A Comprehensive Stop-Word Compilation for Kannada Language Processing
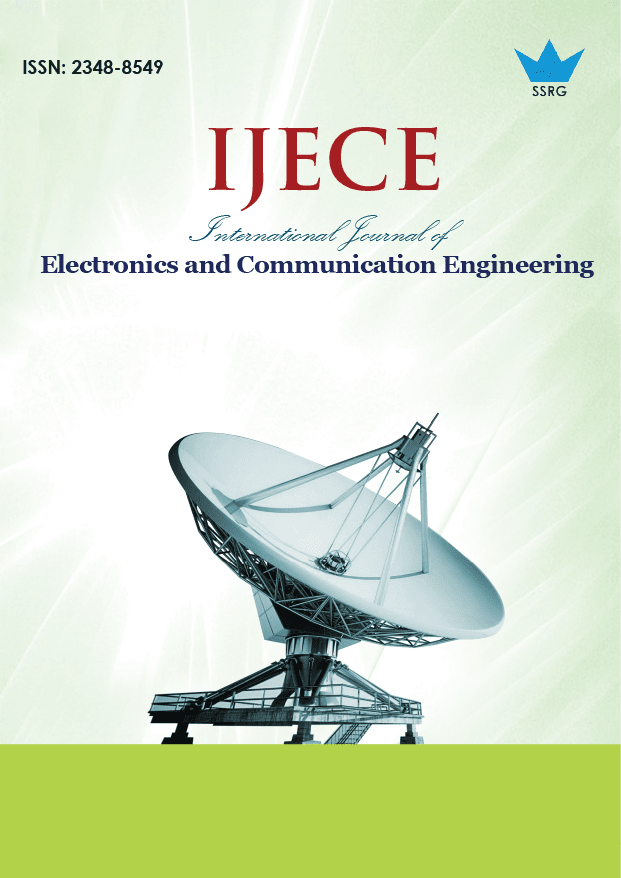
International Journal of Electronics and Communication Engineering |
© 2024 by SSRG - IJECE Journal |
Volume 11 Issue 2 |
Year of Publication : 2024 |
Authors : M.S. Sowmya, M.V. Panduranga Rao |
How to Cite?
M.S. Sowmya, M.V. Panduranga Rao, "A Comprehensive Stop-Word Compilation for Kannada Language Processing," SSRG International Journal of Electronics and Communication Engineering, vol. 11, no. 2, pp. 69-79, 2024. Crossref, https://doi.org/10.14445/23488549/IJECE-V11I2P108
Abstract:
In this work, a vital aspect of Kannada Natural Language Processing (NLP) takes the stage, with the construction of a standardized stop-word list emerging as a pioneering endeavor. This essential list serves as a foundation for improving language comprehension and processing activities. The work offers a rigorous technique that includes data gathering, tokenization, and TF-IDF score computation using the IndicCorp Kannada dataset. The study innovatively pioneers the construction of a stop-word list exclusively designed for the Kannada language, a first in this domain. The findings highlight the significance of these stop words and their prospective applications in diverse NLP endeavors, providing the framework for the upcoming construction of a Kannada-specific text summarizing work. The human refinement procedure ensures precision in stop-word compilation while considering inherent subjectivity and dataset-specific restrictions. Importantly, this study not only gives valuable insights into linguistic characteristics but also pioneers an innovative approach for stop-word generation in Kannada, establishing itself as a pioneering effort in this specific area of research. Furthermore, the study goes beyond its immediate findings by offering methodologies for the automated compilation and validation of stop words, thus laying the groundwork for further research. This foresight adds to the ongoing advancement of Kannada NLP methods.
Keywords:
Text summarization, Natural Language Processing, Stop words, BERTsum, Kannada language.
References:
[1] G. Trishala, and H.R. Mamatha, “Implementation of Stemmer and Lemmatizer for a Low-Resource Language-Kannada,” Proceedings of International Conference on Intelligent Computing, Information and Control Systems, pp. 345-358, 2021.
[CrossRef] [Google Scholar] [Publisher Link]
[2] Walaa Medhat, Ahmed H. Yousef, and Hoda Korashy, “Corpora Preparation and Stopword List Generation for Arabic Data in Social Network,” arXiv, pp. 1-15, 2014.
[CrossRef] [Google Scholar] [Publisher Link]
[3] Bernard Masua, and Noel Masasi, “Enhancing Text Pre-Processing for Swahili Language: Datasets for Common Swahili Stop-Words, Slangs and Typos with Equivalent Proper Words,” Data in Brief, vol. 33, 2020.
[CrossRef] [Google Scholar] [Publisher Link]
[4] Ayanouz Soufyane, Boudhir Anouar Abdelhakim, and Mohamed Ben Ahmed, “An Intelligent Chatbot Using NLP and TF-IDF Algorithm for Text Understanding Applied to the Medical Field,” Emerging Trends in ICT for Sustainable Development, pp. 3-10, 2021.
