Synergistic Excellence: CNN- LSTM Hybrid Model for Improved CKD Diagnosis
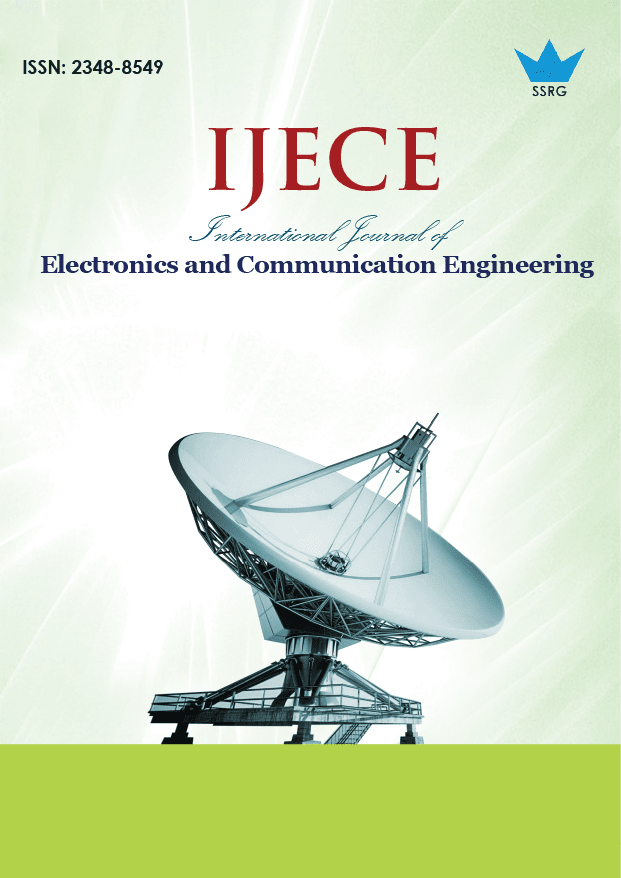
International Journal of Electronics and Communication Engineering |
© 2024 by SSRG - IJECE Journal |
Volume 11 Issue 3 |
Year of Publication : 2024 |
Authors : Jeena Jose, S. Sheeja |
How to Cite?
Jeena Jose, S. Sheeja, "Synergistic Excellence: CNN- LSTM Hybrid Model for Improved CKD Diagnosis," SSRG International Journal of Electronics and Communication Engineering, vol. 11, no. 3, pp. 12-23, 2024. Crossref, https://doi.org/10.14445/23488549/IJECE-V11I3P102
Abstract:
Chronic Kidney Disease (CKD) is a widespread and potentially fatal ailment that impacts millions of individuals globally. Early detection and timely intervention play a crucial role in preventing disease progression and improving patient outcomes. In recent years, advancement in Machine Learning (ML) and data analytics has shown promising potential for aiding the diagnosis and management of diseases. This study investigates the utilization of the CNN LSTM hybrid model to detect CKD using CSV data. To ensure the study’s efficiency, the dataset is collected from the Kaggle repository. The dataset contains 400 samples with 37 different attributes for each sample. The prepared data is utilized for the prediction process, where a CNN is employed. The LSTM network used in this model analyzed the temporal dependencies and patterns in sequential data. The performance of the model was assessed using different performance metrics, resulting in an impressive accuracy rate of 98.75%. The results of this paper carry substantial significance in the progression of Deep Learning (DL) oriented diagnostic instruments for the prompt detection and management of CKD.
Keywords:
Long Short-Term Memory networks, Deep Learning, Kidney function, Machine Learning, Chronic Kidney Disease.
References:
[1] Julian L. Seifter, “Body Fluid Compartments, Cell Membrane Ion Transport, Electrolyte Concentrations, and Acid-Base Balance,” Seminars in Nephrology, vol. 39, no. 4, pp. 368-379, 2019.
[CrossRef] [Google Scholar] [Publisher Link]
[2] Marina Feigenson et al., “Ker-050, A Novel Inhibitor of Tgfβ Superfamily Signalling, Induces Red Blood Cell Production by Promoting Multiple Stages of Erythroid Differentiation,” Blood, vol. 136, no. 1, pp. 1-3, 2020.
[CrossRef] [Google Scholar] [Publisher Link]
[3] Yucheng Tang et al., “Renal Cortex, Medulla and Pelvicaliceal System Segmentation on Arterial Phase CT Images with Random Patch-Based Networks,” Medical Imaging 2021: Image Processing, vol. 11596, 2021.
[CrossRef] [Google Scholar] [Publisher Link]
[4] Jennifer R. Charlton et al., “Nephron Number and Its Determinants: A 2020 Update,” Pediatric Nephrology, vol. 36, pp. 797-807, 2021.
