Leveraging Machine Learning to Predict COVID-19 Vaccination Adoption among Healthcare Professionals in Somalia: A Comparative Analysis
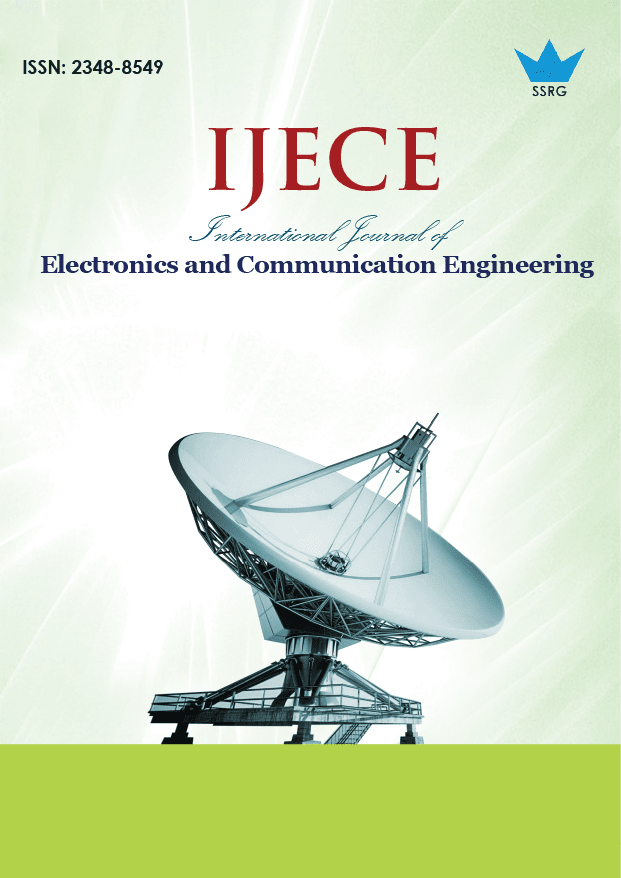
International Journal of Electronics and Communication Engineering |
© 2024 by SSRG - IJECE Journal |
Volume 11 Issue 2 |
Year of Publication : 2024 |
Authors : Mohamed Abdirahman Addow, Abdikadir Hussein Elmi |
How to Cite?
Mohamed Abdirahman Addow, Abdikadir Hussein Elmi, "Leveraging Machine Learning to Predict COVID-19 Vaccination Adoption among Healthcare Professionals in Somalia: A Comparative Analysis," SSRG International Journal of Electronics and Communication Engineering, vol. 11, no. 2, pp. 103-113, 2024. Crossref, https://doi.org/10.14445/23488549/IJECE-V11I2P111
Abstract:
This study explores the application of Machine Learning (ML) techniques to predict COVID-19 vaccination adoption among healthcare professionals in Somalia. Recognizing the pivotal role of vaccination in controlling the pandemic, and the unique challenges faced by Somalia’s healthcare system, this research aims to identify critical factors influencing vaccine uptake and to develop robust predictive models. We employed a rich dataset comprising demographic, health, and institutional variables gathered from a survey of healthcare workers across various Somali states. Three ML algorithms were evaluated: Logistic Regression, Random Forest, and Gradient Boosting. Each model was rigorously assessed using precision, recall, F1-score, and accuracy metrics. Gradient Boosting emerged as the most effective model, demonstrating the highest accuracy (approximately 85%) and F1 score (about 82%). Logistic Regression provided a baseline for comparison, while Random Forest showed notable strengths in certain aspects, particularly in feature importance analysis. The study also involved a detailed examination of confusion matrices for each model, revealing specific strengths and weaknesses in predictive capabilities. These matrices provided insights into the models’ performance, particularly in distinguishing between different vaccination behavior categories. Our findings suggest that ML can be a powerful tool in predicting vaccine adoption, with significant implications for public health strategies. The Gradient Boosting model, in particular, shows promise for practical application in designing targeted interventions to improve vaccination rates among healthcare workers. This research contributes to the growing body of knowledge in public health informatics, offering a novel approach to tackling vaccine hesitancy and enhancing pandemic response efforts in Somalia and similar settings.
