Certain Investigation of the Retinal Hemorrhage Detection in Fundus Images
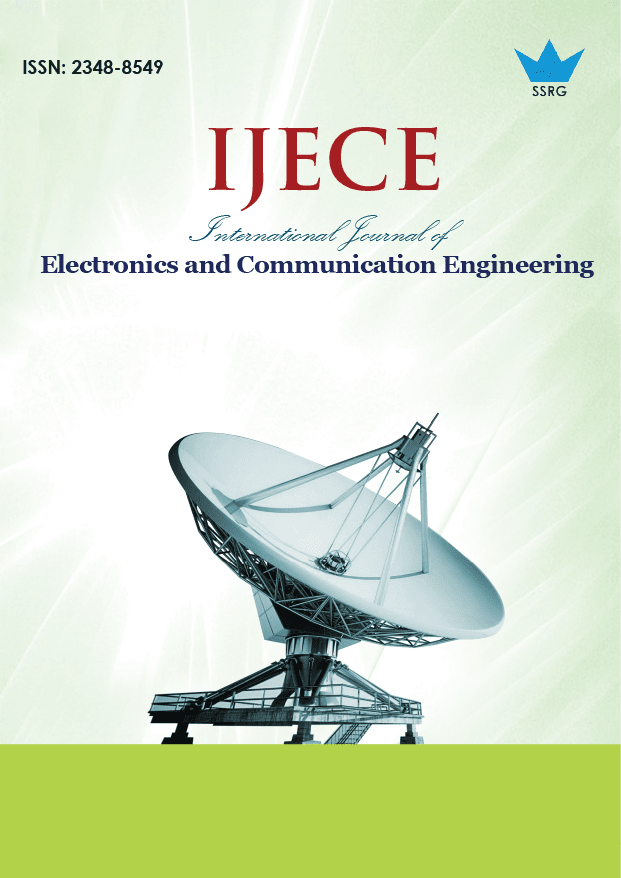
International Journal of Electronics and Communication Engineering |
© 2015 by SSRG - IJECE Journal |
Volume 2 Issue 2 |
Year of Publication : 2015 |
Authors : Ms.S.Deepa and Mr.S.Vijayprasath |
How to Cite?
Ms.S.Deepa and Mr.S.Vijayprasath, "Certain Investigation of the Retinal Hemorrhage Detection in Fundus Images," SSRG International Journal of Electronics and Communication Engineering, vol. 2, no. 2, pp. 24-34, 2015. Crossref, https://doi.org/10.14445/23488549/IJECE-V2I2P106
Abstract:
Diabetic Retinopathy has become a common eye disease in most of the developed countries. It leads to damage of the retina, since fluid leaks from blood vessels into the retina. The presence of hemorrhages in the retina is the primary symptom of diabetic retinopathy. The number and shape of hemorrhages is used to indicate the severity of the disease. Early automated hemorrhage detection can help reduce the occurrence of blindness.Reliable detection of large retinal hemorrhages is important in the development of automated screening systems, which can be translated into practice. Our proposed work is to detect the large retinal hemorrhage detection part based on splat feature classification.Using fundus photographs the images are partitioned into number of splats. Each splat contains pixels with similar color and close spatial location. A set of distinct features was extracted within each splat. Following this, different features are extracted which serves as the guideline to identify and grade the severity of the disease. Based on the extracted features classification, the retinal image is distinguished as normal or abnormal using matlab codings.
Keywords:
Diabetic Retinopathy (DR), Fundus image, Retinal Hemorrhage, Splat feature classification.
References:
[1] O. Faust, R. Acharya U., E. Y. K. Ng, K.-H. Ng, and J. S. Suri, “Algorithms for the automated detection of diabetic retinopathy using digital fundus images: A review,” J. Med. Syst., Apr2010 .
[2] M. Niemeijer, M. D. Abramoff, and B. van Ginneken, “Information fusion for diabetic retinopathy CAD in digital color fundus photographs,” IEEE Trans. Med. Imag., no. 5, pp. 775–785, May 2009.
[3] M. Niemeijer, B. van Ginneken, J. Staal, M. S. A. Suttorp-Schulten, and M. D. Abràmoff, “Automatic detection of red lesions in digital color fundus photographs,” IEEE Trans. Med. Imag., vol. 24, no. 5, 584-592,May 2005.
[4] G. Quellec, S. Russell, and M. Abràmoff, “Optimal filter framework for automated, instantaneous detection of lesions in retinal images,” IEEE Trans. Med. Imag., vol. 30, no. 2, pp. 523–533, Feb. 2011.