[CrossRef] [Google Scholar] [Publisher Link]
[5] Yaowen Xia et al., “Experimental and Numerical Studies on Indoor Thermal Comfort in Fluid Flow: A Case Study on Primary School Classrooms,” Case Studies in Thermal Engineering, vol. 19, pp. 1-8, 2020.
[CrossRef] [Google Scholar] [Publisher Link]
[6] Jeremy Slivnick, and Brent C. Lampert, “Hypertension and Heart Failure,” Heart Failure Clinics, vol. 15, no. 4, pp. 531-541, 2019.
[CrossRef] [Google Scholar] [Publisher Link]
[7] Stavros A. Kavouras, “Hydration, Dehydration, Underhydration, Optimal Hydration: Are we Barking up the Wrong Tree?,” European Journal of Nutrition, vol. 58, no. 2, pp. 471-473, 2019.
[CrossRef] [Google Scholar] [Publisher Link]
[8] Api Chewcharat, and Gary Curhan, “Trends in the Prevalence of Kidney Stones in the United States from 2007 to 2016,” Urolithiasis, vol. 49, no. 1, pp. 27-39, 2021.
[CrossRef] [Google Scholar] [Publisher Link]
[9] Emilie Cornec-Le Gall, Ahsan Alam, and Ronald D. Perrone, “Autosomal Dominant Polycystic Kidney Disease,” The Lancet, vol. 393, no. 10174, pp. 919-935, 2019.
[CrossRef] [Google Scholar] [Publisher Link]
[10] Mina Khorashadi et al., “Proenkephalin: A New Biomarker for Glomerular Filtration Rate and Acute Kidney Injury,” Nephron, vol. 144, no. 12, pp. 655-661, 2020.
[CrossRef] [Google Scholar] [Publisher Link]
[11] Karen M. Krueger, Michael G. Ison, and Cybele Ghossein, “Practical Guide to Vaccination in All Stages of CKD, Including Patients Treated by Dialysis or Kidney Transplantation,” American Journal of Kidney Diseases, vol. 75, no. 3, pp. 417-425, 2020.
[CrossRef] [Google Scholar] [Publisher Link]
[12] Valerie A. Luyckx, David Z.I. Cherney, and Aminu K. Bello, “Preventing CKD in Developed Countries,” Kidney International Reports, vol. 5, no. 3, pp. 263-277, 2020.
[CrossRef] [Google Scholar] [Publisher Link]
[13] Alice Sabatino et al., “Validation by CT Scan of Quadriceps Muscle Thickness Measurement by Ultrasound in Acute Kidney Injury,” Journal of Nephrology, vol. 33, pp. 109-117, 2020.
[CrossRef] [Google Scholar] [Publisher Link]
[14] Israa Alnazer et al., “Recent Advances in Medical Image Processing for the Evaluation of Chronic Kidney Disease,” Medical Image Analysis, vol. 69, 2021.
[CrossRef] [Google Scholar] [Publisher Link]
[15] Lyudmila A. Bratchenko et al., “Raman Spectroscopy of Human Skin for Kidney Failure Detection,” Journal of Biophotonics, vol. 14, no. 2, 2021.
[CrossRef] [Google Scholar] [Publisher Link]
[16] Sundaram Hariharan, Ajay K. Israni, and Gabriel Danovitch, “Long-Term Survival after Kidney Transplantation,” New England Journal of Medicine, vol. 385, no. 8, pp. 729-743, 2021.
[CrossRef] [Google Scholar] [Publisher Link]
[17] Rebecca Noble, and Maarten W. Taal, “Epidemiology and Causes of Chronic Kidney Disease,” Medicine, vol. 47, no. 9, pp. 562-566, 2019.
[CrossRef] [Google Scholar] [Publisher Link]
[18] Thomas Lees et al., “Hydrological Concept Formation Inside Long Short-Term Memory (LSTM) Networks,” Hydrology and Earth System Sciences, vol. 26, no. 12, pp. 3079-3101, 2022.
[CrossRef] [Google Scholar] [Publisher Link]
[19] Fuzhe Ma et al., “Detection and Diagnosis of Chronic Kidney Disease Using Deep Learning-Based Heterogeneous Modified Artificial Neural Network,” Future Generation Computer Systems, vol. 111, pp. 17-26, 2020.
[CrossRef] [Google Scholar] [Publisher Link]
[20] Luana Batista da Cruz et al., “Kidney Segmentation from Computed Tomography Images Using Deep Neural Network,” Computers in Biology and Medicine, vol. 123, 2020.
[CrossRef] [Google Scholar] [Publisher Link]
[21] Himanshu Kriplani, Bhumi Patel, and Sudipta Roy, “Prediction of Chronic Kidney Diseases Using Deep Artificial Neural Network Technique,” Computer-Aided Intervention and Diagnostics in Clinical and Medical Images, pp. 179-187, 2019.
