2016.
[CrossRef] [Google Scholar] [Publisher Link]
[10] Mary B. Makarious et al., “Multi-Modality Machine Learning Predicting Parkinson’s Disease,” npj Parkinson’s Disease, vol. 8, pp. 1- 13, 2022.
[CrossRef] [Google Scholar] [Publisher Link]
[11] Gayatri Khanvilkar, and Deepali Vora, “Activation Functions and Training Algorithms for Deep Neural Network,” International Journal of Computer Engineering in Research Trends, vol. 5, no. 4, pp. 98-104, 2018.
[Google Scholar] [Publisher Link]
[12] Massimo Salvi et al., “Multi-Modality Approaches for Medical Support Systems: A Systematic Review of the Last Decade,” Information Fusion, vol. 103, 2024.
[CrossRef] [Google Scholar] [Publisher Link]
[13] Timothy P. Lillicrap, and Adam Santoro, “Backpropagation through Time and the Brain,” Current Opinion in Neurobiology, vol. 55, pp. 82-89, 2019.
[CrossRef] [Google Scholar] [Publisher Link]
[14] Anuj Karpatne, Ramakrishnan Kannan, and Vipin Kumar, Knowledge Guided Machine Learning: Accelerating Discovery Using Scientific Knowledge and Data, 1 st ed., CRC Press, 2022.
[Google Scholar] [Publisher Link]
[15] Accountability Act, “Health Insurance Portability and Accountability Act of 1996,” Public Law, vol. 104, 1996.
[Google Scholar] [Publisher Link]
[16] Movement Disorder Society Task Force on Rating Scales for Parkinson’s Disease, “The Unified Parkinson’s Disease Rating Scale (UPDRS): Status and Recommendations,” Movement Disorders, vol. 18, no. 7, pp. 738-750, 2003.
[CrossRef] [Google Scholar] [Publisher Link]
[17] Nalini M. Singh et al., “How Machine Learning is Powering Neuroimaging to Improve Brain Health,” Neuroinformatics, vol. 20, pp. 943-964, 2022.
[CrossRef] [Google Scholar] [Publisher Link]
[18] Thomas Welton et al., “Essential Tremor,” Nature Reviews Disease Primers, vol. 7, 2021.
[CrossRef] [Google Scholar] [Publisher Link]
[19] Tarannom Parhizkar, Elham Rafieipour, and Aram Parhizkar, “Evaluation and Improvement of Energy Consumption Prediction Models Using Principal Component Analysis Based Feature Reduction,” Journal of Cleaner Production, vol. 279, 2021.
[CrossRef] [Google Scholar] [Publisher Link]
[20] Reza Moradi, Reza Berangi, and Behrouz Minaei, “A Survey of Regularization Strategies for Deep Models,” Artificial Intelligence Review, vol. 53, pp. 3947-3986, 2020.
[CrossRef] [Google Scholar] [Publisher Link]