[CrossRef] [Google Scholar] [Publisher Link]
[5] Ruby Rani, and D.K. Lobiyal, “Performance Evaluation of Text-Mining Models with Hindi Stopwords Lists,” Journal of King Saud University - Computer and Information Sciences, vol. 34, no. 6, pp. 2771-2786, 2022.
[CrossRef] [Google Scholar] [Publisher Link]
[6] Yaohou Fan, Chetan Arora, and Christoph Treude, “Stop Words for Processing Software Engineering Documents: Do they Matter?,” 2023 IEEE/ACM 2nd International Workshop on Natural Language-Based Software Engineering (NLBSE), Melbourne, Australia, pp. 40-47, 2023.
[CrossRef] [Google Scholar] [Publisher Link]
[7] Ruby Rani, and D.K. Lobiyal, “Automatic Construction of Generic Stop Words List for Hindi Text,” Procedia Computer Science, vol. 132, pp. 362-370, 2018.
[CrossRef] [Google Scholar] [Publisher Link]
[8] Stefano Ferilli, “Automatic Multilingual Stopwords Identification from Very Small Corpora,” Electronics, vol. 10, no. 17, pp. 1-21, 2021.
[CrossRef] [Google Scholar] [Publisher Link]
[9] Elena Campillo-Ageitos et al., “NLP-UNED at eRisk 2021: Self-Harm Early Risk Detection with TF-IDF and Linguistic Features,” CLEF 2021 – Conference and Labs of the Evaluation Forum, Bucharest, Romania, pp. 1-16, 2021.
[Google Scholar] [Publisher Link]
[10] D. Dessı et al., “TF-IDF vs Word Embeddings for Morbidity Identification in Clinical Notes: An Initial Study,” Zenodo (CERN European Organization for Nuclear Research), 2020.
[Google Scholar] [Publisher Link]
[11] Muhammad Ibnu Alfarizi, Lailis Syafaah, and Merinda Lestandy, “Emotional Text Classification Using TF-IDF (Term Frequency-Inverse Document Frequency) and LSTM (Long Short-Term Memory),” Journal of Informatics: Juita, vol. 12, no. 2, pp. 225-232, 2022.
[CrossRef] [Google Scholar] [Publisher Link]
[12] Sheher Bano et al., “Summarization of Scholarly Articles Using BERT and BiGRU: Deep Learning-Based Extractive Approach,” Journal of King Saud University - Computer and Information Sciences, vol. 35, no. 9, 2023.
[CrossRef] [Google Scholar] [Publisher Link]
[13] Junqing Fan et al., “Extractive Social Media Text Summarization Based on MFMMR-BertSum,” Array, vol. 20, pp. 1-7, 2023.
[CrossRef] [Google Scholar] [Publisher Link]
[14] Betul Ay et al., “Turkish Abstractive Text Document Summarization Using Text to Text Transfer Transformer,” Alexandria Engineering Journal, vol. 68, pp. 1-13, 2023.
[CrossRef] [Google Scholar] [Publisher Link]
[15] Hao Liu, Yehoshua Perl, and James Geller, “Concept Placement Using BERT Trained by Transforming and Summarizing Biomedical Ontology Structure,” Journal of Biomedical Informatics, vol. 112, pp. 1-13, 2020.
[CrossRef] [Google Scholar] [Publisher Link]
[16] Qianqian Xie et al., “Pre-Trained Language Models with Domain Knowledge for Biomedical Extractive Summarization,” Knowledge-Based Systems, vol. 252, pp. 1-12, 2022.
[CrossRef] [Google Scholar] [Publisher Link]
[17] Imen Tanfouri, and Fethi Jarray, “GaSUM: A Genetic Algorithm Wrapped BERT for Text Summarization,” Proceedings of the 15th International Conference on Agents and Artificial Intelligence, Lisbon, Portugal, vol. 2, pp. 447-453, 2023.
[CrossRef] [Google Scholar] [Publisher Link]
[18] Max Grusky, “Rogue Scores,” Proceedings of the 61st Annual Meeting of the Association for Computational Linguistics, Toronto, Canada, vol. 1, pp. 1914-1934, 2023.
[CrossRef] [Google Scholar] [Publisher Link]
[19] Mousumi Akter, Naman Bansal, and Shubhra Kanti Karmaker, “Revisiting Automatic Evaluation of Extractive Summarization Task: Can We Do Better than Rouge?,” Findings of the Association for Computational Linguistics: ACL 2022, Dublin, Ireland, pp. 1547-1560, 2022.
[CrossRef] [Google Scholar] [Publisher Link]
[20] Marcello Barbella, and Genoveffa Tortora, “Rouge Metric Evaluation for Text Summarization Techniques,” SSRN Electronic Journal, pp. 1-31, 2022.[CrossRef] [Google Scholar] [Publisher Link]