Keywords:
Machine Learning, COVID-19, Vaccine adoption, Healthcare Professionals, Somalia, Public health.
References:
[1] Ioannis Alexandros Charitos et al., “Update on COVID-19 and Effectiveness of a Vaccination Campaign in a Global Context,” International Journal of Environmental Research and Public Health, vol. 19, no. 17, pp. 1-20, 2022.
[CrossRef] [Google Scholar] [Publisher Link]
[2] Jack Mewhirter, Mustafa Sagir, and Rebecca Sanders, “Towards a Predictive Model of COVID-19 Vaccine Hesitancy among American Adults,” Vaccine, vol. 40, no. 12, pp. 1783-1789, 2022.
[CrossRef] [Google Scholar] [Publisher Link]
[3] Gretchen B. Chapman, and Elliot J. Coups, “Predictors of Influenza Vaccine Acceptance among Healthy Adults,” Preventive Medicine, vol. 29, no. 4, pp. 249-262, 1999.
[CrossRef] [Google Scholar] [Publisher Link]
[4] Bruna Aparecida Souza Machado et al., “The Importance of Vaccination in the Context of the COVID-19 Pandemic: A Brief Update Regarding the Use of Vaccines,” Vaccines, vol. 10, no. 4, pp. 1-25, 2022.
[CrossRef] [Google Scholar] [Publisher Link]
[5] Jana L. Jacobs, Ghady Haidar, and John W. Mellors, “COVID-19: Challenges of Viral Variants,” Annual Review of Medicine, vol. 74, pp. 31-53, 2023.
[CrossRef] [Google Scholar] [Publisher Link]
[6] Emmanuel O. Njoga et al., “Persisting Vaccine Hesitancy in Africa: The Whys, Global Public Health Consequences and Ways-Out-COVID-19 Vaccination Acceptance Rates as Case-in-Point,” Vaccines, vol. 10, no. 11, pp. 1-23, 2022.
[CrossRef] [Google Scholar] [Publisher Link]
[7] Alexandra Savinkina et al., “Estimating Deaths Averted and Cost per Life Saved by Scaling up mRNA COVID-19 Vaccination in Low-Income and Lower-Middle-Income Countries in the COVID-19 Omicron Variant Era: A Modelling Study,” BMJ Open, vol. 12, no. 9, pp. 1-7, 2022.
[CrossRef] [Google Scholar] [Publisher Link]
[8] Maryam Diarra et al., “Non-Pharmaceutical Interventions and COVID-19 Vaccination Strategies in Senegal: A Modelling Study,” BMJ Global Health, vol. 7, no. 2, pp. 1-9, 2022.
[CrossRef] [Google Scholar] [Publisher Link]
[9] Mohammed A.M. Ahmed et al., “Covid-19 Vaccine Acceptability and Adherence to Preventive Measures in Somalia: Results of an Online Survey,” Vaccines, vol. 9, no. 6, pp. 1-11, 2021.
[CrossRef] [Google Scholar] [Publisher Link]
[10] Ayan Aden Moussa, Abdkeren Abdullahi Abdi, and Sharif Alhassan Abdullahi, “Assessment of Knowledge, Attitude and Practice of Healthcare Workers towards Hepatitis B Virus Infection in Mogadishu, Somalia: A Cross-Sectional Study,” Research Square, pp. 1-11, 2020.
[CrossRef] [Google Scholar] [Publisher Link]
[11] Mark McEvoy et al., “Loddon Mallee Healthcare Worker COVID-19 Study - Protocol for a Prospective Cohort Study Examining the Health and Well-Being of Rural Australian Healthcare Workers during the COVID-19 Pandemic,” BMJ Open, vol. 11, no. 8, pp. 1-12, 2021.