[CrossRef] [Google Scholar] [Publisher Link]
[22] Abdullah Al Imran, Md Nur Amin, and Fatema Tuj Johora, “Classification of Chronic Kidney Disease Using Logistic Regression, Feedforward Neural Network and Wide & Deep Learning,” 2018 International Conference on Innovation in Engineering and Technology (ICIET), Dhaka, Bangladesh, pp. 1-6, 2018.
[CrossRef] [Google Scholar] [Publisher Link]
[23] Shamima Akter et al., “Comprehensive Performance Assessment of Deep Learning Models in Early Prediction and Risk Identification of Chronic Kidney Disease,” IEEE Access, vol. 9, pp. 165184-165206, 2021.
[CrossRef] [Google Scholar] [Publisher Link]
[24] Yedilkhan Amirgaliyev, Shahriar Shamiluulu, and Azamat Serek, “Analysis of Chronic Kidney Disease Dataset by Applying Machine Learning Methods,” 2018 IEEE 12th International Conference on Application of Information and Communication Technologies (AICT), Almaty, Kazakhstan, pp. 1-4, 2018.
[CrossRef] [Google Scholar] [Publisher Link]
[25] Jiongming Qin et al., “A Machine Learning Methodology for Diagnosing Chronic Kidney Disease,” IEEE Access, vol. 8, pp. 20991- 21002, 2019.
[CrossRef] [Google Scholar] [Publisher Link]
[26] Md. Rashed-Al-Mahfuz et al., “Clinically Applicable Machine Learning Approaches to Identify Attributes of Chronic Kidney Disease (CKD) for Use in Low-Cost Diagnostic Screening,” IEEE Journal of Translational Engineering in Health and Medicine, vol. 9, pp. 1-11, 2021.
[CrossRef] [Google Scholar] [Publisher Link]
[27] Bilal Khan et al., “An Empirical Evaluation of Machine Learning Techniques for Chronic Kidney Disease Prophecy,” IEEE Access, vol. 8, pp. 55012-55022, 2021.
[CrossRef] [Google Scholar] [Publisher Link]
[28] Pankaj Chittora et al., “Prediction of Chronic Kidney Disease-A Machine Learning Perspective,” IEEE Access, vol. 9, pp. 17312-17334, 2021.
[CrossRef] [Google Scholar] [Publisher Link]
[29] Divya Jain, and Vijendra Singh, “A Two-Phase Hybrid Approach Using Feature Selection and Adaptive SVM for Chronic Disease Classification,” International Journal of Computers and Applications, vol. 43, no. 6, pp. 524-536, 2021.
[CrossRef] [Google Scholar] [Publisher Link]
[30] Manal A. Abdel-Fattah, Nermin Abdelhakim Othman, and Nagwa Goher, “Predicting Chronic Kidney Disease Using Hybrid Machine Learning Based on Apache Spark,” Computational Intelligence and Neuroscience, vol. 2022, pp. 1-12, 2022.
[CrossRef] [Google Scholar] [Publisher Link]
[31] Dhyan Chandra Yadav, and Saurabh Pal, “Performance-Based Evaluation of Algorithms on Chronic Kidney Disease Using Hybrid Ensemble Model in Machine Learning,” Biomedical and Pharmacology Journal, vol. 14, no. 3, pp. 1633-1645, 2021.
[CrossRef] [Google Scholar] [Publisher Link]
[32] Shubham Vashisth, Ishika Dhall, and Shipra Saraswat, “Chronic Kidney Disease (CKD) Diagnosis Using Multi-Layer Perceptron Classifier,” 2020 10th International Conference on Cloud Computing, Data Science & Engineering (Confluence), Noida, India, pp. 346- 350, 2020.
[CrossRef] [Google Scholar] [Publisher Link]
[33] B.V. Ravindra, N. Sriraam, and M. Geetha, “Chronic Kidney Disease Detection Using Back Propagation Neural Network Classifier,” 2018 International Conference on Communication, Computing and Internet of Things (IC3IoT), Chennai, India, pp. 65-68, 2018.
[CrossRef] [Google Scholar] [Publisher Link]
[34] Chalumuru Suresh et al., “A Neural Network-Based Model for Predicting Chronic Kidney Diseases,” 2020 Second International Conference on Inventive Research in Computing Applications (ICIRCA), Coimbatore, India, pp. 157-162, 2020.
[CrossRef] [Google Scholar] [Publisher Link]
[35] Navaneeth Bhaskar, and M. Suchetha, “An Approach for Analysis and Prediction of CKD Using Deep Learning Architecture,” 2019 International Conference on Communication and Electronics Systems (ICCES), Coimbatore, India, pp. 1660-1664, 2019.
[CrossRef] [Google Scholar] [Publisher Link]