[5] Y. Hatanaka, T. Nakagawa, Y. Hayashi, M. Kakogawa, A. Sawada, K. Kawase, T. Hara, and H. Fujita, “Improvement of automatic hemorrhages detection methods using brightness correction on fundus images,” in Proc. SPIE, 2008, vol. 6915, pp. 69 153E-1–69 153E-10.
[6] P. Jitpakdee, P. Aimmanee, and B. Uyyanonvara, “A survey on hemorrhage detection in diabetic retinopathy retinal images,” in Proc. 9th Int. Conf. Elect. Eng./Electron., Comput., Telecommun. Inf. Technol. (ECTI-CON), Bangkok, Thailand, 2012, pp. 1–4, vol.2.
[7] M. Abràmoff, M. Garvin, and M. Sonka, “Retinal imaging and image analysis,” IEEE Rev. Biomed. Eng., vol. 3, pp. 169–208, 2010.
[8] S. C. H. Hoi, R. Jin, J. Zhu, and M. R. Lyu, “Batch mode active learning and its application to medical image classification,” in Proc. ICML, 2006, pp. 417–424.
[9] Fairfield, “Toboggan contrast enhancem ent for contrast segmentation,” in Proc. Int. Conf. Pattern Recognit., 1990, vol. 1, pp. 712–716.
[10] N. V. Chawla, N. Japkowicz, and A. Kotcz, “Editorial: Special issue on learning from imbalanced data sets,” SIGKDD Explorations, no. 1, 1–6, 2004.
[11] C. L. Zitnick and S. B. Kang, “Stereo for image-based rendering using image over segmentation,” Int. J. Comput. Vis., no. 1, pp. 49–65, Feb. 2003.
[12] X. Ren and J. Malik, “Learning a classification model for segmentation,” in Int. Conf. Comput. Vis., 2003, vol. 1.
[13] Y.-C. Lin, Y.-P. Tsai, Y.-P. Hung, and Z.-C. Shih, “Comparison between immersionbased and toboggan based watershed image segmentation,” IEEE Trans. Image Process., no. 3, pp. 632–40, Mar.2006.
[14] G. Li, G. Liqun, P. Zhao-Yu, and W. Kun, “Image segmentation using multiscale gradient toboggan,” in Proc. 2nd IEEE Conf. Ind. Electron. Appl., May 2007, pp. 2206–2209.
[15] G. Quellec, M. Lamard, M. Abràmoff, E. Decencière, B. Lay, A. Erginay, B. Cochener, and G. Cazuguel, “A multiple instance learning framework for diabetic retinopathy screening,” Med. Image Anal, vol. 16, no. 6, pp. 1228–40, 2012.
[16] M. Christopher, D. C. Moga, S. R. Russell, J. C. Folk, T. Scheetz, and M. Abràmoff, “Validation of tablet-based evaluation of color fundus images,” Retina, vol. 32, no. 8, pp. 1.629–35,2003.
[17] M. Niemeijer, M. D. Abràmoff, and B. van Ginneken, “Fast detection of the optic disc and fovea in color fundus photographs,” Med. Image Anal., no. 6, pp. 859–870, Dec.2004.
[18] M. D. Abràmoff, W. L. M. Alward, E. C. Greenlee, L. Shuba, C. Y. Kim, J. H. Fingert, and Y. H. Kwon, “Automated segmentation of the optic disc from stereo color photographs using physiologically plausible features,” Invest. Ophthalmol. Vis. Sci., vol. 48, no. 4, pp. Apr. 2007.
[19] B. M. T. H. Romeny, Front-End Vision and Multi-Scale Image Anal-ysis: Multi-Scale Computer Vision Theory and Applications, Written in Mathematica. Berlin, Germany: Springer, 2003.
[20] L. Tang, M. Niemeijer, and M. Abràmoff, “Splat feature classification: Detection of the presence of large retinal hemorrhages,” in Proc. IEEE 8th Int. Symp. Biomed. Imag. (ISBI), 2011, pp. 681–684.
[21] M. Varma and A. Zisserman, “A statistical approach to texture classi-fication from single images,” Int. J. Comput. Vis., vol. 62, no. 1–2, pp. 61–81, 2005.
[22] T. Wagner, , B. Jahne, H. Haussecker, and P. Geissler, Eds., Texture Analysis. New York: Academic, 1999, vol. 2. 2005.