[CrossRef] [Google Scholar] [Publisher Link]
[12] J. Bryan Sexton, and Kathryn C. Adair, “Forty-Five Good Things: A Prospective Pilot Study of the Three Good Things Well-Being Intervention in the USA for Healthcare Worker Emotional Exhaustion, Depression, Work-Life Balance and Happiness,” BMJ Open, vol. 9, no. 3, 2019.
[CrossRef] [Google Scholar] [Publisher Link]
[13] Frances Kelly et al., “Improving Healthcare Worker Resilience and Well-Being during COVID-19 Using a Self-Directed E-Learning Intervention,” Frontiers in Psychology, vol. 12, pp. 1-9, 2021.
[CrossRef] [Google Scholar] [Publisher Link]
[14] Vivek Raj S.N., and Manivannan S.K., “Machine Learning Models to Predict Covid-19 Vaccination Intention : An Indian Study,” International Journal of Professional Business Review, vol. 7, no. 6, pp. 1-16, 2022.
[CrossRef] [Google Scholar] [Publisher Link]
[15] Alinoor Mohamed Farah et al., “Knowledge, Attitudes, and Practices Regarding COVID-19 among Health Care Workers in Public Health Facilities in Eastern Ethiopia: Cross-Sectional Survey Study,” JMIR Formative Research, vol. 5, no. 10, pp. 1-12, 2021.
[CrossRef] [Google Scholar] [Publisher Link]
[16] Said Adam Sheikh et al., “Infection Prevention Practice and Associated Factors among Health Care Workers in Public Health Facilities of Mogadishu, Somalia, 2022,” Archiv Euromedica, vol. 13, no. 3, pp. 1-19, 2023.
[Google Scholar]
[17] Hassan Abdullahi Dahie et al., “COVID-19 Vaccine Coverage and Potential Drivers of Vaccine Uptake among Healthcare Workers in Somalia: A Cross-Sectional Study,” Vaccines, vol. 10, no. 7, pp. 1-14, 2022.
[CrossRef] [Google Scholar] [Publisher Link]
[18] Lucas Walz et al., “Knowledge, Attitudes and Practices Concerning Breast Cancer, Cervical Cancer and Screening among Healthcare Professionals and Students in Mogadishu, Somalia: A Cross-Sectional Study,” Ecancermedicalscience, vol. 16, pp. 1-14, 2022.
[CrossRef] [Google Scholar] [Publisher Link]
[19] Raed Alharbi et al., “Cultural-Aware Machine Learning Based Analysis of COVID-19 Vaccine Hesitancy,” ICC 2023 - IEEE International Conference on Communications, Rome, Italy, pp. 2864-2869, 2023.
[CrossRef] [Google Scholar] [Publisher Link]
[20] Nikita Agarwal, and Ritam Dutta, “Comparative Predictive Analysis of Mortality Rate after Covid-19 Vaccination Using Various Machine Learning Approaches,” 2022 International Conference on Computer Communication and Informatics (ICCCI), Coimbatore, India, pp. 1-5, 2022.
[CrossRef] [Google Scholar] [Publisher Link]
[21] Dimitris Papadopoulos et al., “Predictive Factors for Neutralizing Antibody Levels Nine Months after Full Vaccination with BNT162b2: Results of a Machine Learning Analysis,” Biomedicines, vol. 10, no. 2, pp. 1-19, 2022.
[CrossRef] [Google Scholar] [Publisher Link]
[22] Miftahul Qorib et al., “Covid-19 Vaccine Hesitancy: Text Mining, Sentiment Analysis and Machine Learning on COVID-19 Vaccination Twitter Dataset,” Expert Systems with Applications, vol. 212, pp. 1-14, 2023.
[CrossRef] [Google Scholar] [Publisher Link]
[23] Stephen Wai Hang Kwok, Sai Kumar Vadde, and Guanjin Wang, “Tweet Topics and Sentiments Relating to COVID-19 Vaccination among Australian Twitter Users: Machine Learning Analysis,” Journal of Medical Internet Research, vol. 23, no. 5, 2021.
[CrossRef] [Google Scholar] [Publisher Link]
[24] Buddavarapu Teja Swaroop, “Evaluating Covid-19 Health Information Using Machine Learning,” International Journal of Engineering Applied Sciences and Technology, vol. 5, no. 5, pp. 302-307, 2020.
[CrossRef] [Google Scholar] [Publisher Link]
[25] Marta Malesza, and Magdalena Bozym, “Factors Influencing COVID-19 Vaccination Uptake in an Elderly Sample in Poland,” medRxiv, 2021.
[CrossRef] [Google Scholar] [Publisher Link]
[26] Richard F. Sear et al., “Quantifying COVID-19 Content in the Online Health Opinion War Using Machine Learning,” IEEE Access, vol. 8, pp. 91886-91893, 2020.
[CrossRef] [Google Scholar] [Publisher Link]
[27] Konstantinos Giannakou, Georgia Fakonti, and Maria Kyprianidou, “Determinants of COVID-19 Vaccine Uptake among Healthcare Professionals and the General Population in Cyprus: A Web-Based Cross-Sectional Survey,” Journal of Evaluation in Clinical Practice, vol. 28, no. 6, pp. 959-969, 2022.
[CrossRef] [Google Scholar] [Publisher Link]
[28] Nazeem Muhajarine et al., “COVID-19 Vaccine Hesitancy and Refusal and Associated Factors in an Adult Population in Saskatchewan, Canada: Evidence from Predictive Modelling,” PLoS One, vol. 16, no. 11, pp. 1-18, 2021.
[CrossRef] [Google Scholar] [Publisher Link]
[29] Auriel A. Willette et al., “Using Machine Learning to Predict COVID-19 Infection and Severity Risk among 4510 Aged Adults: A UK Biobank Cohort Study,” Scientific Reports, vol. 12, pp. 1-11, 2022.
[CrossRef] [Google Scholar] [Publisher Link]
[30] Jishan Ahmed et al., “Explainable Machine Learning Approaches to Assess the COVID-19 Vaccination Uptake: Social, Political, and Economic Aspects,” preprints.org, pp. 1-34, 2022.
[CrossRef] [Google Scholar] [Publisher Link]
[31] Theo Audi Yanto et al., “Psychological Factors Affecting COVID-19 Vaccine Acceptance in Indonesia,” The Egyptian Journal of Neurology, Psychiatry and Neurosurgery, vol. 57, pp. 1-8, 2021.
[CrossRef] [Google Scholar] [Publisher Link]
[32] Landry Signé, Strategies for Effective Health Care for Africa in the Fourth Industrial Revolution: Bridging the Gap between the Promise and Delivery, Africa Growth Initiative at Brookings, pp. 1-46, 2021. [Online]. Available: https://www.brookings.edu/wp-content/uploads/2021/10/Strategies-for-effective-health-care-delivery-in-Africa_FINAL.pdf
[33] Abanoub Riad et al., “Belarusian Healthcare Professionals’ Views on Monkeypox and Vaccine Hesitancy,” Vaccines, vol. 11, no. 8, pp. 1-18, 2023.
[CrossRef] [Google Scholar] [Publisher Link]
[34] Abanoub Riad et al., “Universal Predictors of Dental Students’ Attitudes towards Covid-19 Vaccination: Machine Learning-Based Approach,” Vaccines, vol. 9, no. 10, pp. 1-19, 2021.
[CrossRef] [Google Scholar] [Publisher Link]
[35] Afsheen Afzal et al., “Impact of Local and Demographic Factors on Early COVID-19 Vaccine Hesitancy among Health Care Workers in New York City Public Hospitals,” Vaccines, vol. 10, no. 2, pp. 1-16, 2022.
[CrossRef] [Google Scholar] [Publisher Link]
[36] Ma’mon M. Hatmal et al., “Reported Adverse Effects and Attitudes among Arab Populations Following COVID-19 Vaccination: A Large-Scale Multinational Study Implementing Machine Learning Tools in Predicting Post-Vaccination Adverse Effects Based on Predisposing Factors,” Vaccines, vol. 10, no. 3, pp. 1-36, 2022.
[CrossRef] [Google Scholar] [Publisher Link]